کد مقاله | کد نشریه | سال انتشار | مقاله انگلیسی | نسخه تمام متن |
---|---|---|---|---|
10508474 | 948487 | 2015 | 23 صفحه PDF | دانلود رایگان |
عنوان انگلیسی مقاله ISI
Evaluating machine learning approaches for the interpolation of monthly air temperature at Mt. Kilimanjaro, Tanzania
دانلود مقاله + سفارش ترجمه
دانلود مقاله ISI انگلیسی
رایگان برای ایرانیان
کلمات کلیدی
موضوعات مرتبط
مهندسی و علوم پایه
علوم زمین و سیارات
علوم زمین و سیاره ای (عمومی)
پیش نمایش صفحه اول مقاله
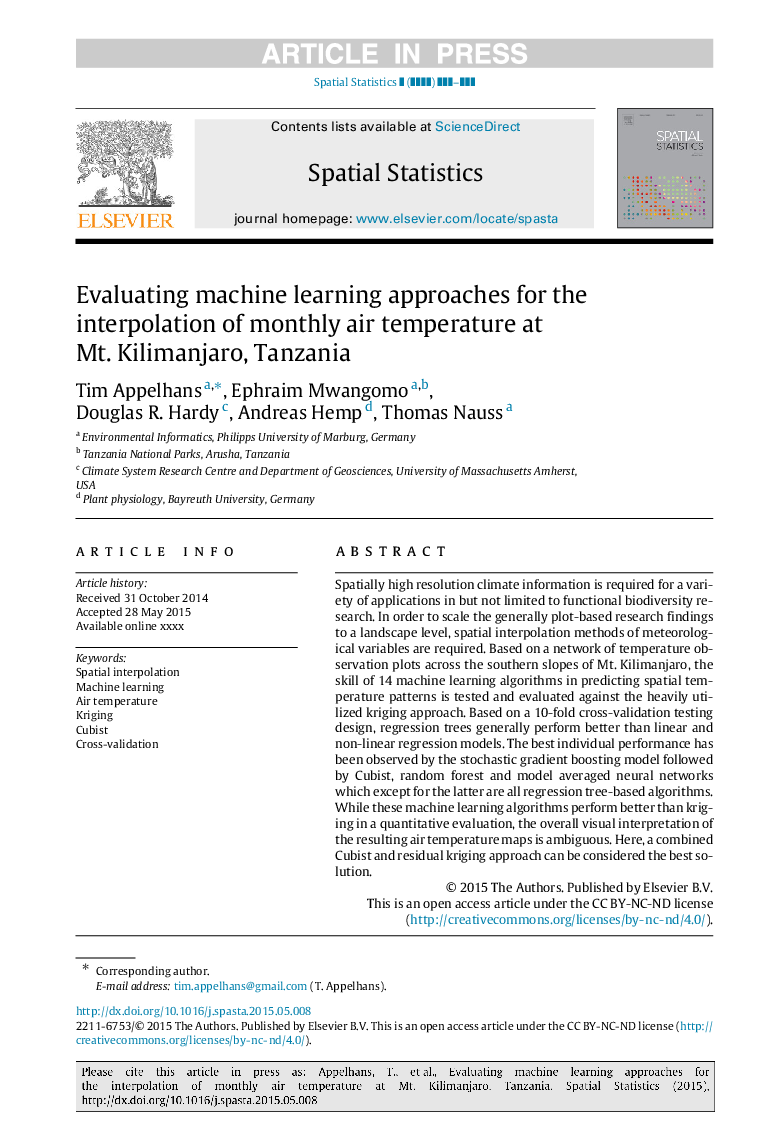
چکیده انگلیسی
Spatially high resolution climate information is required for a variety of applications in but not limited to functional biodiversity research. In order to scale the generally plot-based research findings to a landscape level, spatial interpolation methods of meteorological variables are required. Based on a network of temperature observation plots across the southern slopes of Mt. Kilimanjaro, the skill of 14 machine learning algorithms in predicting spatial temperature patterns is tested and evaluated against the heavily utilized kriging approach. Based on a 10-fold cross-validation testing design, regression trees generally perform better than linear and non-linear regression models. The best individual performance has been observed by the stochastic gradient boosting model followed by Cubist, random forest and model averaged neural networks which except for the latter are all regression tree-based algorithms. While these machine learning algorithms perform better than kriging in a quantitative evaluation, the overall visual interpretation of the resulting air temperature maps is ambiguous. Here, a combined Cubist and residual kriging approach can be considered the best solution.
ناشر
Database: Elsevier - ScienceDirect (ساینس دایرکت)
Journal: Spatial Statistics - Volume 14, Part A, November 2015, Pages 91-113
Journal: Spatial Statistics - Volume 14, Part A, November 2015, Pages 91-113
نویسندگان
Tim Appelhans, Ephraim Mwangomo, Douglas R. Hardy, Andreas Hemp, Thomas Nauss,