کد مقاله | کد نشریه | سال انتشار | مقاله انگلیسی | نسخه تمام متن |
---|---|---|---|---|
4605681 | 1337592 | 2007 | 22 صفحه PDF | دانلود رایگان |
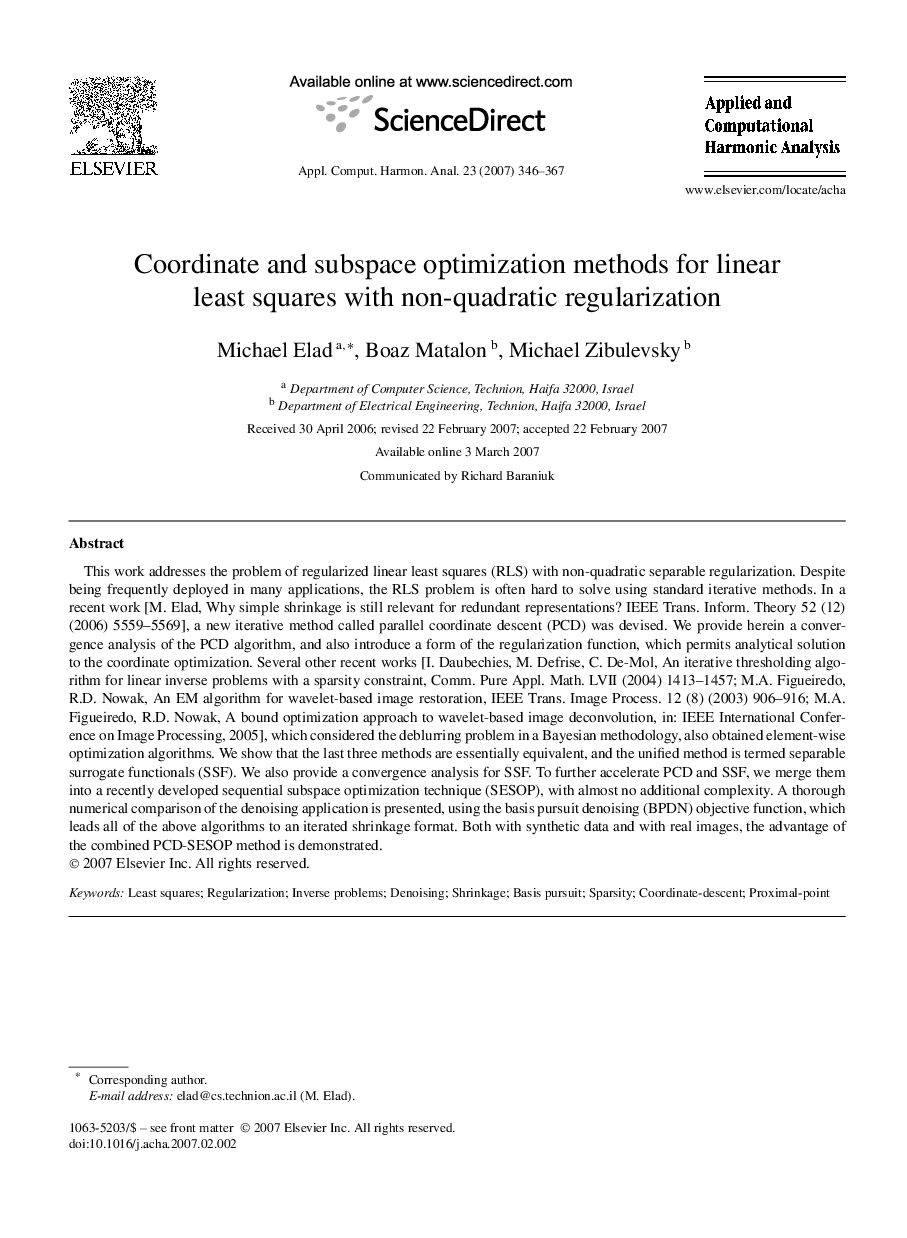
This work addresses the problem of regularized linear least squares (RLS) with non-quadratic separable regularization. Despite being frequently deployed in many applications, the RLS problem is often hard to solve using standard iterative methods. In a recent work [M. Elad, Why simple shrinkage is still relevant for redundant representations? IEEE Trans. Inform. Theory 52 (12) (2006) 5559–5569], a new iterative method called parallel coordinate descent (PCD) was devised. We provide herein a convergence analysis of the PCD algorithm, and also introduce a form of the regularization function, which permits analytical solution to the coordinate optimization. Several other recent works [I. Daubechies, M. Defrise, C. De-Mol, An iterative thresholding algorithm for linear inverse problems with a sparsity constraint, Comm. Pure Appl. Math. LVII (2004) 1413–1457; M.A. Figueiredo, R.D. Nowak, An EM algorithm for wavelet-based image restoration, IEEE Trans. Image Process. 12 (8) (2003) 906–916; M.A. Figueiredo, R.D. Nowak, A bound optimization approach to wavelet-based image deconvolution, in: IEEE International Conference on Image Processing, 2005], which considered the deblurring problem in a Bayesian methodology, also obtained element-wise optimization algorithms. We show that the last three methods are essentially equivalent, and the unified method is termed separable surrogate functionals (SSF). We also provide a convergence analysis for SSF. To further accelerate PCD and SSF, we merge them into a recently developed sequential subspace optimization technique (SESOP), with almost no additional complexity. A thorough numerical comparison of the denoising application is presented, using the basis pursuit denoising (BPDN) objective function, which leads all of the above algorithms to an iterated shrinkage format. Both with synthetic data and with real images, the advantage of the combined PCD-SESOP method is demonstrated.
Journal: Applied and Computational Harmonic Analysis - Volume 23, Issue 3, November 2007, Pages 346-367