کد مقاله | کد نشریه | سال انتشار | مقاله انگلیسی | نسخه تمام متن |
---|---|---|---|---|
1181040 | 962893 | 2011 | 16 صفحه PDF | دانلود رایگان |
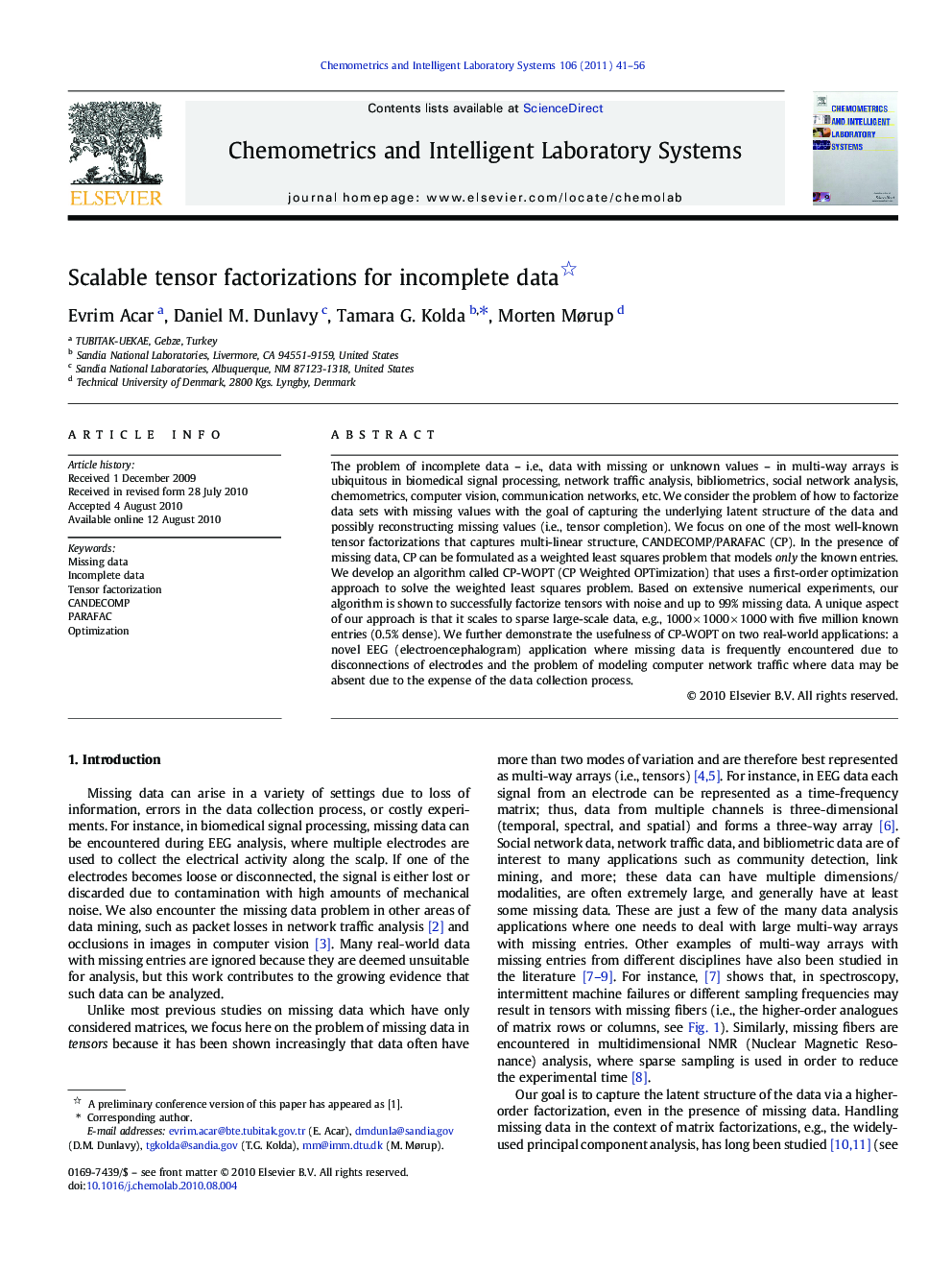
The problem of incomplete data – i.e., data with missing or unknown values – in multi-way arrays is ubiquitous in biomedical signal processing, network traffic analysis, bibliometrics, social network analysis, chemometrics, computer vision, communication networks, etc. We consider the problem of how to factorize data sets with missing values with the goal of capturing the underlying latent structure of the data and possibly reconstructing missing values (i.e., tensor completion). We focus on one of the most well-known tensor factorizations that captures multi-linear structure, CANDECOMP/PARAFAC (CP). In the presence of missing data, CP can be formulated as a weighted least squares problem that models only the known entries. We develop an algorithm called CP-WOPT (CP Weighted OPTimization) that uses a first-order optimization approach to solve the weighted least squares problem. Based on extensive numerical experiments, our algorithm is shown to successfully factorize tensors with noise and up to 99% missing data. A unique aspect of our approach is that it scales to sparse large-scale data, e.g., 1000 × 1000 × 1000 with five million known entries (0.5% dense). We further demonstrate the usefulness of CP-WOPT on two real-world applications: a novel EEG (electroencephalogram) application where missing data is frequently encountered due to disconnections of electrodes and the problem of modeling computer network traffic where data may be absent due to the expense of the data collection process.
Journal: Chemometrics and Intelligent Laboratory Systems - Volume 106, Issue 1, 15 March 2011, Pages 41–56