کد مقاله | کد نشریه | سال انتشار | مقاله انگلیسی | نسخه تمام متن |
---|---|---|---|---|
6856207 | 1437949 | 2018 | 19 صفحه PDF | دانلود رایگان |
عنوان انگلیسی مقاله ISI
Predicting attributes and friends of mobile users from AP-Trajectories
دانلود مقاله + سفارش ترجمه
دانلود مقاله ISI انگلیسی
رایگان برای ایرانیان
موضوعات مرتبط
مهندسی و علوم پایه
مهندسی کامپیوتر
هوش مصنوعی
پیش نمایش صفحه اول مقاله
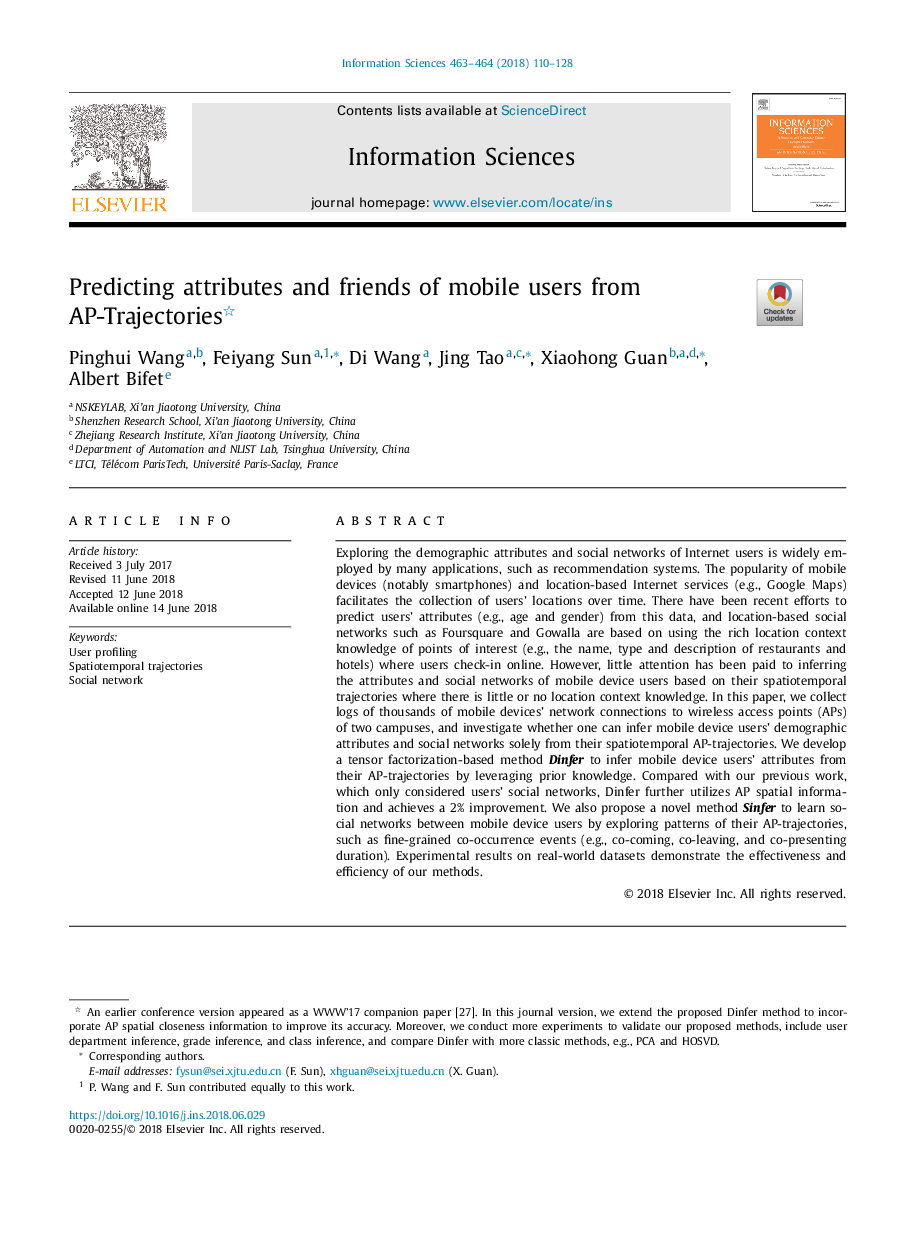
چکیده انگلیسی
Exploring the demographic attributes and social networks of Internet users is widely employed by many applications, such as recommendation systems. The popularity of mobile devices (notably smartphones) and location-based Internet services (e.g., Google Maps) facilitates the collection of users' locations over time. There have been recent efforts to predict users' attributes (e.g., age and gender) from this data, and location-based social networks such as Foursquare and Gowalla are based on using the rich location context knowledge of points of interest (e.g., the name, type and description of restaurants and hotels) where users check-in online. However, little attention has been paid to inferring the attributes and social networks of mobile device users based on their spatiotemporal trajectories where there is little or no location context knowledge. In this paper, we collect logs of thousands of mobile devices' network connections to wireless access points (APs) of two campuses, and investigate whether one can infer mobile device users' demographic attributes and social networks solely from their spatiotemporal AP-trajectories. We develop a tensor factorization-based method Dinfer to infer mobile device users' attributes from their AP-trajectories by leveraging prior knowledge. Compared with our previous work, which only considered users' social networks, Dinfer further utilizes AP spatial information and achieves a 2% improvement. We also propose a novel method Sinfer to learn social networks between mobile device users by exploring patterns of their AP-trajectories, such as fine-grained co-occurrence events (e.g., co-coming, co-leaving, and co-presenting duration). Experimental results on real-world datasets demonstrate the effectiveness and efficiency of our methods.
ناشر
Database: Elsevier - ScienceDirect (ساینس دایرکت)
Journal: Information Sciences - Volumes 463â464, October 2018, Pages 110-128
Journal: Information Sciences - Volumes 463â464, October 2018, Pages 110-128
نویسندگان
Pinghui Wang, Feiyang Sun, Di Wang, Jing Tao, Xiaohong Guan, Albert Bifet,