کد مقاله | کد نشریه | سال انتشار | مقاله انگلیسی | نسخه تمام متن |
---|---|---|---|---|
4979250 | 1453022 | 2018 | 8 صفحه PDF | دانلود رایگان |
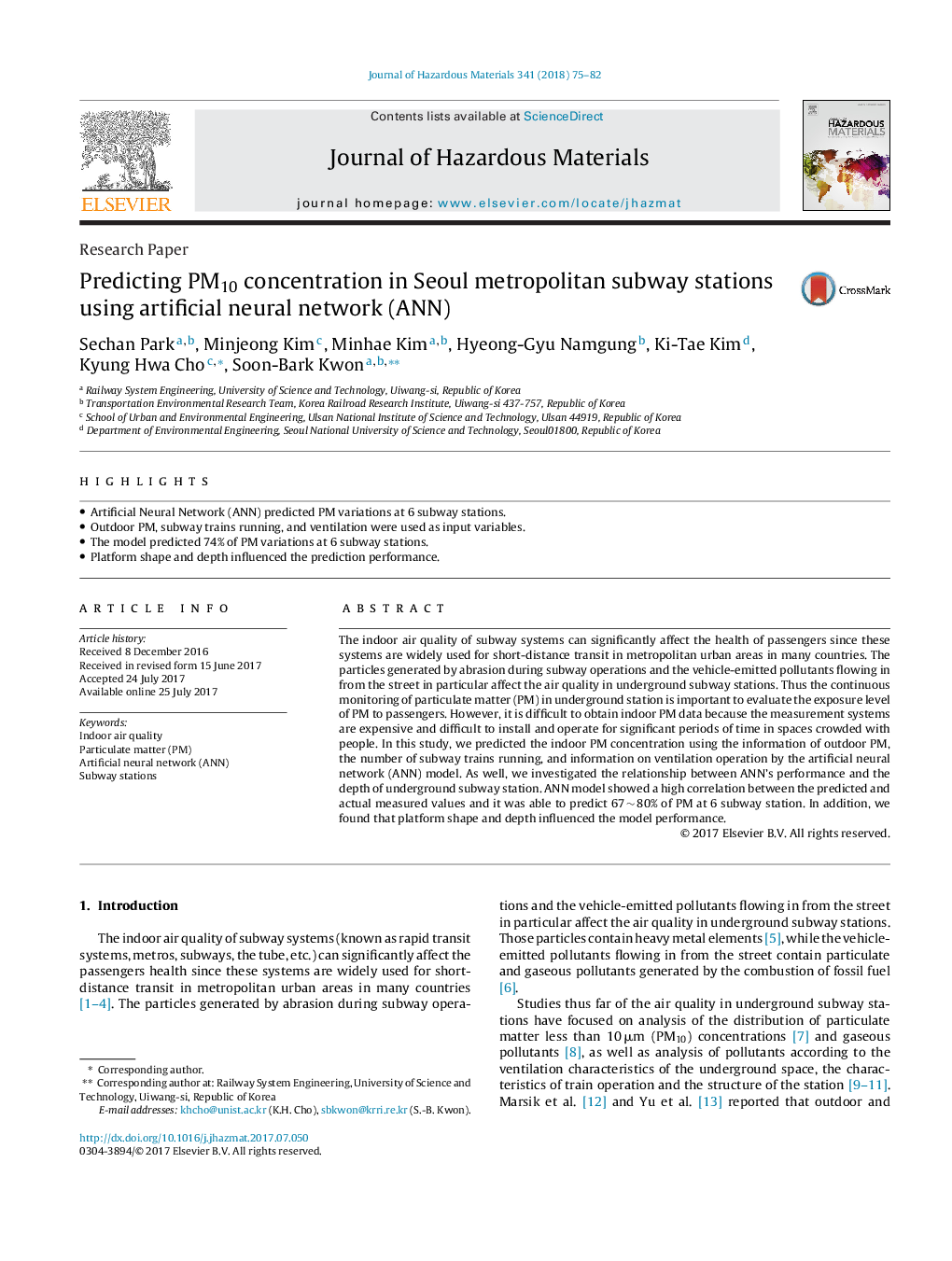
- Artificial Neural Network (ANN) predicted PM variations at 6 subway stations.
- Outdoor PM, subway trains running, and ventilation were used as input variables.
- The model predicted 74% of PM variations at 6 subway stations.
- Platform shape and depth influenced the prediction performance.
The indoor air quality of subway systems can significantly affect the health of passengers since these systems are widely used for short-distance transit in metropolitan urban areas in many countries. The particles generated by abrasion during subway operations and the vehicle-emitted pollutants flowing in from the street in particular affect the air quality in underground subway stations. Thus the continuous monitoring of particulate matter (PM) in underground station is important to evaluate the exposure level of PM to passengers. However, it is difficult to obtain indoor PM data because the measurement systems are expensive and difficult to install and operate for significant periods of time in spaces crowded with people. In this study, we predicted the indoor PM concentration using the information of outdoor PM, the number of subway trains running, and information on ventilation operation by the artificial neural network (ANN) model. As well, we investigated the relationship between ANN's performance and the depth of underground subway station. ANN model showed a high correlation between the predicted and actual measured values and it was able to predict 67 â¼Â 80% of PM at 6 subway station. In addition, we found that platform shape and depth influenced the model performance.
Journal: Journal of Hazardous Materials - Volume 341, 5 January 2018, Pages 75-82