کد مقاله | کد نشریه | سال انتشار | مقاله انگلیسی | نسخه تمام متن |
---|---|---|---|---|
6856212 | 1437949 | 2018 | 20 صفحه PDF | دانلود رایگان |
عنوان انگلیسی مقاله ISI
A new distance with derivative information for functional k-means clustering algorithm
دانلود مقاله + سفارش ترجمه
دانلود مقاله ISI انگلیسی
رایگان برای ایرانیان
موضوعات مرتبط
مهندسی و علوم پایه
مهندسی کامپیوتر
هوش مصنوعی
پیش نمایش صفحه اول مقاله
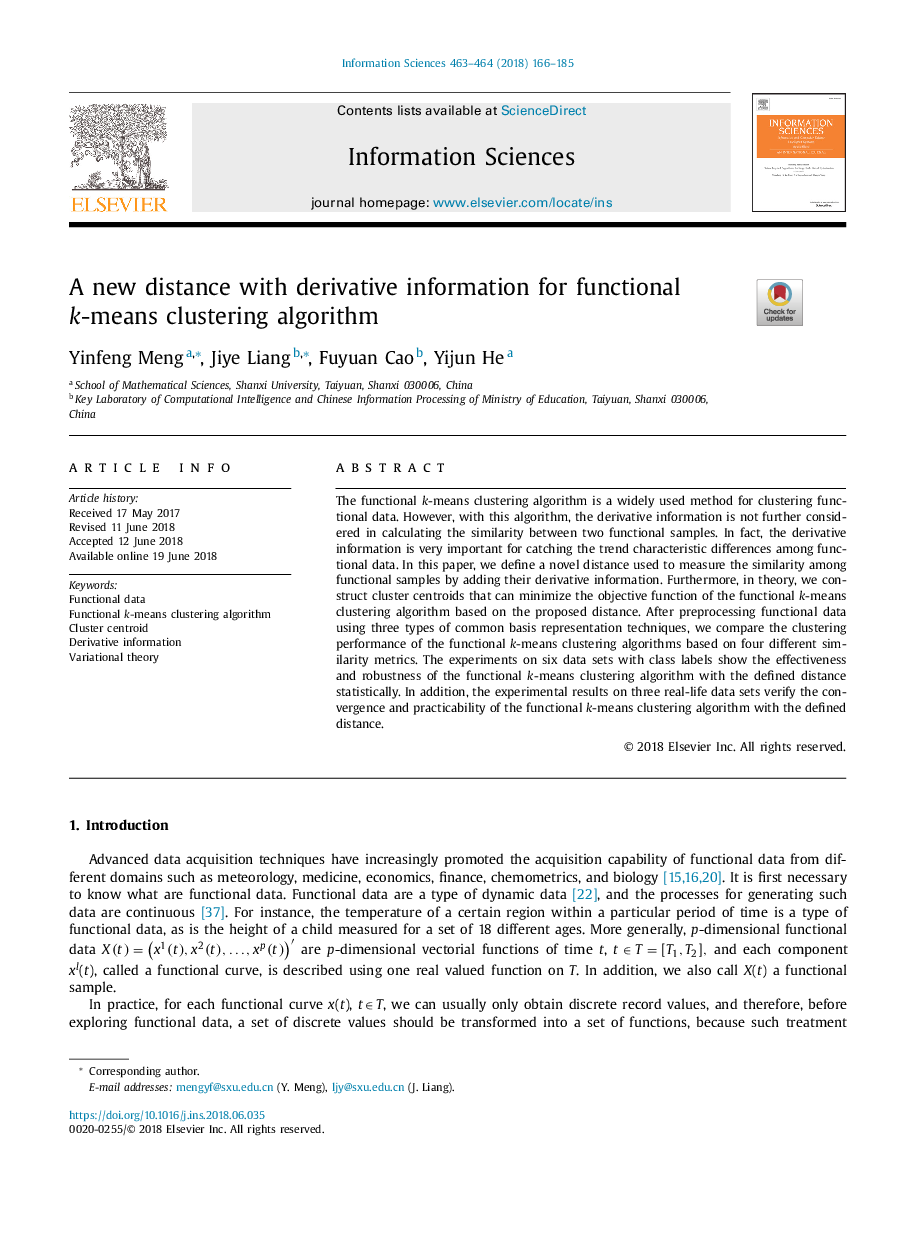
چکیده انگلیسی
The functional k-means clustering algorithm is a widely used method for clustering functional data. However, with this algorithm, the derivative information is not further considered in calculating the similarity between two functional samples. In fact, the derivative information is very important for catching the trend characteristic differences among functional data. In this paper, we define a novel distance used to measure the similarity among functional samples by adding their derivative information. Furthermore, in theory, we construct cluster centroids that can minimize the objective function of the functional k-means clustering algorithm based on the proposed distance. After preprocessing functional data using three types of common basis representation techniques, we compare the clustering performance of the functional k-means clustering algorithms based on four different similarity metrics. The experiments on six data sets with class labels show the effectiveness and robustness of the functional k-means clustering algorithm with the defined distance statistically. In addition, the experimental results on three real-life data sets verify the convergence and practicability of the functional k-means clustering algorithm with the defined distance.
ناشر
Database: Elsevier - ScienceDirect (ساینس دایرکت)
Journal: Information Sciences - Volumes 463â464, October 2018, Pages 166-185
Journal: Information Sciences - Volumes 463â464, October 2018, Pages 166-185
نویسندگان
Yinfeng Meng, Jiye Liang, Fuyuan Cao, Yijun He,