کد مقاله | کد نشریه | سال انتشار | مقاله انگلیسی | نسخه تمام متن |
---|---|---|---|---|
1180561 | 1491535 | 2015 | 12 صفحه PDF | دانلود رایگان |
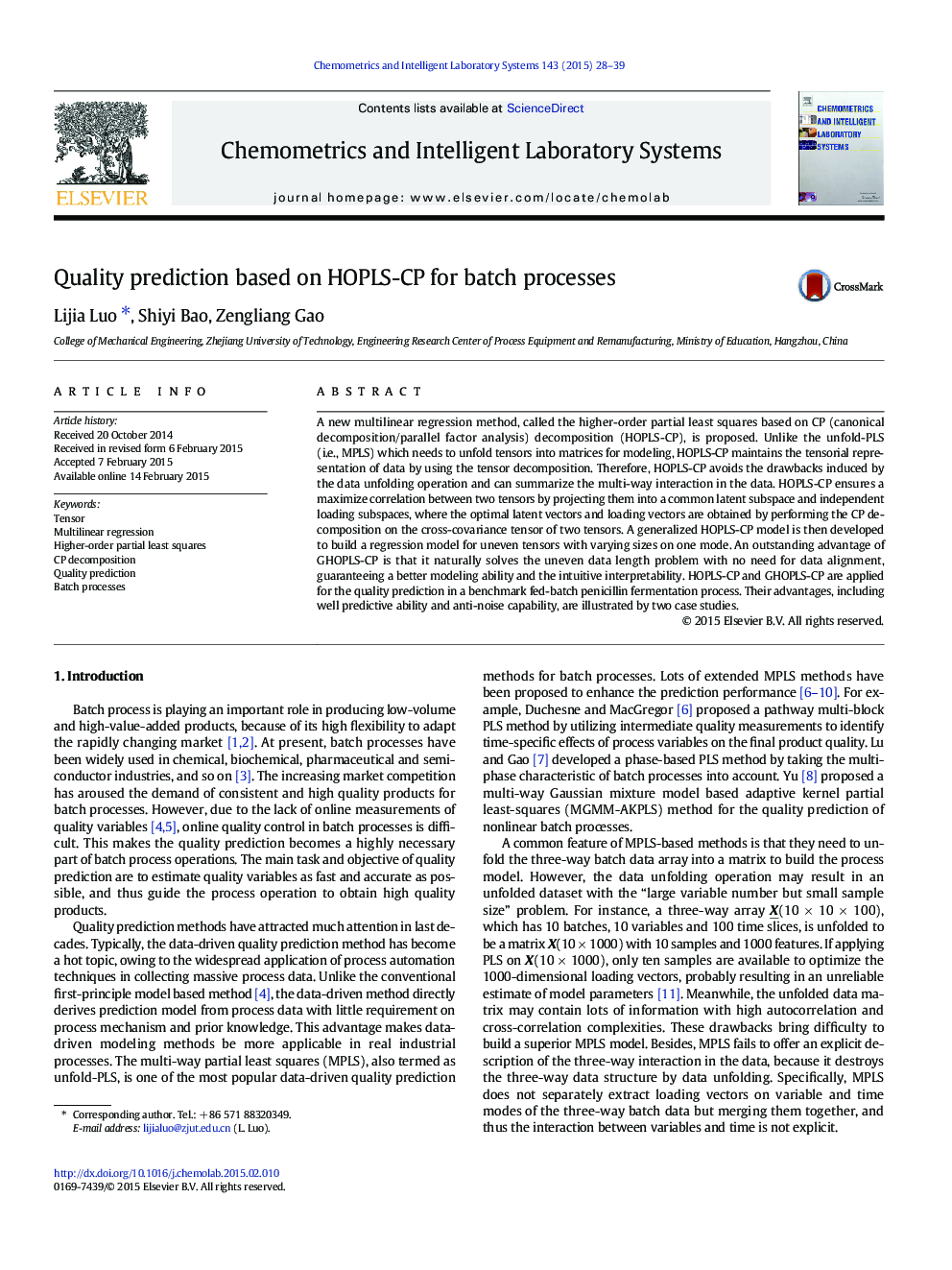
• A new multilinear regression method named HOPLS-CP is proposed.
• A generalized HOPLS-CP (GHOPLS-CP) model is developed for uneven tensors.
• HOPLS-CP and GHOPLS-CP are applied for quality prediction in a batch process.
• HOPLS-CP and GHOPLS-CP have well predictive ability and anti-noise capability.
A new multilinear regression method, called the higher-order partial least squares based on CP (canonical decomposition/parallel factor analysis) decomposition (HOPLS-CP), is proposed. Unlike the unfold-PLS (i.e., MPLS) which needs to unfold tensors into matrices for modeling, HOPLS-CP maintains the tensorial representation of data by using the tensor decomposition. Therefore, HOPLS-CP avoids the drawbacks induced by the data unfolding operation and can summarize the multi-way interaction in the data. HOPLS-CP ensures a maximize correlation between two tensors by projecting them into a common latent subspace and independent loading subspaces, where the optimal latent vectors and loading vectors are obtained by performing the CP decomposition on the cross-covariance tensor of two tensors. A generalized HOPLS-CP model is then developed to build a regression model for uneven tensors with varying sizes on one mode. An outstanding advantage of GHOPLS-CP is that it naturally solves the uneven data length problem with no need for data alignment, guaranteeing a better modeling ability and the intuitive interpretability. HOPLS-CP and GHOPLS-CP are applied for the quality prediction in a benchmark fed-batch penicillin fermentation process. Their advantages, including well predictive ability and anti-noise capability, are illustrated by two case studies.
Journal: Chemometrics and Intelligent Laboratory Systems - Volume 143, 15 April 2015, Pages 28–39