کد مقاله | کد نشریه | سال انتشار | مقاله انگلیسی | نسخه تمام متن |
---|---|---|---|---|
1181126 | 1491559 | 2012 | 11 صفحه PDF | دانلود رایگان |
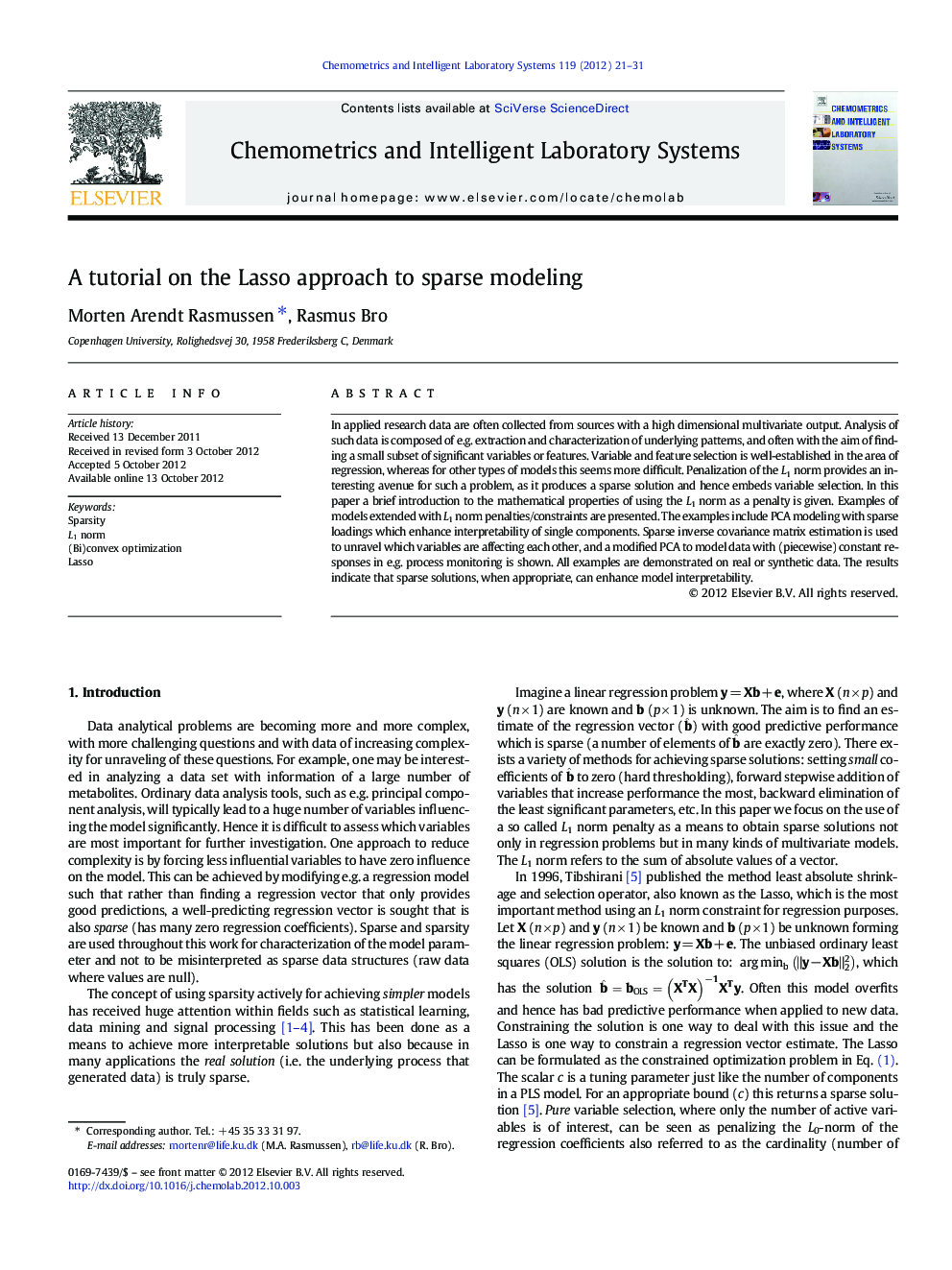
In applied research data are often collected from sources with a high dimensional multivariate output. Analysis of such data is composed of e.g. extraction and characterization of underlying patterns, and often with the aim of finding a small subset of significant variables or features. Variable and feature selection is well-established in the area of regression, whereas for other types of models this seems more difficult. Penalization of the L1 norm provides an interesting avenue for such a problem, as it produces a sparse solution and hence embeds variable selection. In this paper a brief introduction to the mathematical properties of using the L1 norm as a penalty is given. Examples of models extended with L1 norm penalties/constraints are presented. The examples include PCA modeling with sparse loadings which enhance interpretability of single components. Sparse inverse covariance matrix estimation is used to unravel which variables are affecting each other, and a modified PCA to model data with (piecewise) constant responses in e.g. process monitoring is shown. All examples are demonstrated on real or synthetic data. The results indicate that sparse solutions, when appropriate, can enhance model interpretability.
Journal: Chemometrics and Intelligent Laboratory Systems - Volume 119, 1 October 2012, Pages 21–31