کد مقاله | کد نشریه | سال انتشار | مقاله انگلیسی | نسخه تمام متن |
---|---|---|---|---|
6866951 | 679667 | 2012 | 12 صفحه PDF | دانلود رایگان |
عنوان انگلیسی مقاله ISI
Locally linear embedding based on correntropy measure for visualization and classification
دانلود مقاله + سفارش ترجمه
دانلود مقاله ISI انگلیسی
رایگان برای ایرانیان
کلمات کلیدی
موضوعات مرتبط
مهندسی و علوم پایه
مهندسی کامپیوتر
هوش مصنوعی
پیش نمایش صفحه اول مقاله
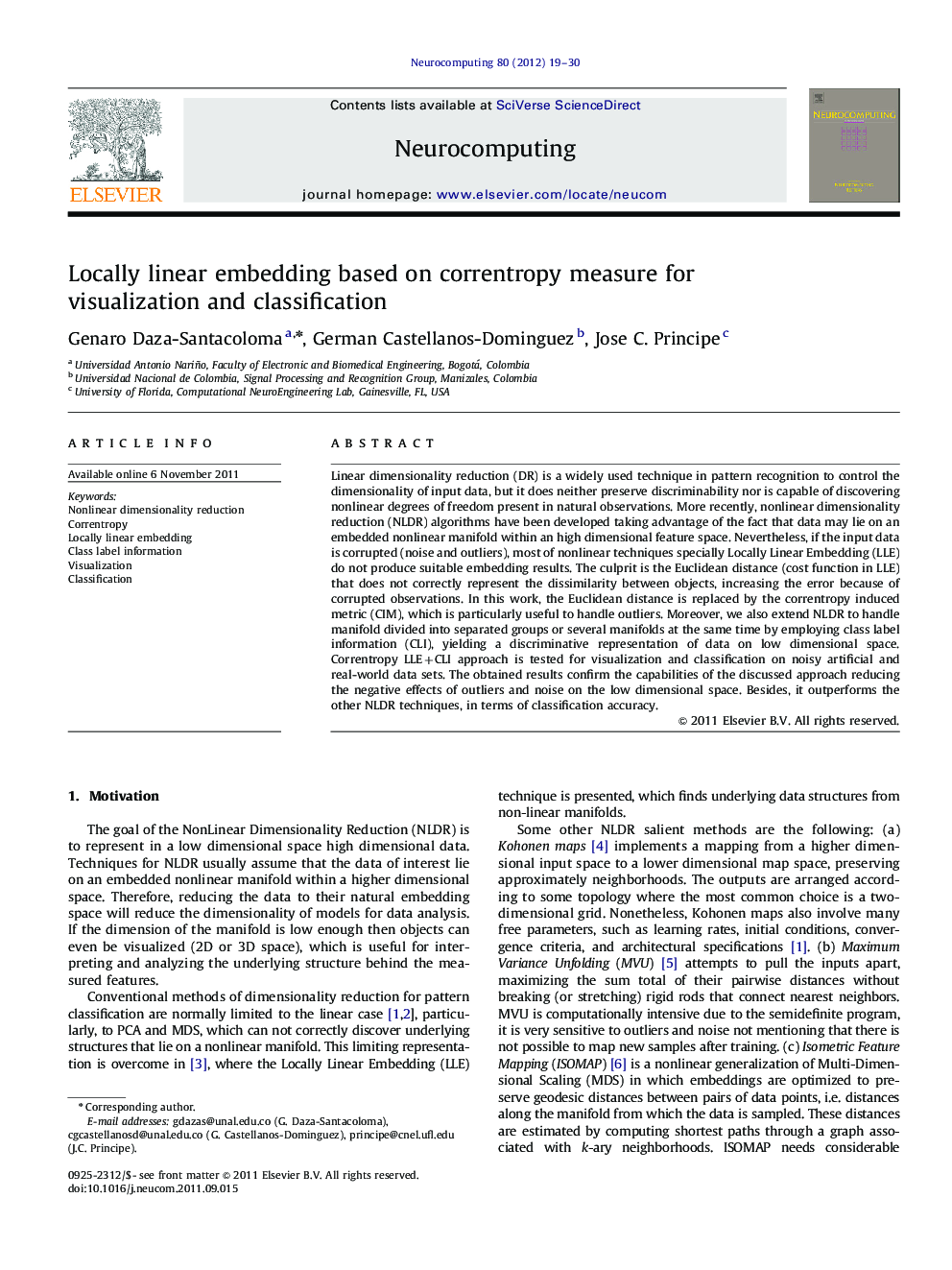
چکیده انگلیسی
Linear dimensionality reduction (DR) is a widely used technique in pattern recognition to control the dimensionality of input data, but it does neither preserve discriminability nor is capable of discovering nonlinear degrees of freedom present in natural observations. More recently, nonlinear dimensionality reduction (NLDR) algorithms have been developed taking advantage of the fact that data may lie on an embedded nonlinear manifold within an high dimensional feature space. Nevertheless, if the input data is corrupted (noise and outliers), most of nonlinear techniques specially Locally Linear Embedding (LLE) do not produce suitable embedding results. The culprit is the Euclidean distance (cost function in LLE) that does not correctly represent the dissimilarity between objects, increasing the error because of corrupted observations. In this work, the Euclidean distance is replaced by the correntropy induced metric (CIM), which is particularly useful to handle outliers. Moreover, we also extend NLDR to handle manifold divided into separated groups or several manifolds at the same time by employing class label information (CLI), yielding a discriminative representation of data on low dimensional space. Correntropy LLE+CLI approach is tested for visualization and classification on noisy artificial and real-world data sets. The obtained results confirm the capabilities of the discussed approach reducing the negative effects of outliers and noise on the low dimensional space. Besides, it outperforms the other NLDR techniques, in terms of classification accuracy.
ناشر
Database: Elsevier - ScienceDirect (ساینس دایرکت)
Journal: Neurocomputing - Volume 80, 15 March 2012, Pages 19-30
Journal: Neurocomputing - Volume 80, 15 March 2012, Pages 19-30
نویسندگان
Genaro Daza-Santacoloma, German Castellanos-Dominguez, Jose C. Principe,