کد مقاله | کد نشریه | سال انتشار | مقاله انگلیسی | نسخه تمام متن |
---|---|---|---|---|
725738 | 1461241 | 2012 | 7 صفحه PDF | دانلود رایگان |
عنوان انگلیسی مقاله ISI
Indoor localization via â1-graph regularized semi-supervised manifold learning
دانلود مقاله + سفارش ترجمه
دانلود مقاله ISI انگلیسی
رایگان برای ایرانیان
کلمات کلیدی
موضوعات مرتبط
مهندسی و علوم پایه
سایر رشته های مهندسی
مهندسی برق و الکترونیک
پیش نمایش صفحه اول مقاله
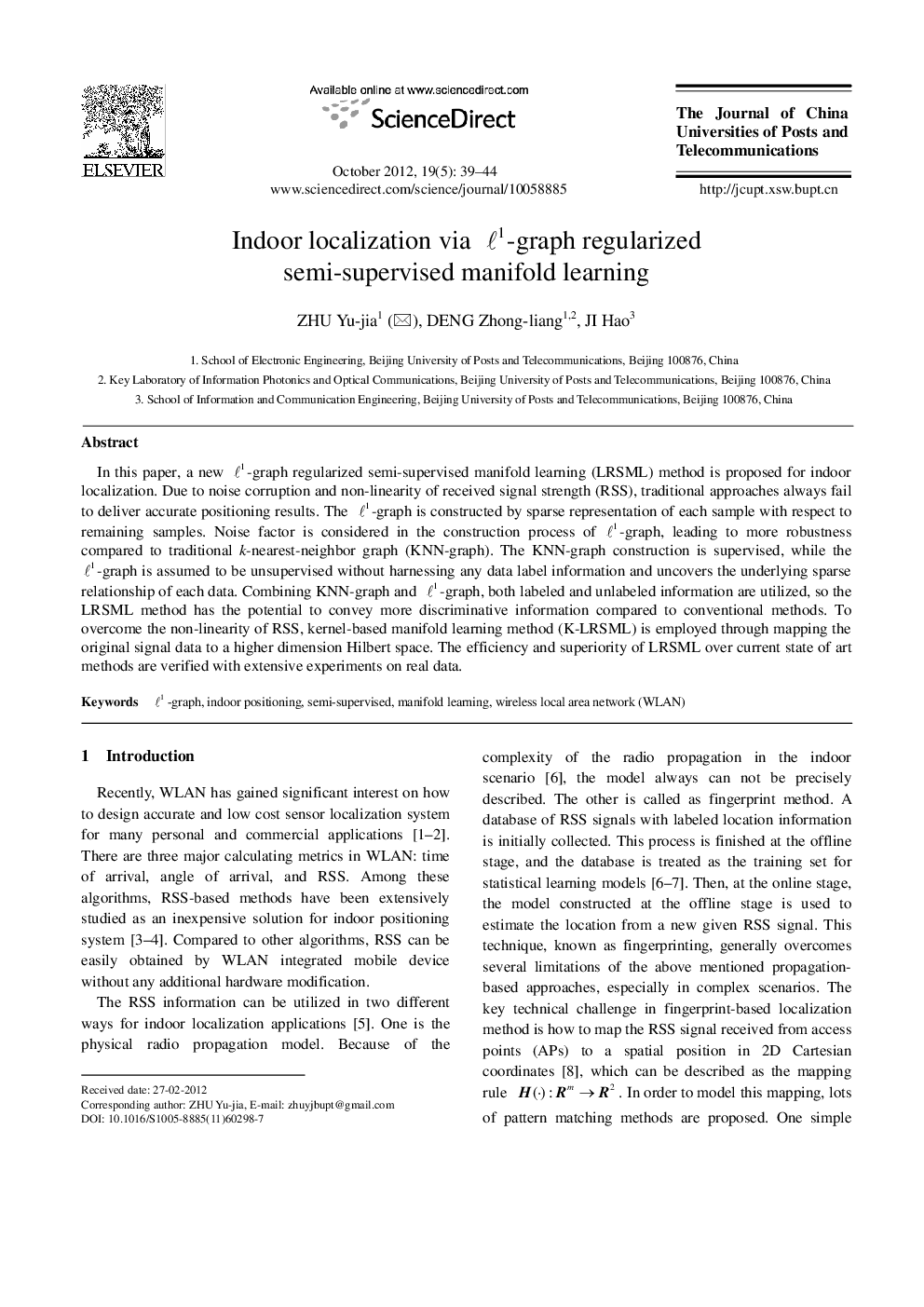
چکیده انگلیسی
In this paper, a new â1-graph regularized semi-supervised manifold learning (LRSML) method is proposed for indoor localization. Due to noise corruption and non-linearity of received signal strength (RSS), traditional approaches always fail to deliver accurate positioning results. The â1-graph is constructed by sparse representation of each sample with respect to remaining samples. Noise factor is considered in the construction process of â1-graph, leading to more robustness compared to traditional k-nearest-neighbor graph (KNN-graph). The KNN-graph construction is supervised, while the â1-graph is assumed to be unsupervised without harnessing any data label information and uncovers the underlying sparse relationship of each data. Combining KNN-graph and â1-graph, both labeled and unlabeled information are utilized, so the LRSML method has the potential to convey more discriminative information compared to conventional methods. To overcome the non-linearity of RSS, kernel-based manifold learning method (K-LRSML) is employed through mapping the original signal data to a higher dimension Hilbert space. The efficiency and superiority of LRSML over current state of art methods are verified with extensive experiments on real data.
ناشر
Database: Elsevier - ScienceDirect (ساینس دایرکت)
Journal: The Journal of China Universities of Posts and Telecommunications - Volume 19, Issue 5, October 2012, Pages 39-44, 91
Journal: The Journal of China Universities of Posts and Telecommunications - Volume 19, Issue 5, October 2012, Pages 39-44, 91
نویسندگان
Yu-jia ZHU, Zhong-liang DENG, Hao JI,