کد مقاله | کد نشریه | سال انتشار | مقاله انگلیسی | نسخه تمام متن |
---|---|---|---|---|
6856442 | 1437957 | 2018 | 26 صفحه PDF | دانلود رایگان |
عنوان انگلیسی مقاله ISI
Group sparsity with orthogonal dictionary and nonconvex regularization for exact MRI reconstruction
دانلود مقاله + سفارش ترجمه
دانلود مقاله ISI انگلیسی
رایگان برای ایرانیان
کلمات کلیدی
موضوعات مرتبط
مهندسی و علوم پایه
مهندسی کامپیوتر
هوش مصنوعی
پیش نمایش صفحه اول مقاله
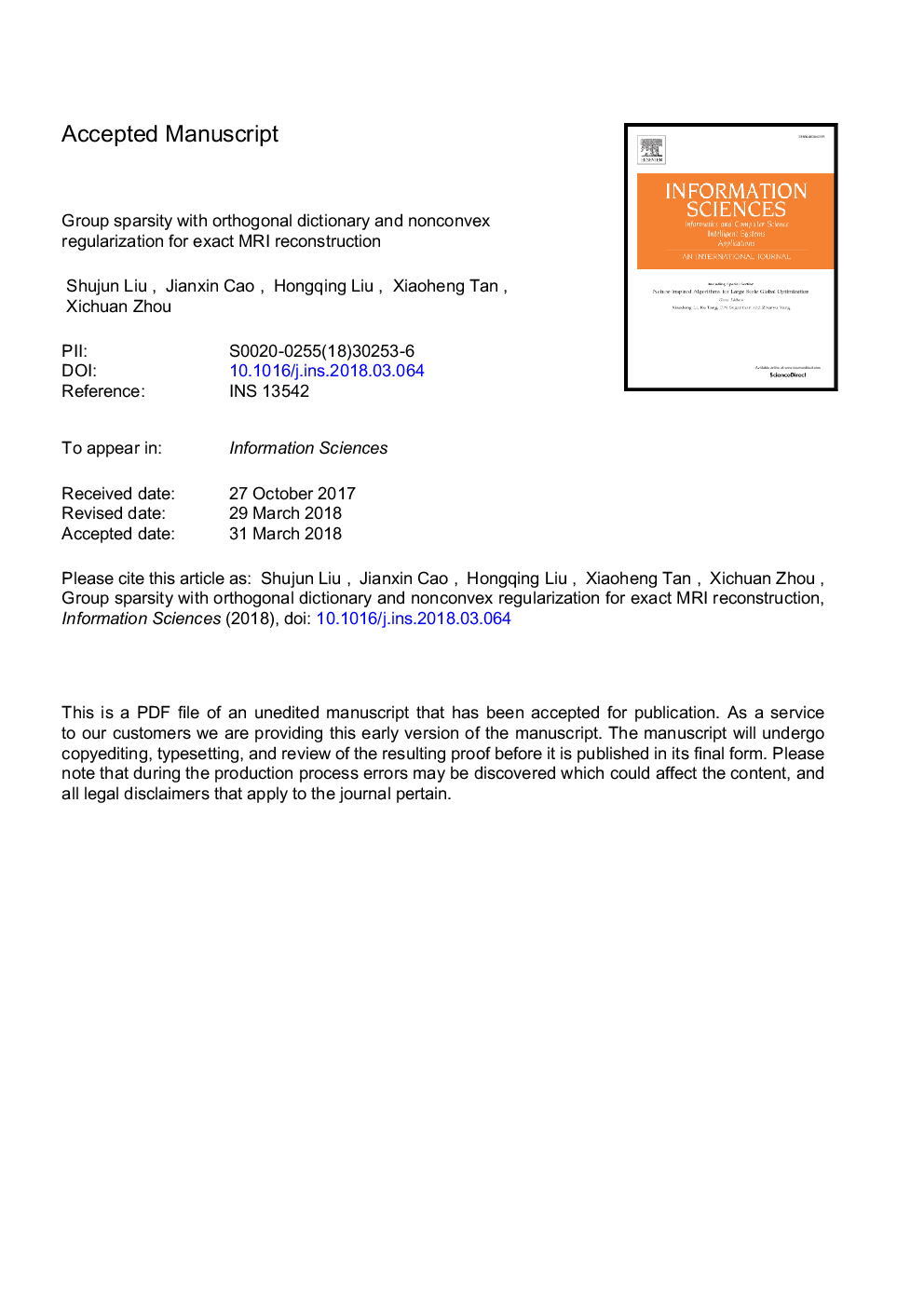
چکیده انگلیسی
Compressed sensing MRI (CS-MRI) significantly accelerates scanning time via accurate reconstruction of the image from undersampled k-space data. In this work, combining two priors of sparsity and nonlocal similarity, an algorithm of group sparsity with an orthogonal dictionary (GSOD) is proposed to realize CS-MRI reconstruction within an optimization framework. To efficiently solve the resultant non-convex optimization, a lower bound of the original problem is derived, and generalized soft-thresholding is then applied to obtain the solution from that lower bound in a fast and accurate manner. Moreover, considering the important role of the dictionary in sparse representation, a modified GSOD (M-GSOD) approach is also developed in which the orthogonal dictionary is adaptively learned from the group. It is proven that the proposed sparse coding model in the M-GSOD is equivalent to the low-rank model, and the connection between the two independent models is established for the first time. Finally, a fast and accurate algorithm to solve M-GSOD is provided. Compared with the current methods, the proposed methods demonstrate a state-of-the-art performance, which shows the correctness of the non-convex regularization and optimal dictionary learning.
ناشر
Database: Elsevier - ScienceDirect (ساینس دایرکت)
Journal: Information Sciences - Volumes 451â452, July 2018, Pages 161-179
Journal: Information Sciences - Volumes 451â452, July 2018, Pages 161-179
نویسندگان
Liu Shujun, Cao Jianxin, Liu Hongqing, Tan Xiaoheng, Zhou Xichuan,