کد مقاله | کد نشریه | سال انتشار | مقاله انگلیسی | نسخه تمام متن |
---|---|---|---|---|
6960664 | 1452001 | 2018 | 20 صفحه PDF | دانلود رایگان |
عنوان انگلیسی مقاله ISI
Automatic segmentation of speech articulators from real-time midsagittal MRI based on supervised learning
دانلود مقاله + سفارش ترجمه
دانلود مقاله ISI انگلیسی
رایگان برای ایرانیان
کلمات کلیدی
real-time MRISPFAAMFpsMLRROIASMEMARMSMSDPCA - PCAMRI - امآرآی یا تصویرسازی تشدید مغناطیسیPrincipal components analysis - تجزیه و تحلیل اجزای اصلیMagnetic resonance imaging - تصویربرداری رزونانس مغناطیسیHogs - توت هاMultiple linear regression - رگرسیون خطی چندگانه Speech articulation - سخن گفتنActive shape models - مدل های شکل فعالActive appearance models - مدل های ظاهر فعالElectromagnetic articulography - مقالات الکترومغناطیسیregion of interest - منطقه مورد نظرSURF - موج سواریroot mean square - میانگین مربع ریشهhistogram of oriented gradients - هیستوگرام گرادیان گرا
موضوعات مرتبط
مهندسی و علوم پایه
مهندسی کامپیوتر
پردازش سیگنال
پیش نمایش صفحه اول مقاله
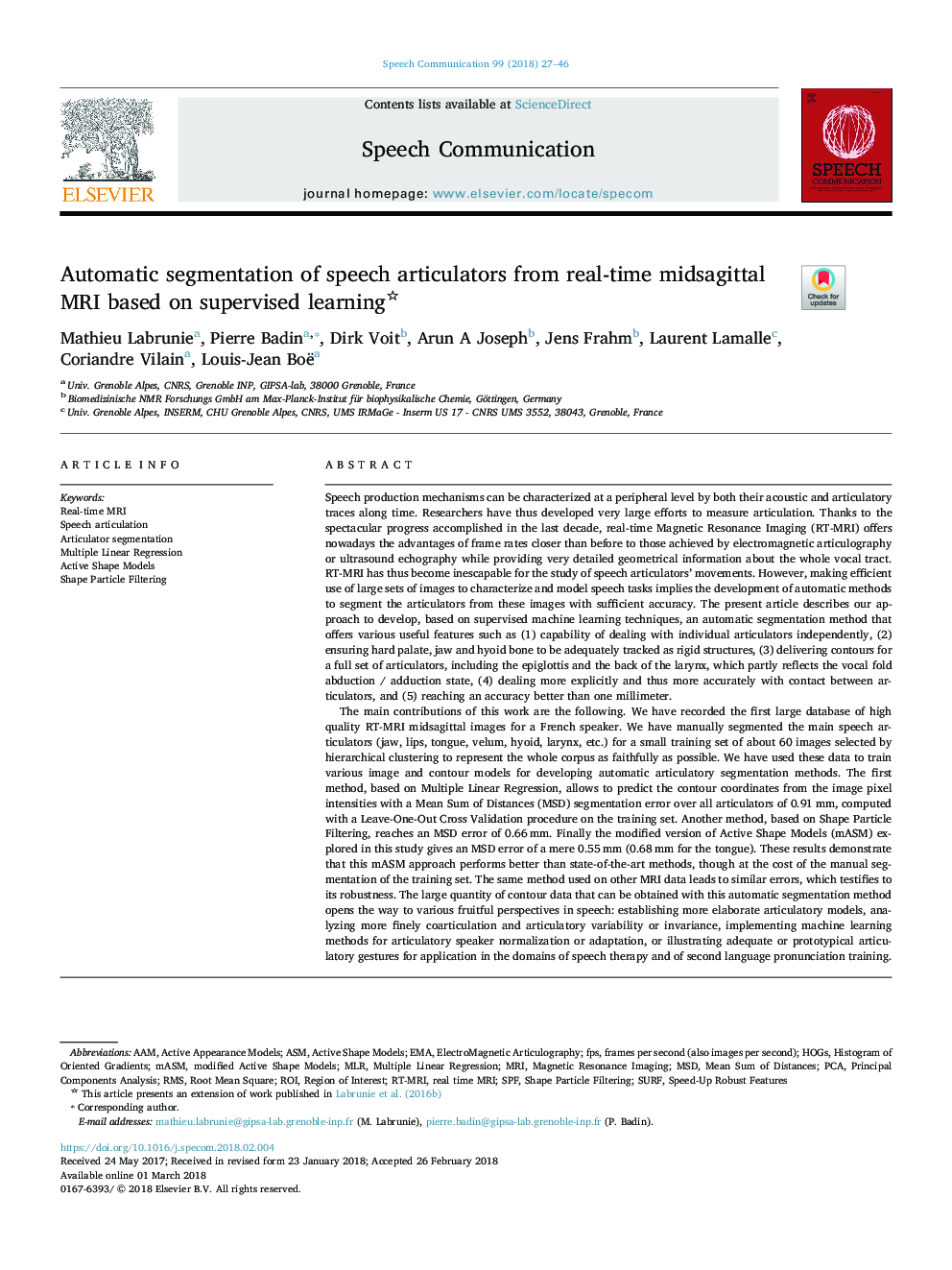
چکیده انگلیسی
The main contributions of this work are the following. We have recorded the first large database of high quality RT-MRI midsagittal images for a French speaker. We have manually segmented the main speech articulators (jaw, lips, tongue, velum, hyoid, larynx, etc.) for a small training set of about 60 images selected by hierarchical clustering to represent the whole corpus as faithfully as possible. We have used these data to train various image and contour models for developing automatic articulatory segmentation methods. The first method, based on Multiple Linear Regression, allows to predict the contour coordinates from the image pixel intensities with a Mean Sum of Distances (MSD) segmentation error over all articulators of 0.91â¯mm, computed with a Leave-One-Out Cross Validation procedure on the training set. Another method, based on Shape Particle Filtering, reaches an MSD error of 0.66â¯mm. Finally the modified version of Active Shape Models (mASM) explored in this study gives an MSD error of a mere 0.55â¯mm (0.68â¯mm for the tongue). These results demonstrate that this mASM approach performs better than state-of-the-art methods, though at the cost of the manual segmentation of the training set. The same method used on other MRI data leads to similar errors, which testifies to its robustness. The large quantity of contour data that can be obtained with this automatic segmentation method opens the way to various fruitful perspectives in speech: establishing more elaborate articulatory models, analyzing more finely coarticulation and articulatory variability or invariance, implementing machine learning methods for articulatory speaker normalization or adaptation, or illustrating adequate or prototypical articulatory gestures for application in the domains of speech therapy and of second language pronunciation training.
ناشر
Database: Elsevier - ScienceDirect (ساینس دایرکت)
Journal: Speech Communication - Volume 99, May 2018, Pages 27-46
Journal: Speech Communication - Volume 99, May 2018, Pages 27-46
نویسندگان
Mathieu Labrunie, Pierre Badin, Dirk Voit, Arun A Joseph, Jens Frahm, Laurent Lamalle, Coriandre Vilain, Louis-Jean Boë,