کد مقاله | کد نشریه | سال انتشار | مقاله انگلیسی | نسخه تمام متن |
---|---|---|---|---|
973845 | 1480149 | 2015 | 15 صفحه PDF | دانلود رایگان |
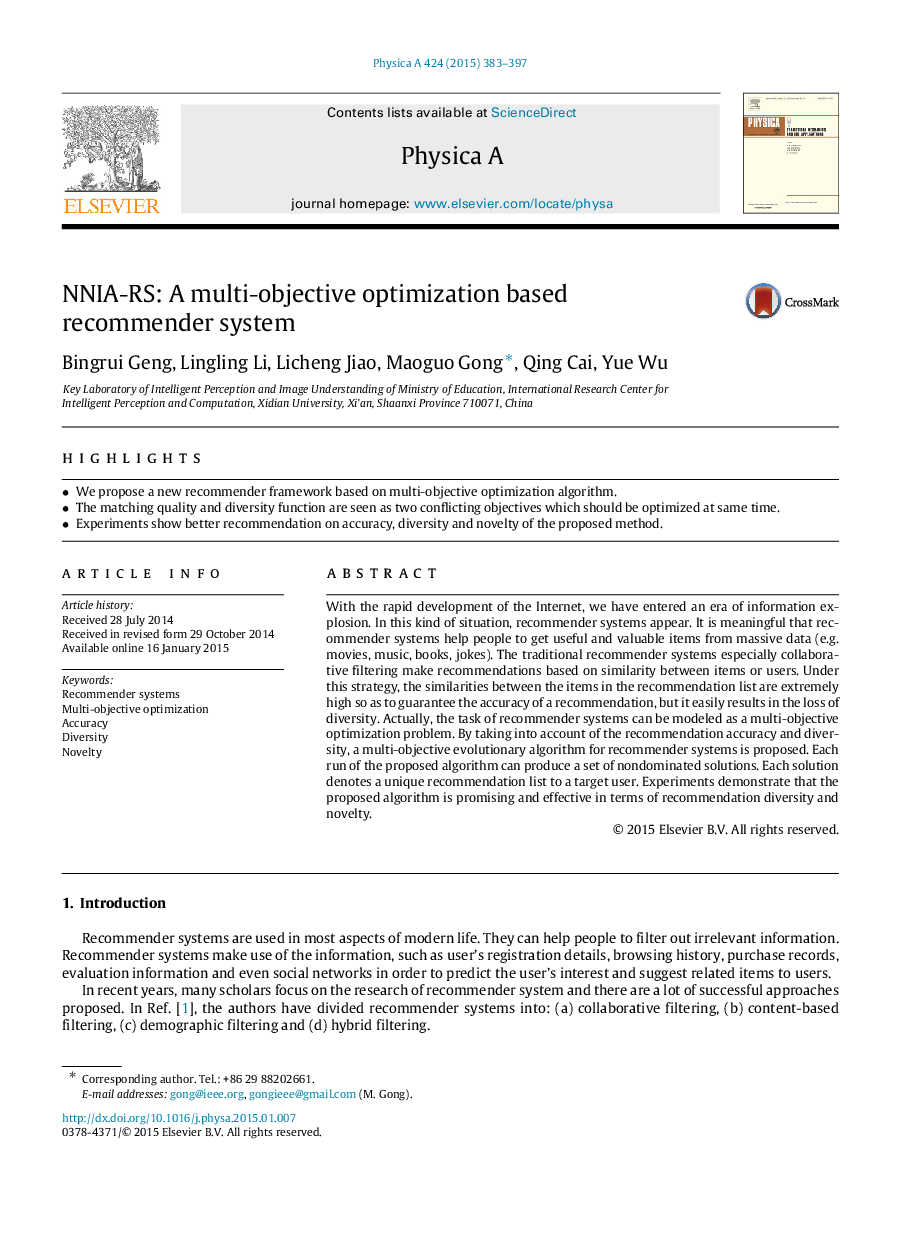
• We propose a new recommender framework based on multi-objective optimization algorithm.
• The matching quality and diversity function are seen as two conflicting objectives which should be optimized at same time.
• Experiments show better recommendation on accuracy, diversity and novelty of the proposed method.
With the rapid development of the Internet, we have entered an era of information explosion. In this kind of situation, recommender systems appear. It is meaningful that recommender systems help people to get useful and valuable items from massive data (e.g. movies, music, books, jokes). The traditional recommender systems especially collaborative filtering make recommendations based on similarity between items or users. Under this strategy, the similarities between the items in the recommendation list are extremely high so as to guarantee the accuracy of a recommendation, but it easily results in the loss of diversity. Actually, the task of recommender systems can be modeled as a multi-objective optimization problem. By taking into account of the recommendation accuracy and diversity, a multi-objective evolutionary algorithm for recommender systems is proposed. Each run of the proposed algorithm can produce a set of nondominated solutions. Each solution denotes a unique recommendation list to a target user. Experiments demonstrate that the proposed algorithm is promising and effective in terms of recommendation diversity and novelty.
Journal: Physica A: Statistical Mechanics and its Applications - Volume 424, 15 April 2015, Pages 383–397