کد مقاله | کد نشریه | سال انتشار | مقاله انگلیسی | نسخه تمام متن |
---|---|---|---|---|
5042217 | 1474376 | 2017 | 10 صفحه PDF | دانلود رایگان |
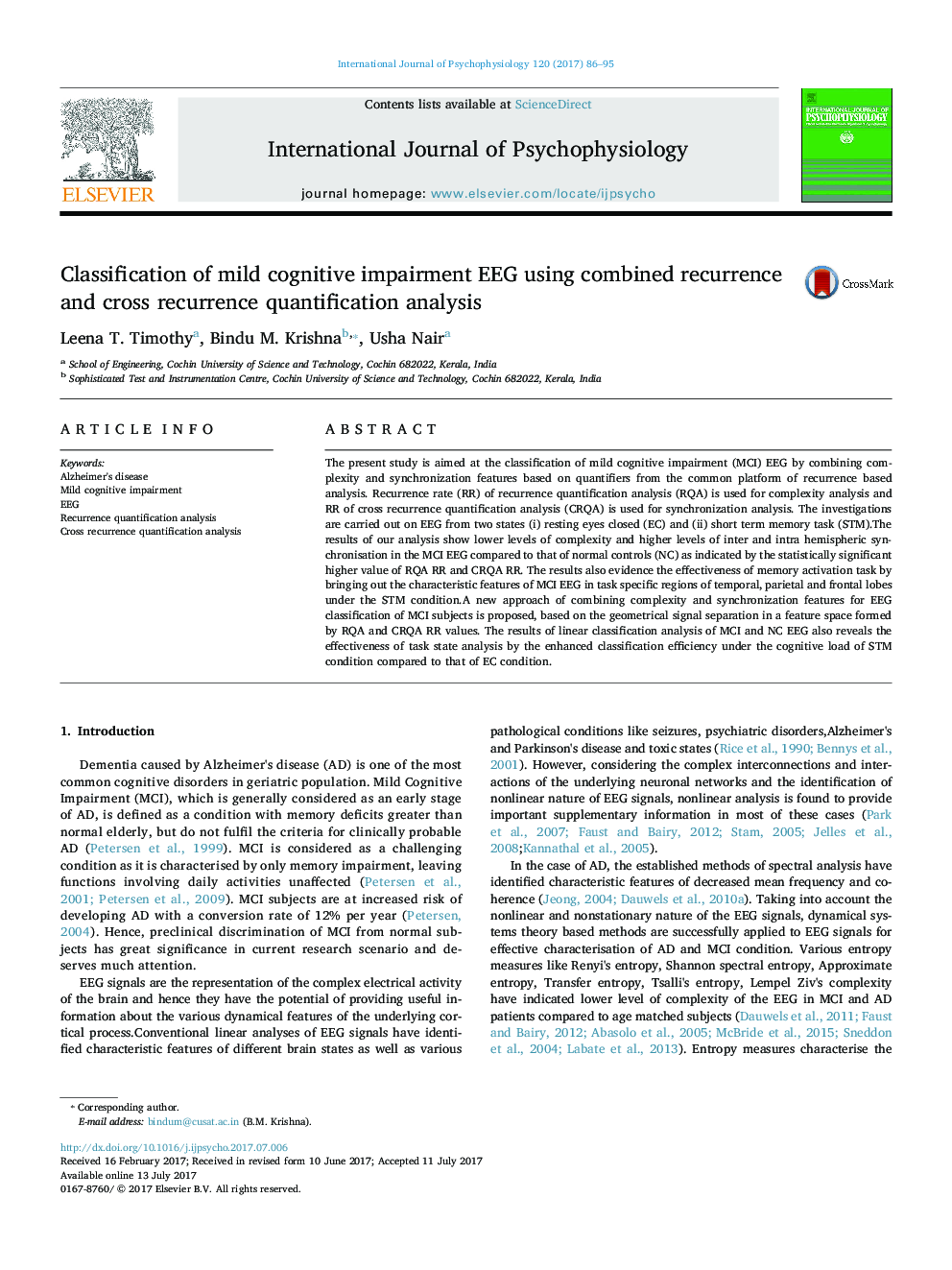
- A new approach of using complexity and synchronization features jointly for the classification of MCI EEG is proposed.
- Lowered complexity and increased synchronization are observed in EEG of MCI compared to normal controls (NC).
- RQA and CRQA measures are combined to form a feature space for geometrical separation of MCI and NC EEG.
- MCI group is differentiated from NC group using support vector machine (SVM) in the proposed feature space.
- A better classification is obtained under short term memory task condition (STM) compared to eyes closed (EC) condition.
The present study is aimed at the classification of mild cognitive impairment (MCI) EEG by combining complexity and synchronization features based on quantifiers from the common platform of recurrence based analysis. Recurrence rate (RR) of recurrence quantification analysis (RQA) is used for complexity analysis and RR of cross recurrence quantification analysis (CRQA) is used for synchronization analysis. The investigations are carried out on EEG from two states (i) resting eyes closed (EC) and (ii) short term memory task (STM).The results of our analysis show lower levels of complexity and higher levels of inter and intra hemispheric synchronisation in the MCI EEG compared to that of normal controls (NC) as indicated by the statistically significant higher value of RQA RR and CRQA RR. The results also evidence the effectiveness of memory activation task by bringing out the characteristic features of MCI EEG in task specific regions of temporal, parietal and frontal lobes under the STM condition.A new approach of combining complexity and synchronization features for EEG classification of MCI subjects is proposed, based on the geometrical signal separation in a feature space formed by RQA and CRQA RR values. The results of linear classification analysis of MCI and NC EEG also reveals the effectiveness of task state analysis by the enhanced classification efficiency under the cognitive load of STM condition compared to that of EC condition.
Journal: International Journal of Psychophysiology - Volume 120, October 2017, Pages 86-95