کد مقاله | کد نشریه | سال انتشار | مقاله انگلیسی | نسخه تمام متن |
---|---|---|---|---|
6854551 | 1437452 | 2014 | 17 صفحه PDF | دانلود رایگان |
عنوان انگلیسی مقاله ISI
Supervised feature selection for linear and non-linear regression of Lâaâbâ color from multispectral images of meat
دانلود مقاله + سفارش ترجمه
دانلود مقاله ISI انگلیسی
رایگان برای ایرانیان
کلمات کلیدی
موضوعات مرتبط
مهندسی و علوم پایه
مهندسی کامپیوتر
هوش مصنوعی
پیش نمایش صفحه اول مقاله
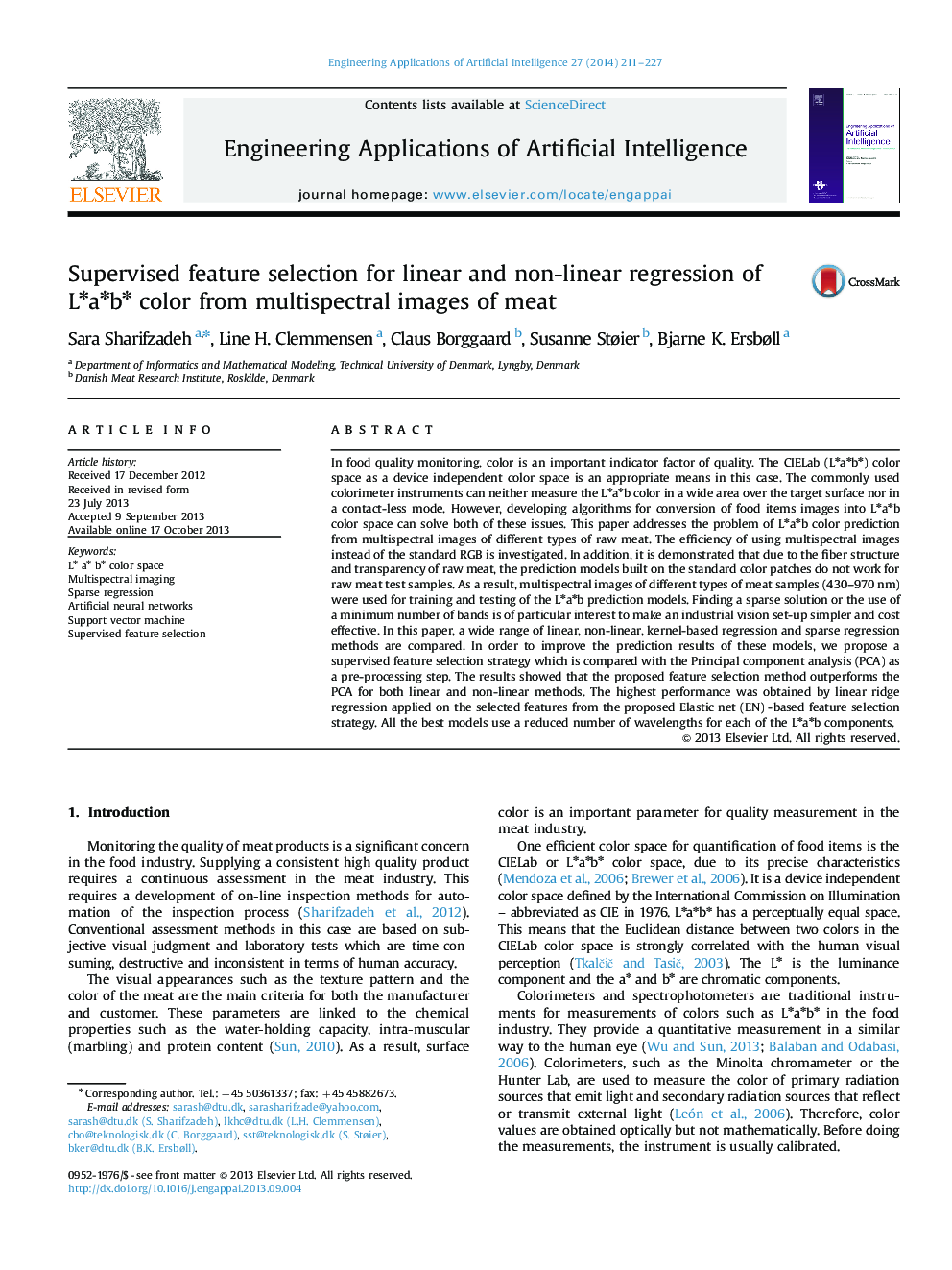
چکیده انگلیسی
In food quality monitoring, color is an important indicator factor of quality. The CIELab (Lâaâbâ) color space as a device independent color space is an appropriate means in this case. The commonly used colorimeter instruments can neither measure the Lâaâb color in a wide area over the target surface nor in a contact-less mode. However, developing algorithms for conversion of food items images into Lâaâb color space can solve both of these issues. This paper addresses the problem of Lâaâb color prediction from multispectral images of different types of raw meat. The efficiency of using multispectral images instead of the standard RGB is investigated. In addition, it is demonstrated that due to the fiber structure and transparency of raw meat, the prediction models built on the standard color patches do not work for raw meat test samples. As a result, multispectral images of different types of meat samples (430-970Â nm) were used for training and testing of the Lâaâb prediction models. Finding a sparse solution or the use of a minimum number of bands is of particular interest to make an industrial vision set-up simpler and cost effective. In this paper, a wide range of linear, non-linear, kernel-based regression and sparse regression methods are compared. In order to improve the prediction results of these models, we propose a supervised feature selection strategy which is compared with the Principal component analysis (PCA) as a pre-processing step. The results showed that the proposed feature selection method outperforms the PCA for both linear and non-linear methods. The highest performance was obtained by linear ridge regression applied on the selected features from the proposed Elastic net (EN) -based feature selection strategy. All the best models use a reduced number of wavelengths for each of the Lâaâb components.
ناشر
Database: Elsevier - ScienceDirect (ساینس دایرکت)
Journal: Engineering Applications of Artificial Intelligence - Volume 27, January 2014, Pages 211-227
Journal: Engineering Applications of Artificial Intelligence - Volume 27, January 2014, Pages 211-227
نویسندگان
Sara Sharifzadeh, Line H. Clemmensen, Claus Borggaard, Susanne Støier, Bjarne K. Ersbøll,