کد مقاله | کد نشریه | سال انتشار | مقاله انگلیسی | نسخه تمام متن |
---|---|---|---|---|
1179692 | 1491541 | 2014 | 14 صفحه PDF | دانلود رایگان |
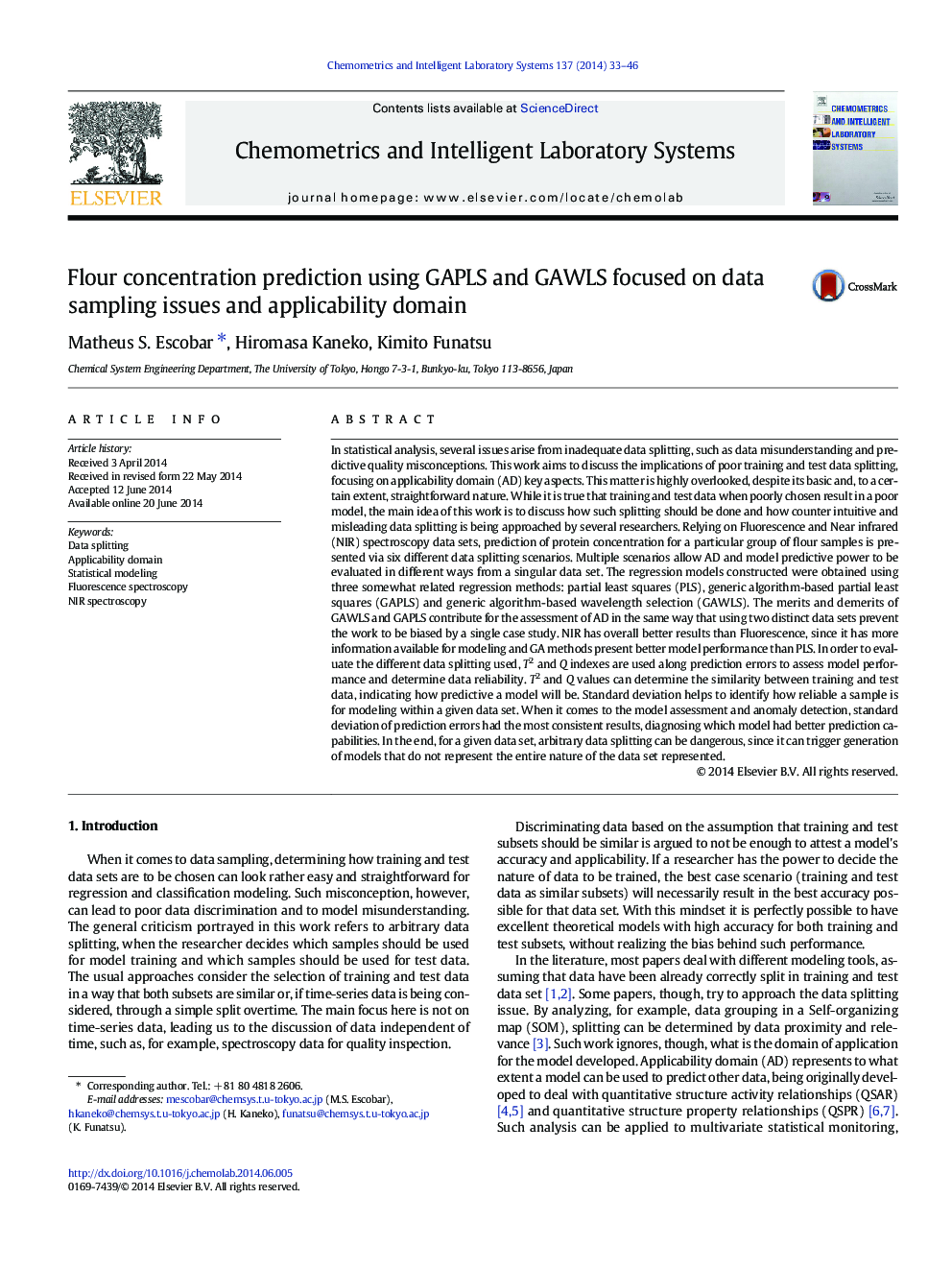
• Incorrect data splitting may lead to misleading modeling.
• Applicability domain investigation is fundamental for proper modeling.
• NIR models had better results in this work than fluorescence spectroscopy models.
• T2 and Q statistics are not reliable approaches for model assessment.
• Standard deviation of prediction errors is a valid approach for model assessment.
In statistical analysis, several issues arise from inadequate data splitting, such as data misunderstanding and predictive quality misconceptions. This work aims to discuss the implications of poor training and test data splitting, focusing on applicability domain (AD) key aspects. This matter is highly overlooked, despite its basic and, to a certain extent, straightforward nature. While it is true that training and test data when poorly chosen result in a poor model, the main idea of this work is to discuss how such splitting should be done and how counter intuitive and misleading data splitting is being approached by several researchers. Relying on Fluorescence and Near infrared (NIR) spectroscopy data sets, prediction of protein concentration for a particular group of flour samples is presented via six different data splitting scenarios. Multiple scenarios allow AD and model predictive power to be evaluated in different ways from a singular data set. The regression models constructed were obtained using three somewhat related regression methods: partial least squares (PLS), generic algorithm-based partial least squares (GAPLS) and generic algorithm-based wavelength selection (GAWLS). The merits and demerits of GAWLS and GAPLS contribute for the assessment of AD in the same way that using two distinct data sets prevent the work to be biased by a single case study. NIR has overall better results than Fluorescence, since it has more information available for modeling and GA methods present better model performance than PLS. In order to evaluate the different data splitting used, T2 and Q indexes are used along prediction errors to assess model performance and determine data reliability. T2 and Q values can determine the similarity between training and test data, indicating how predictive a model will be. Standard deviation helps to identify how reliable a sample is for modeling within a given data set. When it comes to the model assessment and anomaly detection, standard deviation of prediction errors had the most consistent results, diagnosing which model had better prediction capabilities. In the end, for a given data set, arbitrary data splitting can be dangerous, since it can trigger generation of models that do not represent the entire nature of the data set represented.
Journal: Chemometrics and Intelligent Laboratory Systems - Volume 137, 15 October 2014, Pages 33–46