کد مقاله | کد نشریه | سال انتشار | مقاله انگلیسی | نسخه تمام متن |
---|---|---|---|---|
4464682 | 1621817 | 2015 | 11 صفحه PDF | دانلود رایگان |
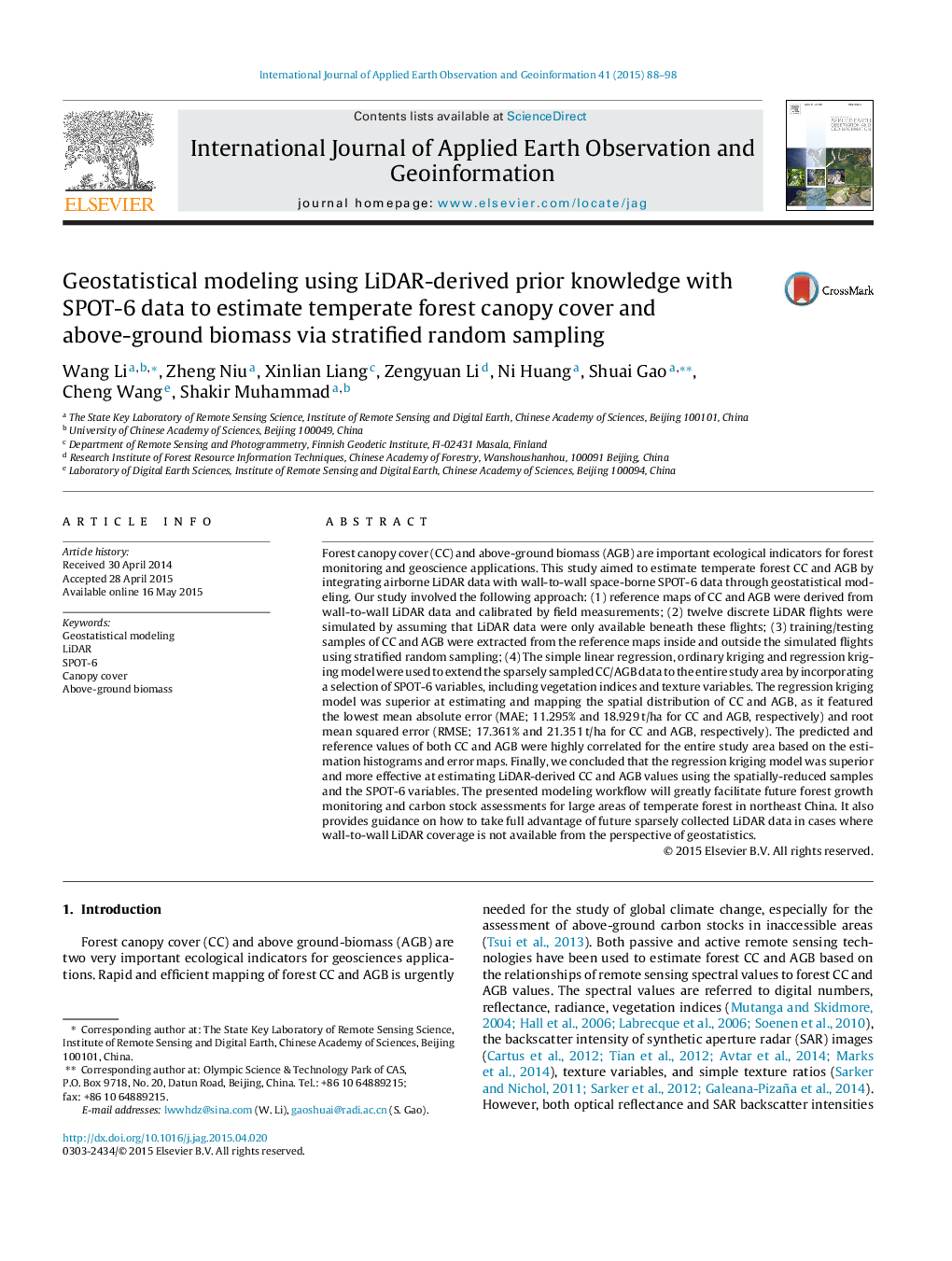
• A stratified random sampling strategy based on LiDAR prior knowledge was proposed.
• Regression kriging model is superior and more effective at estimating LiDAR-derived CC and AGB values using the spatially-reduced samples and the SPOT-6 variables.
• Reliable wall-to-wall products of canopy cover and above-ground biomass can be produced using samples of airborne LiDAR data, calibrated with field data, in conjunction with space-borne SPOT-6 data.
• The presented modeling workflow will greatly facilitate future forest growth monitoring and carbon stock assessments for large areas of temperate forest in northeast China.
Forest canopy cover (CC) and above-ground biomass (AGB) are important ecological indicators for forest monitoring and geoscience applications. This study aimed to estimate temperate forest CC and AGB by integrating airborne LiDAR data with wall-to-wall space-borne SPOT-6 data through geostatistical modeling. Our study involved the following approach: (1) reference maps of CC and AGB were derived from wall-to-wall LiDAR data and calibrated by field measurements; (2) twelve discrete LiDAR flights were simulated by assuming that LiDAR data were only available beneath these flights; (3) training/testing samples of CC and AGB were extracted from the reference maps inside and outside the simulated flights using stratified random sampling; (4) The simple linear regression, ordinary kriging and regression kriging model were used to extend the sparsely sampled CC/AGB data to the entire study area by incorporating a selection of SPOT-6 variables, including vegetation indices and texture variables. The regression kriging model was superior at estimating and mapping the spatial distribution of CC and AGB, as it featured the lowest mean absolute error (MAE; 11.295% and 18.929 t/ha for CC and AGB, respectively) and root mean squared error (RMSE; 17.361% and 21.351 t/ha for CC and AGB, respectively). The predicted and reference values of both CC and AGB were highly correlated for the entire study area based on the estimation histograms and error maps. Finally, we concluded that the regression kriging model was superior and more effective at estimating LiDAR-derived CC and AGB values using the spatially-reduced samples and the SPOT-6 variables. The presented modeling workflow will greatly facilitate future forest growth monitoring and carbon stock assessments for large areas of temperate forest in northeast China. It also provides guidance on how to take full advantage of future sparsely collected LiDAR data in cases where wall-to-wall LiDAR coverage is not available from the perspective of geostatistics.
Journal: International Journal of Applied Earth Observation and Geoinformation - Volume 41, September 2015, Pages 88–98