کد مقاله | کد نشریه | سال انتشار | مقاله انگلیسی | نسخه تمام متن |
---|---|---|---|---|
1931454 | 1050553 | 2010 | 6 صفحه PDF | دانلود رایگان |
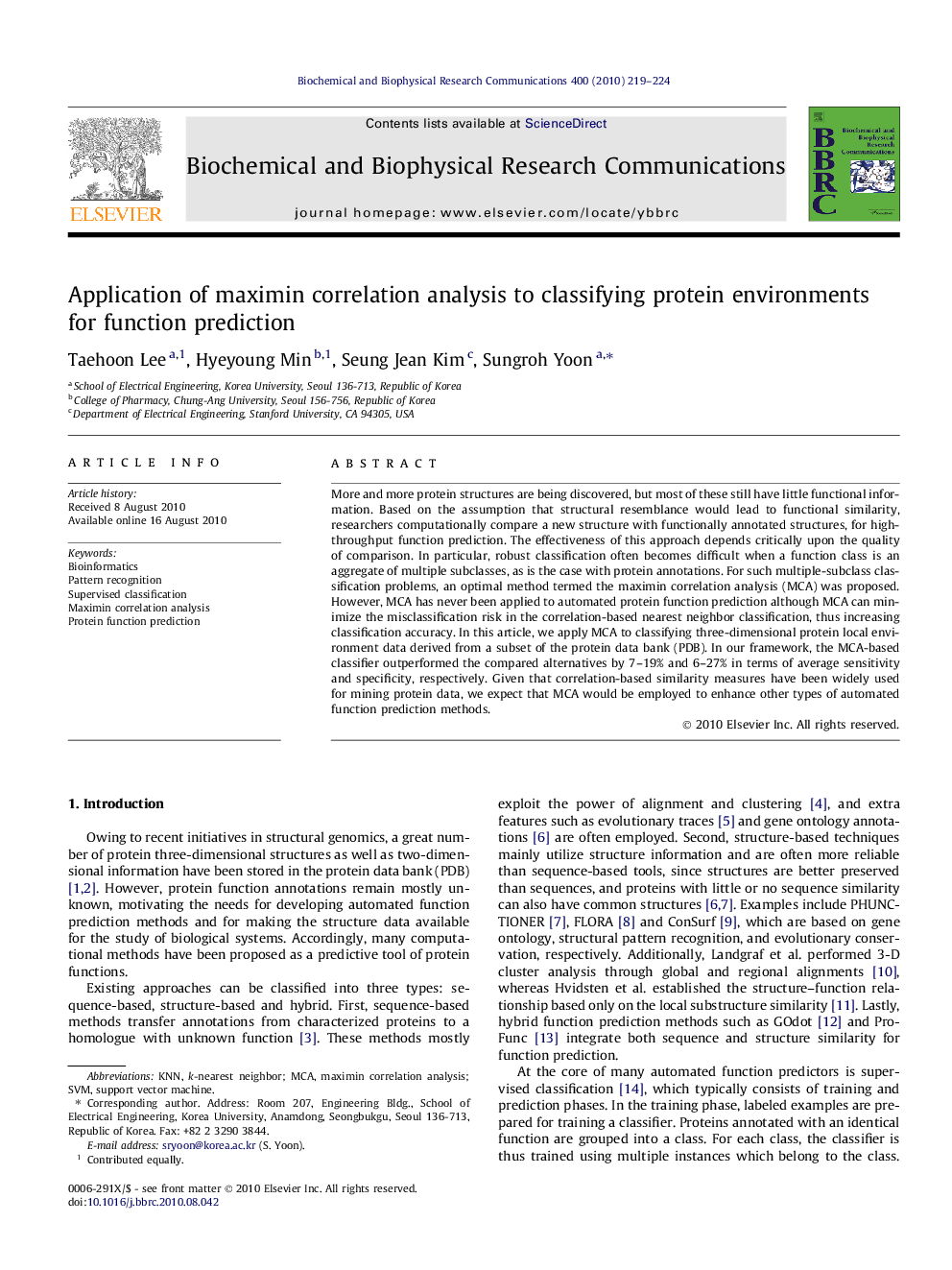
More and more protein structures are being discovered, but most of these still have little functional information. Based on the assumption that structural resemblance would lead to functional similarity, researchers computationally compare a new structure with functionally annotated structures, for high-throughput function prediction. The effectiveness of this approach depends critically upon the quality of comparison. In particular, robust classification often becomes difficult when a function class is an aggregate of multiple subclasses, as is the case with protein annotations. For such multiple-subclass classification problems, an optimal method termed the maximin correlation analysis (MCA) was proposed. However, MCA has never been applied to automated protein function prediction although MCA can minimize the misclassification risk in the correlation-based nearest neighbor classification, thus increasing classification accuracy. In this article, we apply MCA to classifying three-dimensional protein local environment data derived from a subset of the protein data bank (PDB). In our framework, the MCA-based classifier outperformed the compared alternatives by 7–19% and 6–27% in terms of average sensitivity and specificity, respectively. Given that correlation-based similarity measures have been widely used for mining protein data, we expect that MCA would be employed to enhance other types of automated function prediction methods.
Research highlights
► This is the first work applying maximin correlation analysis (MCA) to biological data.
► We applied MCA to 3-dimensional protein local environments to see its effectiveness.
► Our MCA-based approach outperformed the conventional state-of-the-art classifiers.
► The sensitivity and specificity of the MCA method were up to 27% better.
► Many other correlation-based biological data analyzers can also be improved by MCA.
Journal: Biochemical and Biophysical Research Communications - Volume 400, Issue 2, 17 September 2010, Pages 219–224