کد مقاله | کد نشریه | سال انتشار | مقاله انگلیسی | نسخه تمام متن |
---|---|---|---|---|
6865490 | 679032 | 2016 | 20 صفحه PDF | دانلود رایگان |
عنوان انگلیسی مقاله ISI
Adaptive memetic algorithm enhanced with data geometry analysis to select training data for SVMs
دانلود مقاله + سفارش ترجمه
دانلود مقاله ISI انگلیسی
رایگان برای ایرانیان
کلمات کلیدی
موضوعات مرتبط
مهندسی و علوم پایه
مهندسی کامپیوتر
هوش مصنوعی
پیش نمایش صفحه اول مقاله
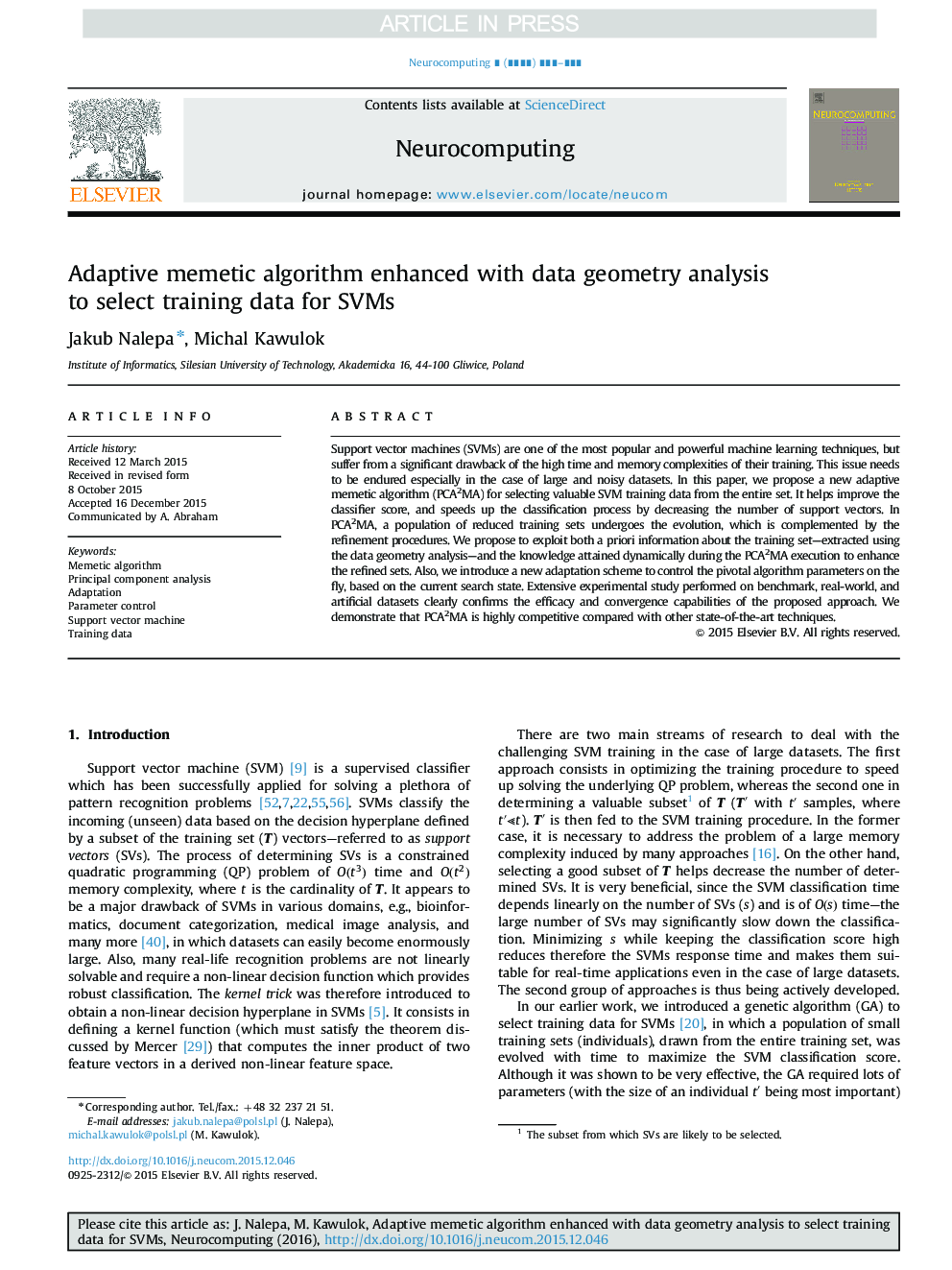
چکیده انگلیسی
Support vector machines (SVMs) are one of the most popular and powerful machine learning techniques, but suffer from a significant drawback of the high time and memory complexities of their training. This issue needs to be endured especially in the case of large and noisy datasets. In this paper, we propose a new adaptive memetic algorithm (PCA2MA) for selecting valuable SVM training data from the entire set. It helps improve the classifier score, and speeds up the classification process by decreasing the number of support vectors. In PCA2MA, a population of reduced training sets undergoes the evolution, which is complemented by the refinement procedures. We propose to exploit both a priori information about the training set-extracted using the data geometry analysis-and the knowledge attained dynamically during the PCA2MA execution to enhance the refined sets. Also, we introduce a new adaptation scheme to control the pivotal algorithm parameters on the fly, based on the current search state. Extensive experimental study performed on benchmark, real-world, and artificial datasets clearly confirms the efficacy and convergence capabilities of the proposed approach. We demonstrate that PCA2MA is highly competitive compared with other state-of-the-art techniques.
ناشر
Database: Elsevier - ScienceDirect (ساینس دایرکت)
Journal: Neurocomputing - Volume 185, 12 April 2016, Pages 113-132
Journal: Neurocomputing - Volume 185, 12 April 2016, Pages 113-132
نویسندگان
Jakub Nalepa, Michal Kawulok,