کد مقاله | کد نشریه | سال انتشار | مقاله انگلیسی | نسخه تمام متن |
---|---|---|---|---|
431891 | 688648 | 2013 | 12 صفحه PDF | دانلود رایگان |
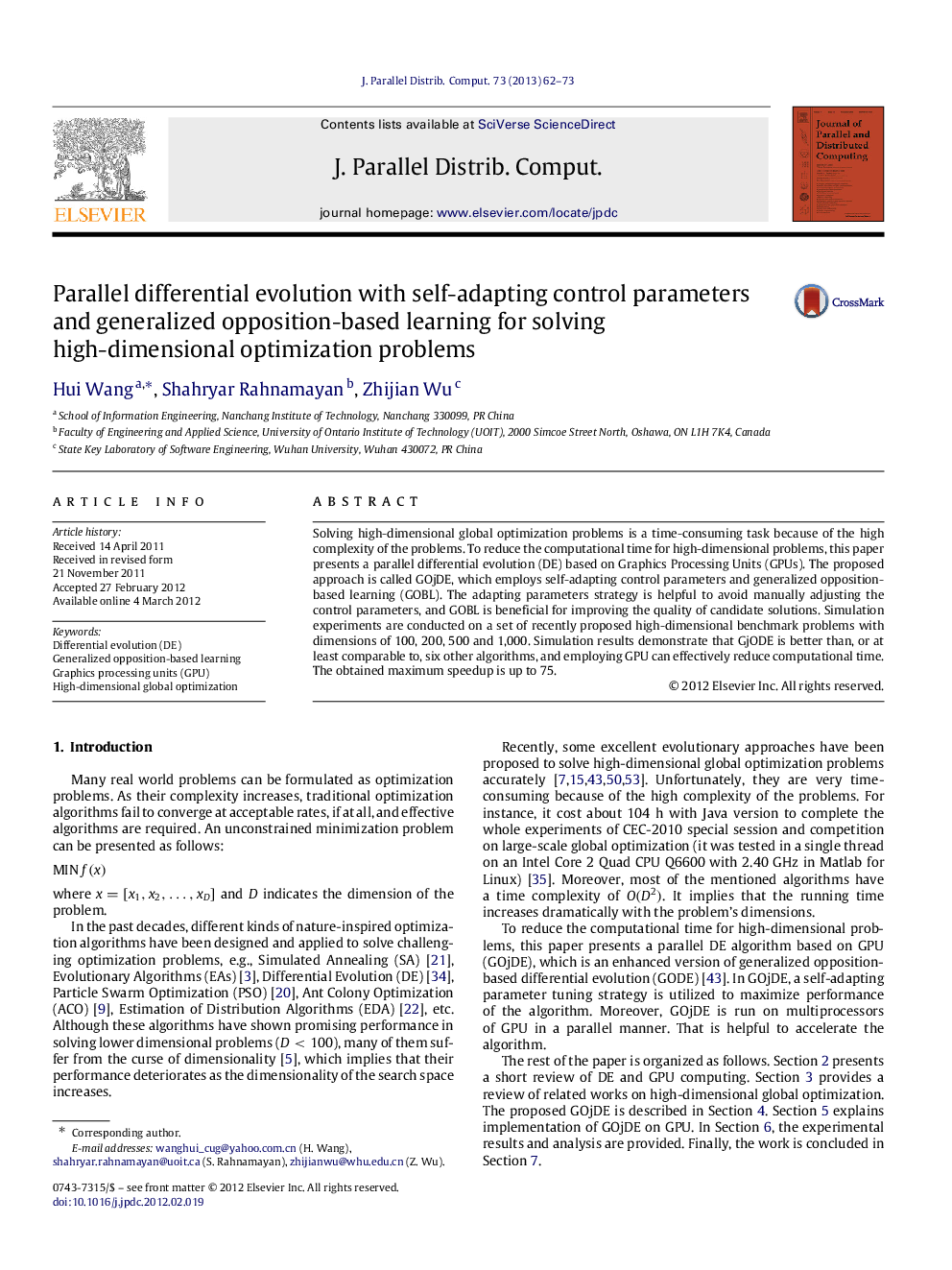
Solving high-dimensional global optimization problems is a time-consuming task because of the high complexity of the problems. To reduce the computational time for high-dimensional problems, this paper presents a parallel differential evolution (DE) based on Graphics Processing Units (GPUs). The proposed approach is called GOjDE, which employs self-adapting control parameters and generalized opposition-based learning (GOBL). The adapting parameters strategy is helpful to avoid manually adjusting the control parameters, and GOBL is beneficial for improving the quality of candidate solutions. Simulation experiments are conducted on a set of recently proposed high-dimensional benchmark problems with dimensions of 100, 200, 500 and 1,000. Simulation results demonstrate that GjODE is better than, or at least comparable to, six other algorithms, and employing GPU can effectively reduce computational time. The obtained maximum speedup is up to 75.
► Solving high-dimensional global optimization problems is a time-consuming task.
► GPU is used to reduce the computational time for solving high-dimensional problems.
► Increasing population size will improve the speedup.
► It is applicable to use GPU to solve higher dimensional optimization problems.
Journal: Journal of Parallel and Distributed Computing - Volume 73, Issue 1, January 2013, Pages 62–73