کد مقاله | کد نشریه | سال انتشار | مقاله انگلیسی | نسخه تمام متن |
---|---|---|---|---|
1170973 | 960698 | 2007 | 10 صفحه PDF | دانلود رایگان |
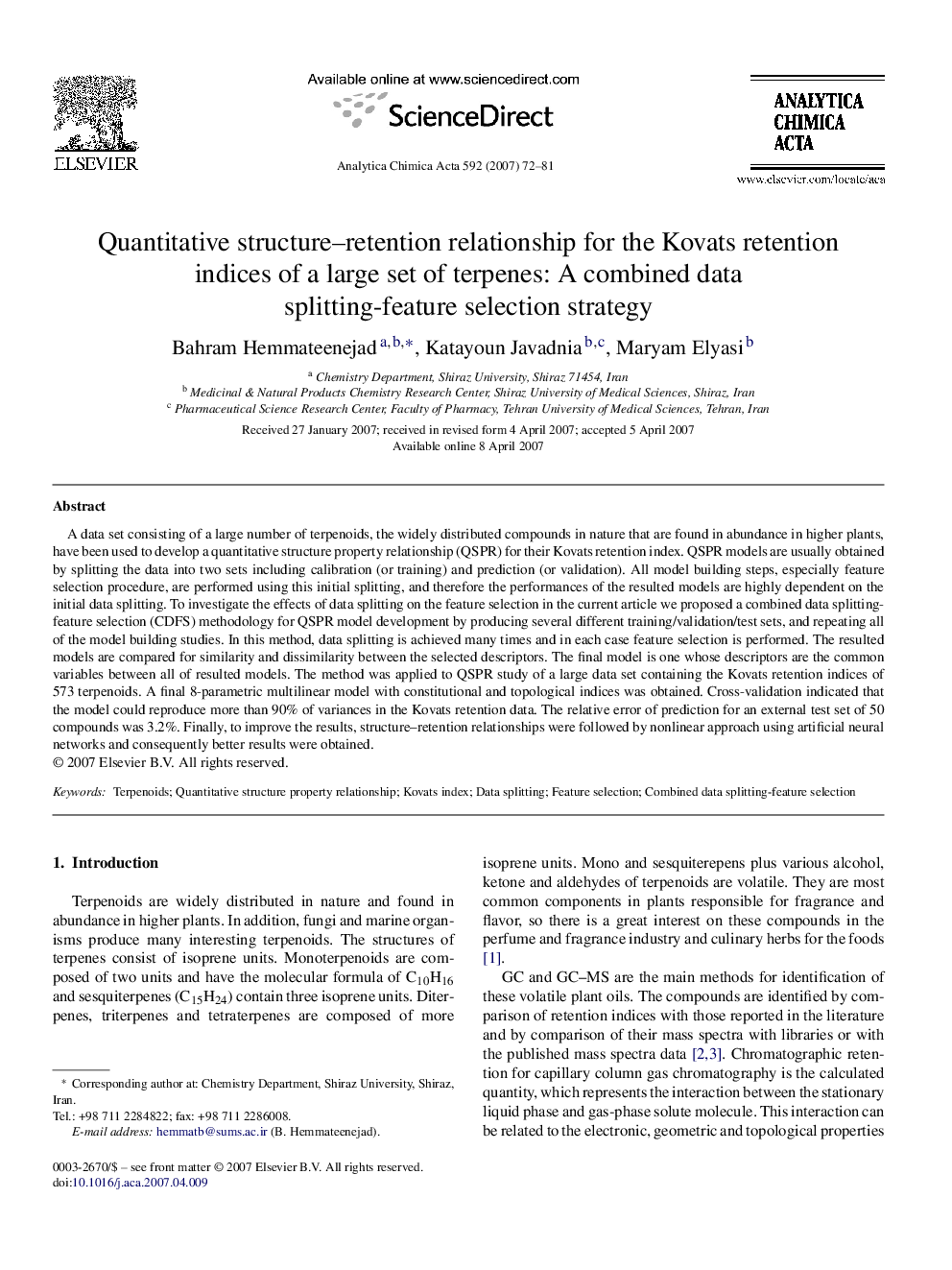
A data set consisting of a large number of terpenoids, the widely distributed compounds in nature that are found in abundance in higher plants, have been used to develop a quantitative structure property relationship (QSPR) for their Kovats retention index. QSPR models are usually obtained by splitting the data into two sets including calibration (or training) and prediction (or validation). All model building steps, especially feature selection procedure, are performed using this initial splitting, and therefore the performances of the resulted models are highly dependent on the initial data splitting. To investigate the effects of data splitting on the feature selection in the current article we proposed a combined data splitting-feature selection (CDFS) methodology for QSPR model development by producing several different training/validation/test sets, and repeating all of the model building studies. In this method, data splitting is achieved many times and in each case feature selection is performed. The resulted models are compared for similarity and dissimilarity between the selected descriptors. The final model is one whose descriptors are the common variables between all of resulted models. The method was applied to QSPR study of a large data set containing the Kovats retention indices of 573 terpenoids. A final 8-parametric multilinear model with constitutional and topological indices was obtained. Cross-validation indicated that the model could reproduce more than 90% of variances in the Kovats retention data. The relative error of prediction for an external test set of 50 compounds was 3.2%. Finally, to improve the results, structure–retention relationships were followed by nonlinear approach using artificial neural networks and consequently better results were obtained.
Journal: Analytica Chimica Acta - Volume 592, Issue 1, 29 May 2007, Pages 72–81