کد مقاله | کد نشریه | سال انتشار | مقاله انگلیسی | نسخه تمام متن |
---|---|---|---|---|
637398 | 883685 | 2009 | 12 صفحه PDF | دانلود رایگان |
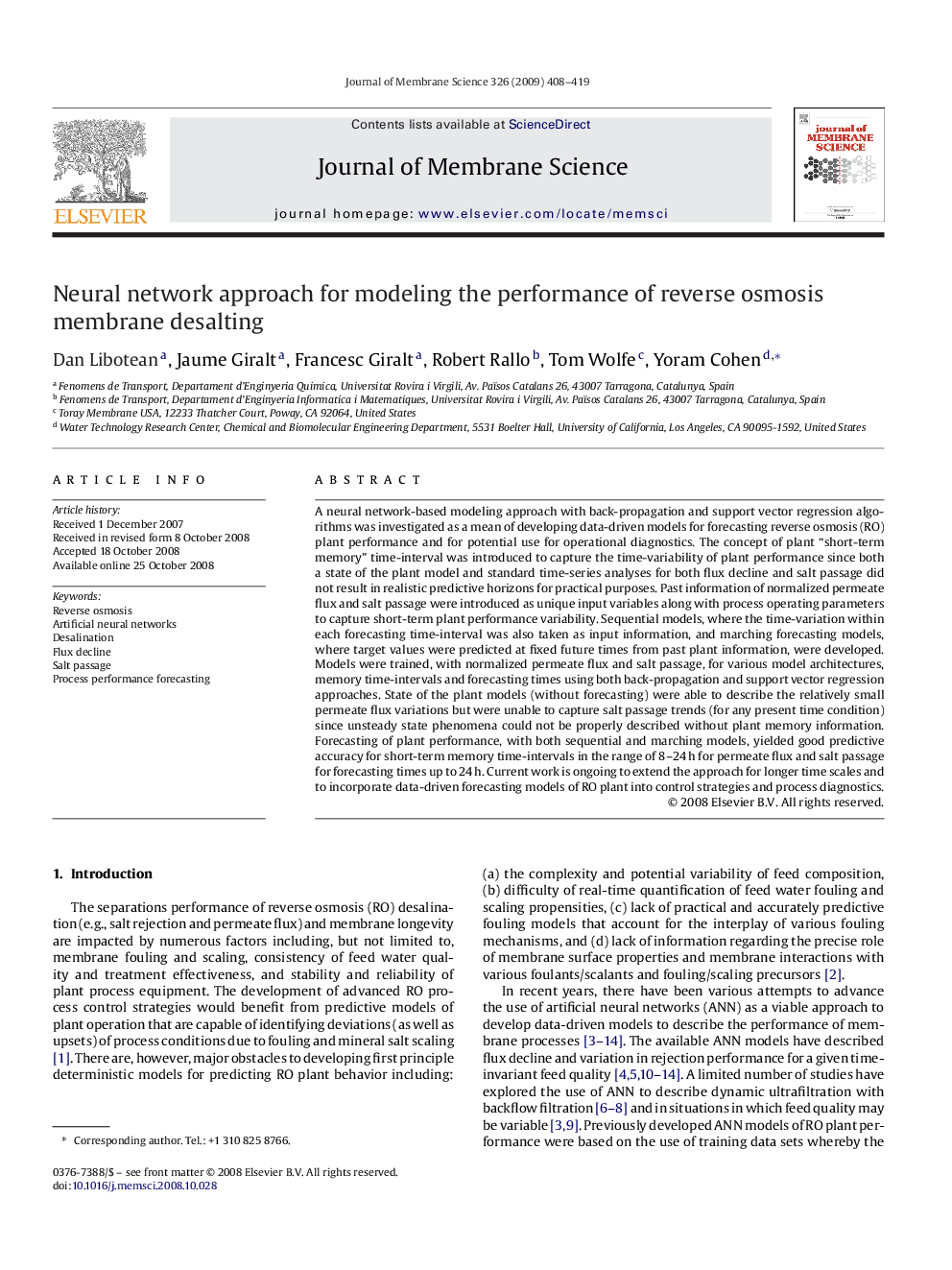
A neural network-based modeling approach with back-propagation and support vector regression algorithms was investigated as a mean of developing data-driven models for forecasting reverse osmosis (RO) plant performance and for potential use for operational diagnostics. The concept of plant “short-term memory” time-interval was introduced to capture the time-variability of plant performance since both a state of the plant model and standard time-series analyses for both flux decline and salt passage did not result in realistic predictive horizons for practical purposes. Past information of normalized permeate flux and salt passage were introduced as unique input variables along with process operating parameters to capture short-term plant performance variability. Sequential models, where the time-variation within each forecasting time-interval was also taken as input information, and marching forecasting models, where target values were predicted at fixed future times from past plant information, were developed. Models were trained, with normalized permeate flux and salt passage, for various model architectures, memory time-intervals and forecasting times using both back-propagation and support vector regression approaches. State of the plant models (without forecasting) were able to describe the relatively small permeate flux variations but were unable to capture salt passage trends (for any present time condition) since unsteady state phenomena could not be properly described without plant memory information. Forecasting of plant performance, with both sequential and marching models, yielded good predictive accuracy for short-term memory time-intervals in the range of 8–24 h for permeate flux and salt passage for forecasting times up to 24 h. Current work is ongoing to extend the approach for longer time scales and to incorporate data-driven forecasting models of RO plant into control strategies and process diagnostics.
Journal: Journal of Membrane Science - Volume 326, Issue 2, 20 January 2009, Pages 408–419