کد مقاله | کد نشریه | سال انتشار | مقاله انگلیسی | نسخه تمام متن |
---|---|---|---|---|
6864808 | 1439552 | 2018 | 12 صفحه PDF | دانلود رایگان |
عنوان انگلیسی مقاله ISI
An improved class of real-coded Genetic Algorithms for numerical optimizationâ°
دانلود مقاله + سفارش ترجمه
دانلود مقاله ISI انگلیسی
رایگان برای ایرانیان
کلمات کلیدی
موضوعات مرتبط
مهندسی و علوم پایه
مهندسی کامپیوتر
هوش مصنوعی
پیش نمایش صفحه اول مقاله
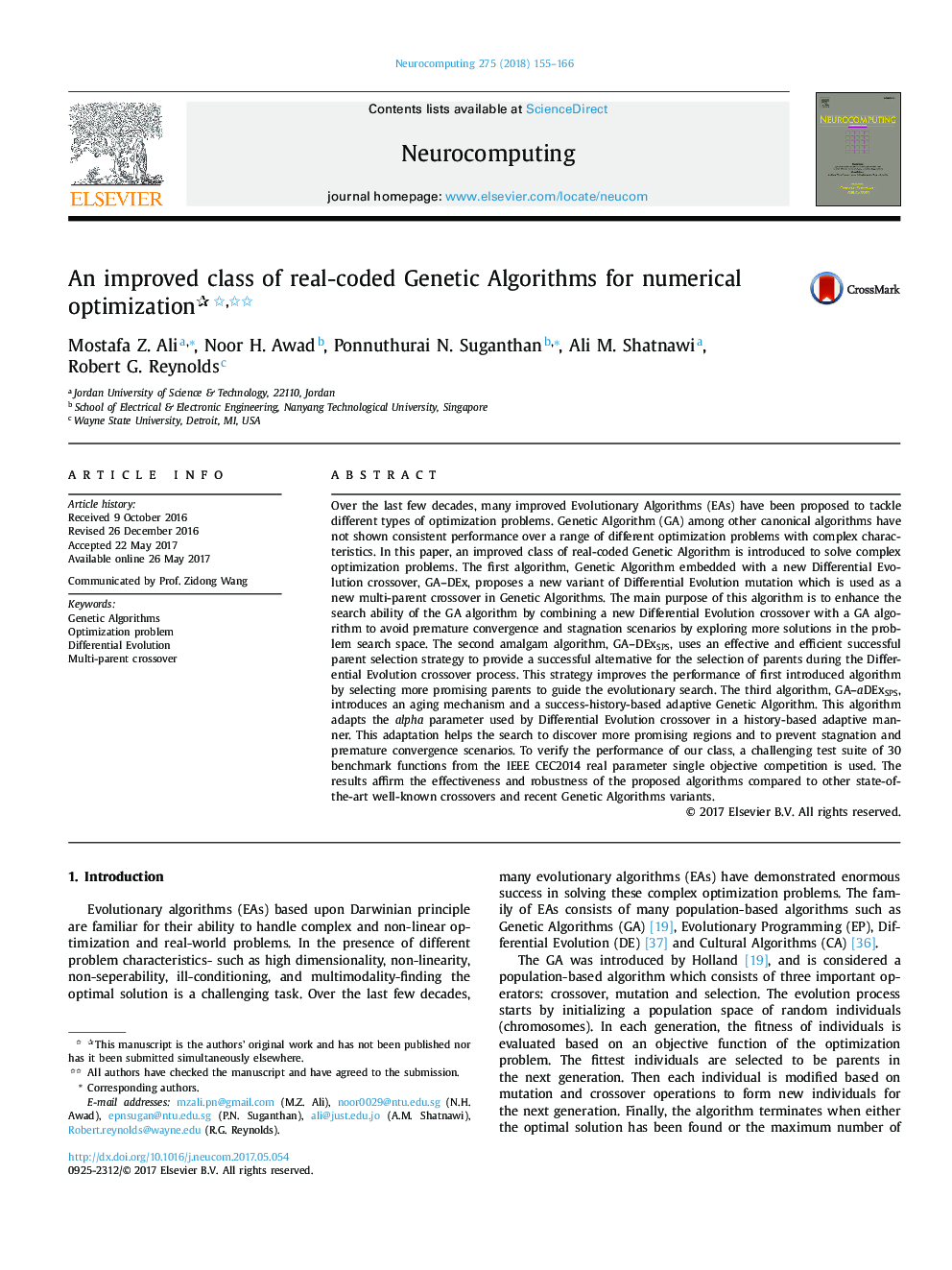
چکیده انگلیسی
Over the last few decades, many improved Evolutionary Algorithms (EAs) have been proposed to tackle different types of optimization problems. Genetic Algorithm (GA) among other canonical algorithms have not shown consistent performance over a range of different optimization problems with complex characteristics. In this paper, an improved class of real-coded Genetic Algorithm is introduced to solve complex optimization problems. The first algorithm, Genetic Algorithm embedded with a new Differential Evolution crossover, GA-DEx, proposes a new variant of Differential Evolution mutation which is used as a new multi-parent crossover in Genetic Algorithms. The main purpose of this algorithm is to enhance the search ability of the GA algorithm by combining a new Differential Evolution crossover with a GA algorithm to avoid premature convergence and stagnation scenarios by exploring more solutions in the problem search space. The second amalgam algorithm, GA-DExSPS, uses an effective and efficient successful parent selection strategy to provide a successful alternative for the selection of parents during the Differential Evolution crossover process. This strategy improves the performance of first introduced algorithm by selecting more promising parents to guide the evolutionary search. The third algorithm, GA-aDExSPS, introduces an aging mechanism and a success-history-based adaptive Genetic Algorithm. This algorithm adapts the alpha parameter used by Differential Evolution crossover in a history-based adaptive manner. This adaptation helps the search to discover more promising regions and to prevent stagnation and premature convergence scenarios. To verify the performance of our class, a challenging test suite of 30 benchmark functions from the IEEE CEC2014 real parameter single objective competition is used. The results affirm the effectiveness and robustness of the proposed algorithms compared to other state-of-the-art well-known crossovers and recent Genetic Algorithms variants.
ناشر
Database: Elsevier - ScienceDirect (ساینس دایرکت)
Journal: Neurocomputing - Volume 275, 31 January 2018, Pages 155-166
Journal: Neurocomputing - Volume 275, 31 January 2018, Pages 155-166
نویسندگان
Mostafa Z. Ali, Noor H. Awad, Ponnuthurai N. Suganthan, Ali M. Shatnawi, Robert G. Reynolds,