کد مقاله | کد نشریه | سال انتشار | مقاله انگلیسی | نسخه تمام متن |
---|---|---|---|---|
1181433 | 1491555 | 2013 | 13 صفحه PDF | دانلود رایگان |
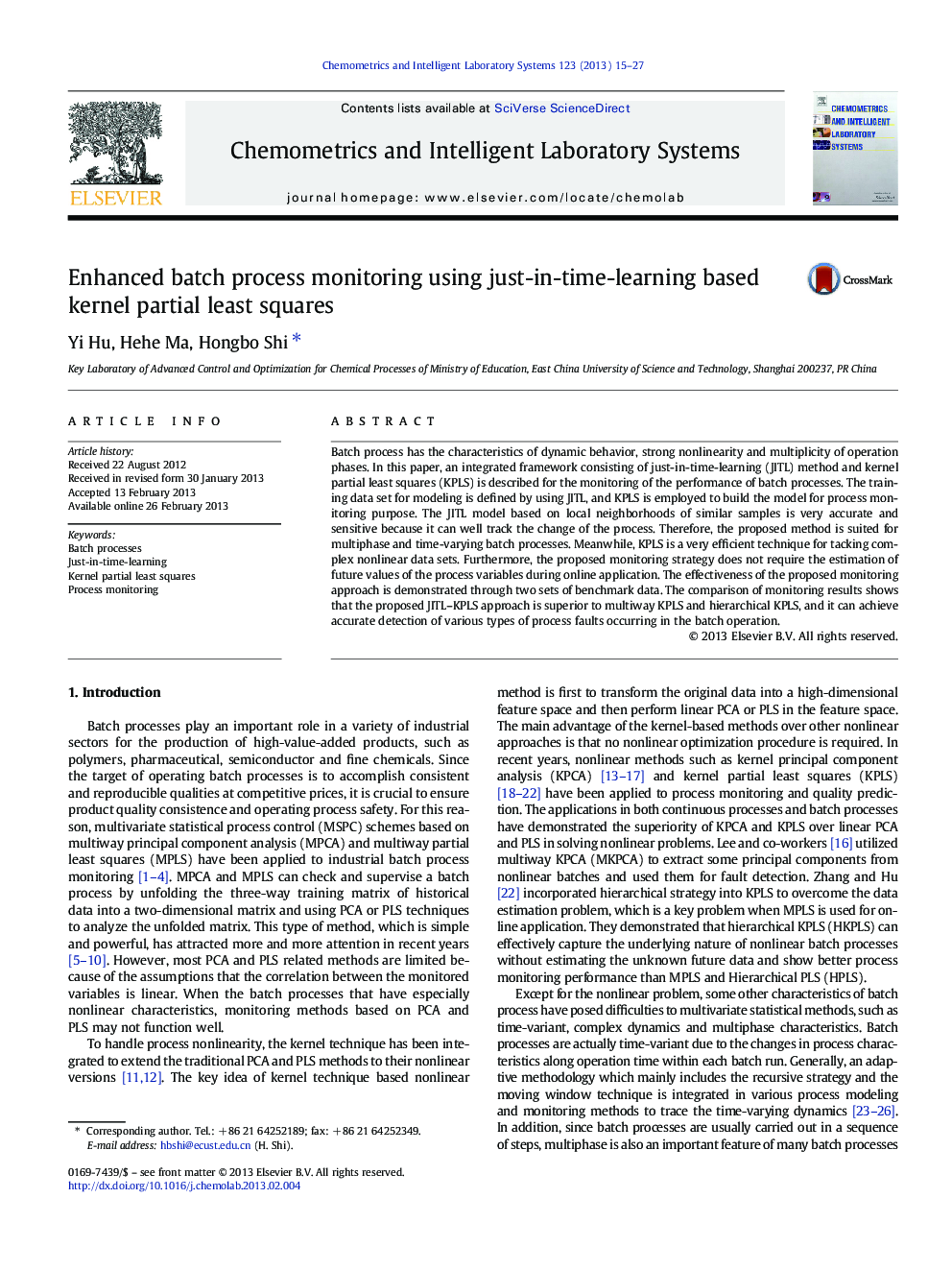
Batch process has the characteristics of dynamic behavior, strong nonlinearity and multiplicity of operation phases. In this paper, an integrated framework consisting of just-in-time-learning (JITL) method and kernel partial least squares (KPLS) is described for the monitoring of the performance of batch processes. The training data set for modeling is defined by using JITL, and KPLS is employed to build the model for process monitoring purpose. The JITL model based on local neighborhoods of similar samples is very accurate and sensitive because it can well track the change of the process. Therefore, the proposed method is suited for multiphase and time-varying batch processes. Meanwhile, KPLS is a very efficient technique for tacking complex nonlinear data sets. Furthermore, the proposed monitoring strategy does not require the estimation of future values of the process variables during online application. The effectiveness of the proposed monitoring approach is demonstrated through two sets of benchmark data. The comparison of monitoring results shows that the proposed JITL–KPLS approach is superior to multiway KPLS and hierarchical KPLS, and it can achieve accurate detection of various types of process faults occurring in the batch operation.
► An enhanced process monitoring framework for batch processes is proposed.
► The new local method is better than conventional multiway and hierarchical methods.
► The proposed method can handle the problem of uneven-length phases.
► We use a moving window strategy in order to satisfy the real-time requirement.
► Estimation of future values of the process variables is avoided.
Journal: Chemometrics and Intelligent Laboratory Systems - Volume 123, 15 April 2013, Pages 15–27