کد مقاله | کد نشریه | سال انتشار | مقاله انگلیسی | نسخه تمام متن |
---|---|---|---|---|
1180525 | 1491536 | 2015 | 14 صفحه PDF | دانلود رایگان |
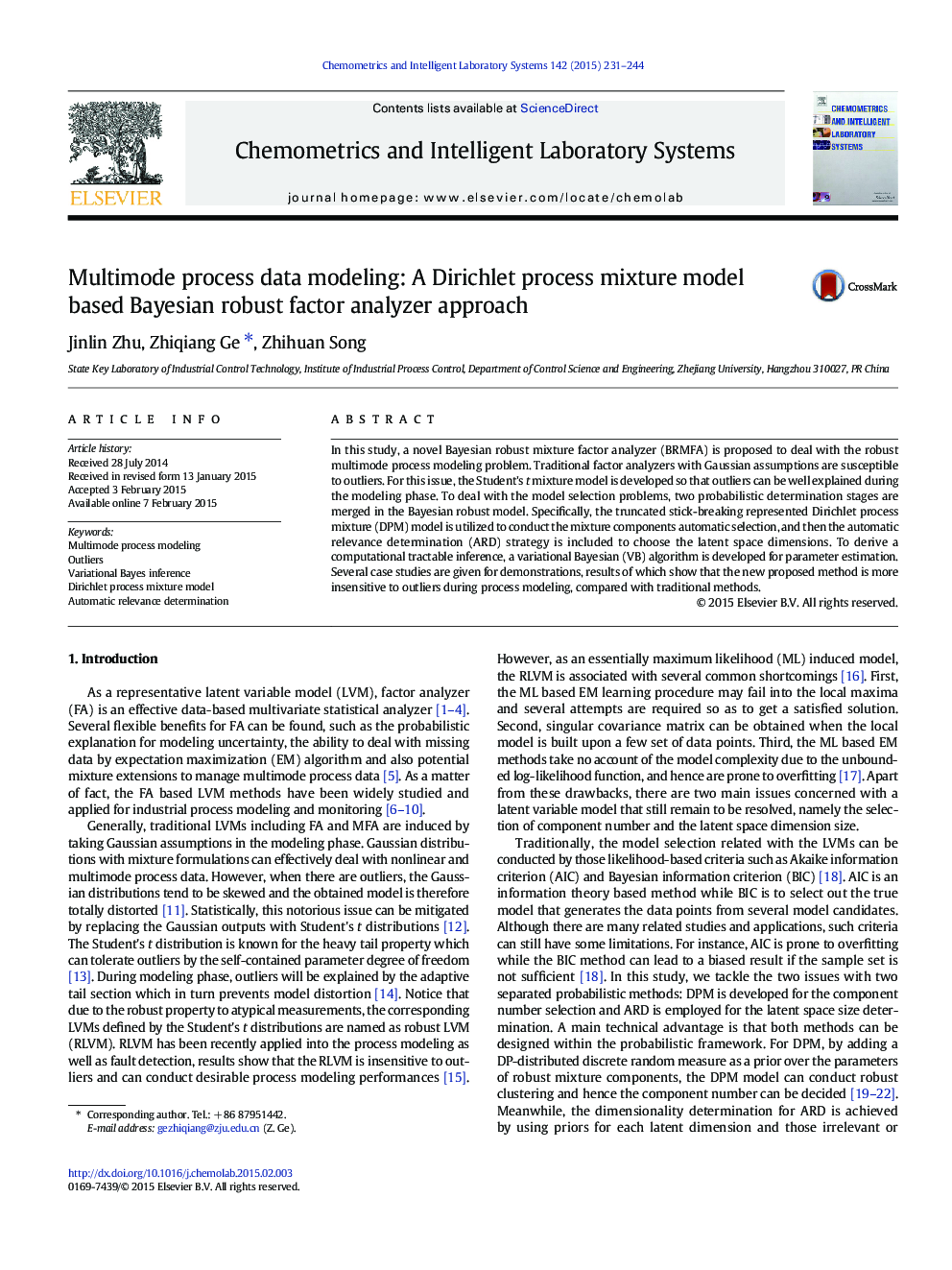
• A novel Bayesian robust mixture factor analyzer is proposed to deal with the robust multimode process modeling problem.
• The Student’s t mixture model is developed so that outliers can be well explained during the modeling phase.
• A Dirichlet process mixture model is utilized to conduct the mixture components automatic selection.
• The automatic relevance determination strategy is included to choose the latent space dimensions.
• A variational Bayesian algorithm is developed for parameter estimation.
In this study, a novel Bayesian robust mixture factor analyzer (BRMFA) is proposed to deal with the robust multimode process modeling problem. Traditional factor analyzers with Gaussian assumptions are susceptible to outliers. For this issue, the Student's t mixture model is developed so that outliers can be well explained during the modeling phase. To deal with the model selection problems, two probabilistic determination stages are merged in the Bayesian robust model. Specifically, the truncated stick-breaking represented Dirichlet process mixture (DPM) model is utilized to conduct the mixture components automatic selection, and then the automatic relevance determination (ARD) strategy is included to choose the latent space dimensions. To derive a computational tractable inference, a variational Bayesian (VB) algorithm is developed for parameter estimation. Several case studies are given for demonstrations, results of which show that the new proposed method is more insensitive to outliers during process modeling, compared with traditional methods.
Journal: Chemometrics and Intelligent Laboratory Systems - Volume 142, 15 March 2015, Pages 231–244