کد مقاله | کد نشریه | سال انتشار | مقاله انگلیسی | نسخه تمام متن |
---|---|---|---|---|
6855899 | 1437695 | 2018 | 18 صفحه PDF | دانلود رایگان |
عنوان انگلیسی مقاله ISI
On the unification of possibilistic fuzzy clustering: Axiomatic development and convergence analysis
دانلود مقاله + سفارش ترجمه
دانلود مقاله ISI انگلیسی
رایگان برای ایرانیان
کلمات کلیدی
موضوعات مرتبط
مهندسی و علوم پایه
مهندسی کامپیوتر
هوش مصنوعی
پیش نمایش صفحه اول مقاله
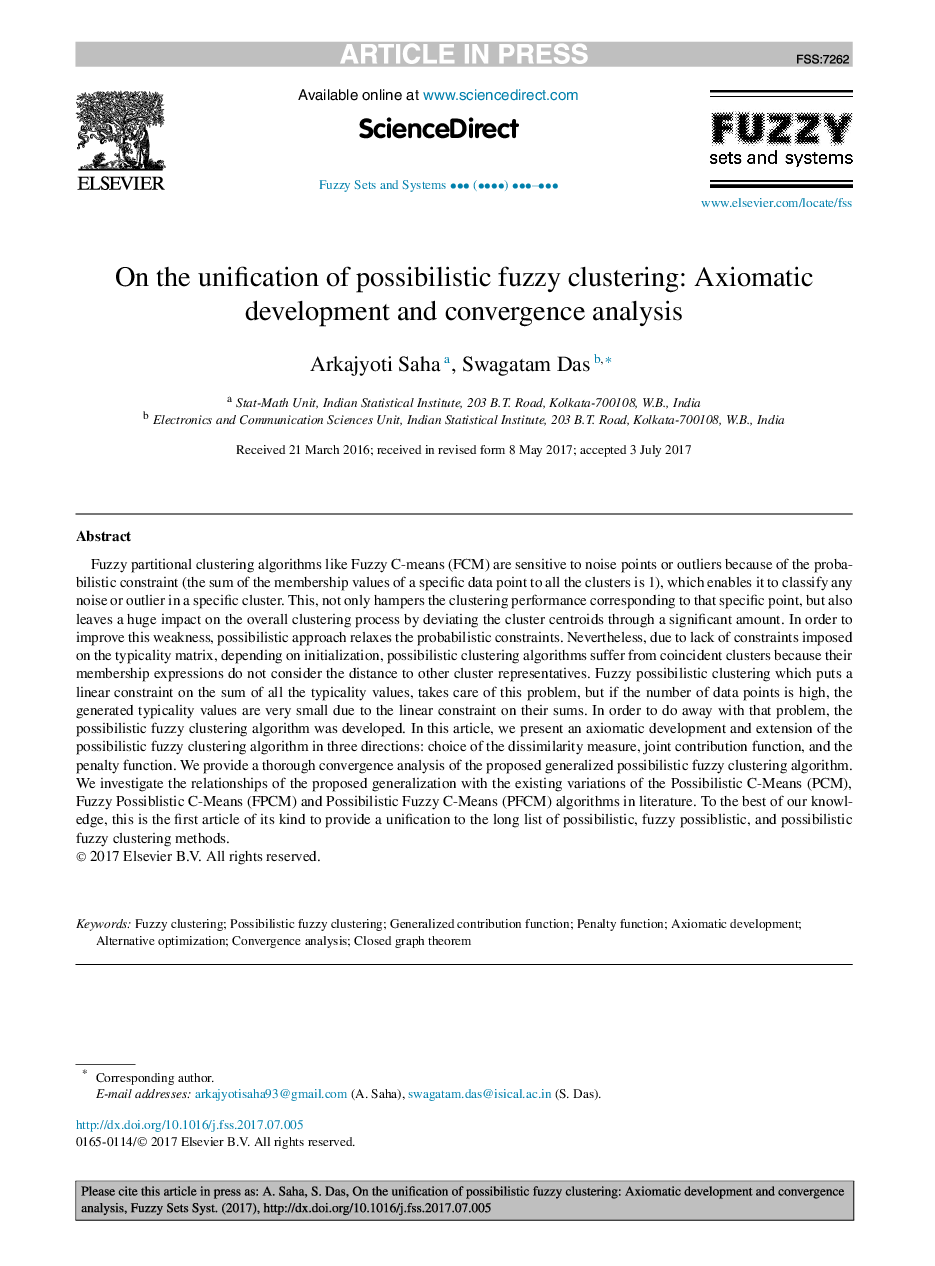
چکیده انگلیسی
Fuzzy partitional clustering algorithms like Fuzzy C-means (FCM) are sensitive to noise points or outliers because of the probabilistic constraint (the sum of the membership values of a specific data point to all the clusters is 1), which enables it to classify any noise or outlier in a specific cluster. This, not only hampers the clustering performance corresponding to that specific point, but also leaves a huge impact on the overall clustering process by deviating the cluster centroids through a significant amount. In order to improve this weakness, possibilistic approach relaxes the probabilistic constraints. Nevertheless, due to lack of constraints imposed on the typicality matrix, depending on initialization, possibilistic clustering algorithms suffer from coincident clusters because their membership expressions do not consider the distance to other cluster representatives. Fuzzy possibilistic clustering which puts a linear constraint on the sum of all the typicality values, takes care of this problem, but if the number of data points is high, the generated typicality values are very small due to the linear constraint on their sums. In order to do away with that problem, the possibilistic fuzzy clustering algorithm was developed. In this article, we present an axiomatic development and extension of the possibilistic fuzzy clustering algorithm in three directions: choice of the dissimilarity measure, joint contribution function, and the penalty function. We provide a thorough convergence analysis of the proposed generalized possibilistic fuzzy clustering algorithm. We investigate the relationships of the proposed generalization with the existing variations of the Possibilistic C-Means (PCM), Fuzzy Possiblistic C-Means (FPCM) and Possibilistic Fuzzy C-Means (PFCM) algorithms in literature. To the best of our knowledge, this is the first article of its kind to provide a unification to the long list of possibilistic, fuzzy possiblistic, and possibilistic fuzzy clustering methods.
ناشر
Database: Elsevier - ScienceDirect (ساینس دایرکت)
Journal: Fuzzy Sets and Systems - Volume 340, 1 June 2018, Pages 73-90
Journal: Fuzzy Sets and Systems - Volume 340, 1 June 2018, Pages 73-90
نویسندگان
Arkajyoti Saha, Swagatam Das,