کد مقاله | کد نشریه | سال انتشار | مقاله انگلیسی | نسخه تمام متن |
---|---|---|---|---|
4464995 | 1621841 | 2012 | 13 صفحه PDF | دانلود رایگان |
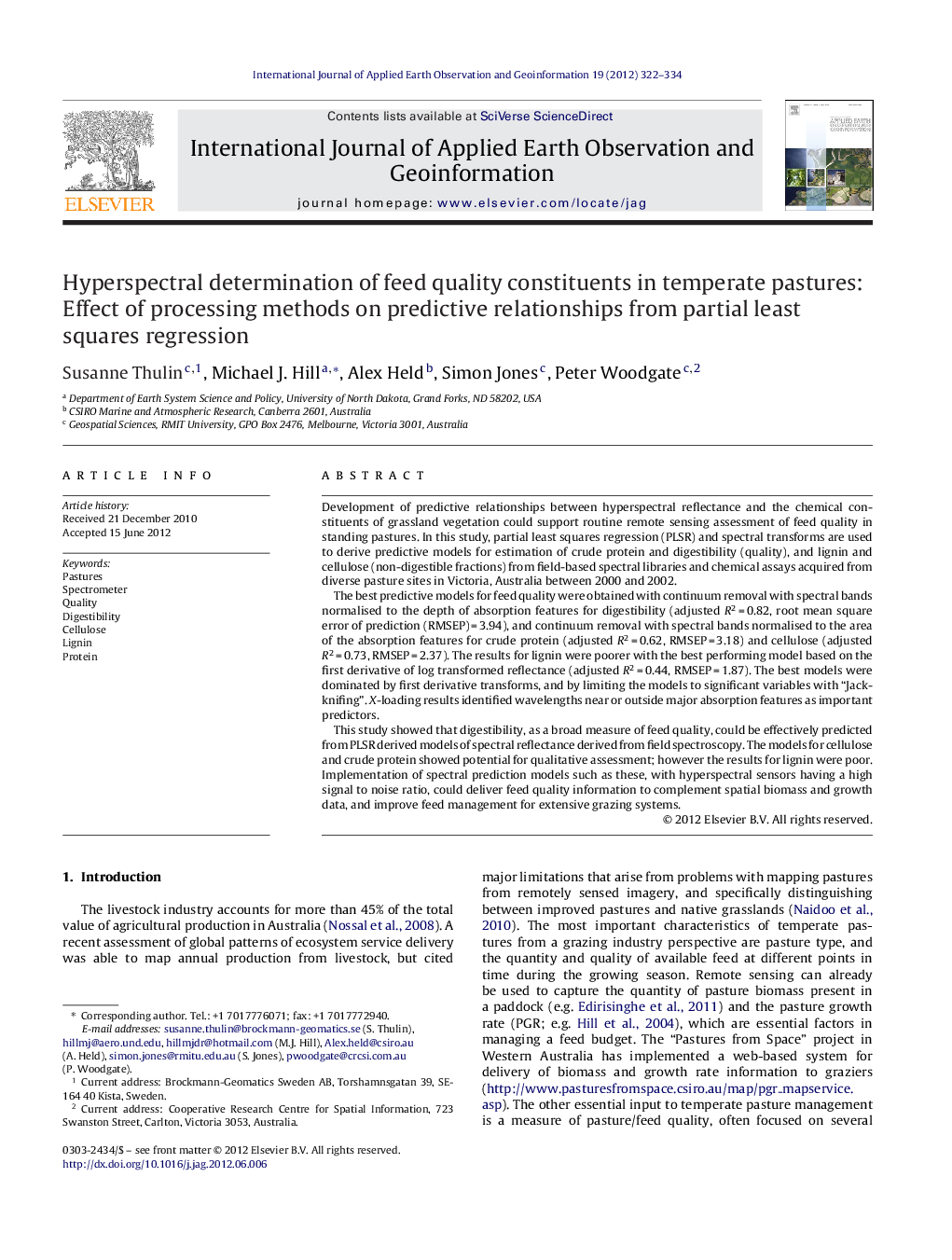
Development of predictive relationships between hyperspectral reflectance and the chemical constituents of grassland vegetation could support routine remote sensing assessment of feed quality in standing pastures. In this study, partial least squares regression (PLSR) and spectral transforms are used to derive predictive models for estimation of crude protein and digestibility (quality), and lignin and cellulose (non-digestible fractions) from field-based spectral libraries and chemical assays acquired from diverse pasture sites in Victoria, Australia between 2000 and 2002.The best predictive models for feed quality were obtained with continuum removal with spectral bands normalised to the depth of absorption features for digestibility (adjusted R2 = 0.82, root mean square error of prediction (RMSEP) = 3.94), and continuum removal with spectral bands normalised to the area of the absorption features for crude protein (adjusted R2 = 0.62, RMSEP = 3.18) and cellulose (adjusted R2 = 0.73, RMSEP = 2.37). The results for lignin were poorer with the best performing model based on the first derivative of log transformed reflectance (adjusted R2 = 0.44, RMSEP = 1.87). The best models were dominated by first derivative transforms, and by limiting the models to significant variables with “Jack-knifing”. X-loading results identified wavelengths near or outside major absorption features as important predictors.This study showed that digestibility, as a broad measure of feed quality, could be effectively predicted from PLSR derived models of spectral reflectance derived from field spectroscopy. The models for cellulose and crude protein showed potential for qualitative assessment; however the results for lignin were poor. Implementation of spectral prediction models such as these, with hyperspectral sensors having a high signal to noise ratio, could deliver feed quality information to complement spatial biomass and growth data, and improve feed management for extensive grazing systems.
► Predictive models are developed from analysis of pasture spectra.
► Smoothing methods and spectral transforms influence wavelength selection.
► Useful predictive relationships obtained for cellulose, crude protein and digestibility.
► Large confidence intervals may be overcome using class ranges or thresholds.
Journal: International Journal of Applied Earth Observation and Geoinformation - Volume 19, October 2012, Pages 322–334