کد مقاله | کد نشریه | سال انتشار | مقاله انگلیسی | نسخه تمام متن |
---|---|---|---|---|
6484216 | 1416076 | 2017 | 12 صفحه PDF | دانلود رایگان |
عنوان انگلیسی مقاله ISI
A multi-layered incremental feature selection algorithm for adjuvant chemotherapy effectiveness/futileness assessment in non-small cell lung cancer
دانلود مقاله + سفارش ترجمه
دانلود مقاله ISI انگلیسی
رایگان برای ایرانیان
کلمات کلیدی
موضوعات مرتبط
مهندسی و علوم پایه
مهندسی شیمی
بیو مهندسی (مهندسی زیستی)
پیش نمایش صفحه اول مقاله
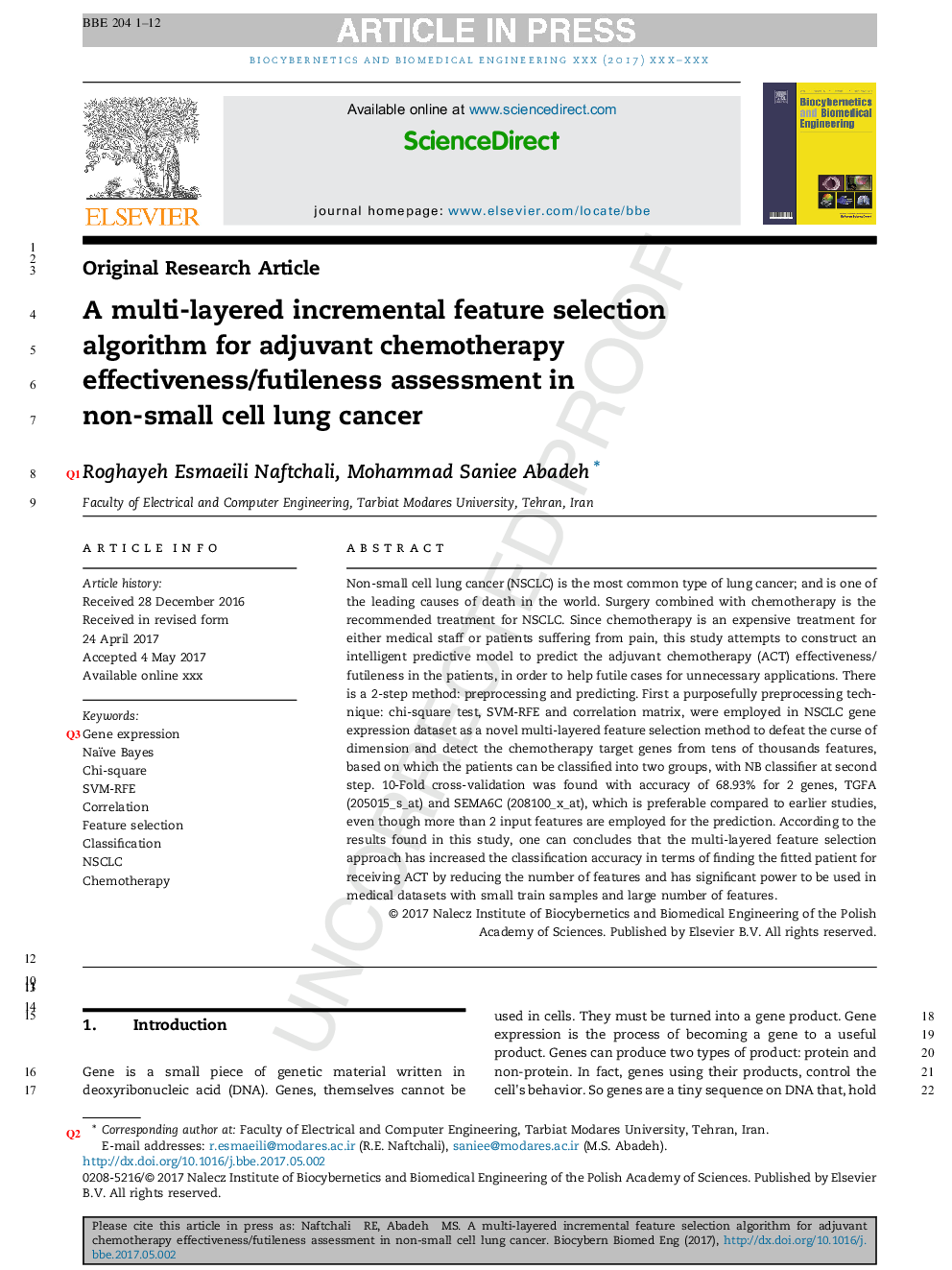
چکیده انگلیسی
Non-small cell lung cancer (NSCLC) is the most common type of lung cancer; and is one of the leading causes of death in the world. Surgery combined with chemotherapy is the recommended treatment for NSCLC. Since chemotherapy is an expensive treatment for either medical staff or patients suffering from pain, this study attempts to construct an intelligent predictive model to predict the adjuvant chemotherapy (ACT) effectiveness/futileness in the patients, in order to help futile cases for unnecessary applications. There is a 2-step method: preprocessing and predicting. First a purposefully preprocessing technique: chi-square test, SVM-RFE and correlation matrix, were employed in NSCLC gene expression dataset as a novel multi-layered feature selection method to defeat the curse of dimension and detect the chemotherapy target genes from tens of thousands features, based on which the patients can be classified into two groups, with NB classifier at second step. 10-Fold cross-validation was found with accuracy of 68.93% for 2 genes, TGFA (205015_s_at) and SEMA6C (208100_x_at), which is preferable compared to earlier studies, even though more than 2 input features are employed for the prediction. According to the results found in this study, one can concludes that the multi-layered feature selection approach has increased the classification accuracy in terms of finding the fitted patient for receiving ACT by reducing the number of features and has significant power to be used in medical datasets with small train samples and large number of features.
ناشر
Database: Elsevier - ScienceDirect (ساینس دایرکت)
Journal: Biocybernetics and Biomedical Engineering - Volume 37, Issue 3, 2017, Pages 477-488
Journal: Biocybernetics and Biomedical Engineering - Volume 37, Issue 3, 2017, Pages 477-488
نویسندگان
Roghayeh Esmaeili Naftchali, Mohammad Saniee Abadeh,