کد مقاله | کد نشریه | سال انتشار | مقاله انگلیسی | نسخه تمام متن |
---|---|---|---|---|
5095745 | 1376482 | 2016 | 17 صفحه PDF | دانلود رایگان |
عنوان انگلیسی مقاله ISI
â1-regularization of high-dimensional time-series models with non-Gaussian and heteroskedastic errors
دانلود مقاله + سفارش ترجمه
دانلود مقاله ISI انگلیسی
رایگان برای ایرانیان
کلمات کلیدی
موضوعات مرتبط
مهندسی و علوم پایه
ریاضیات
آمار و احتمال
پیش نمایش صفحه اول مقاله
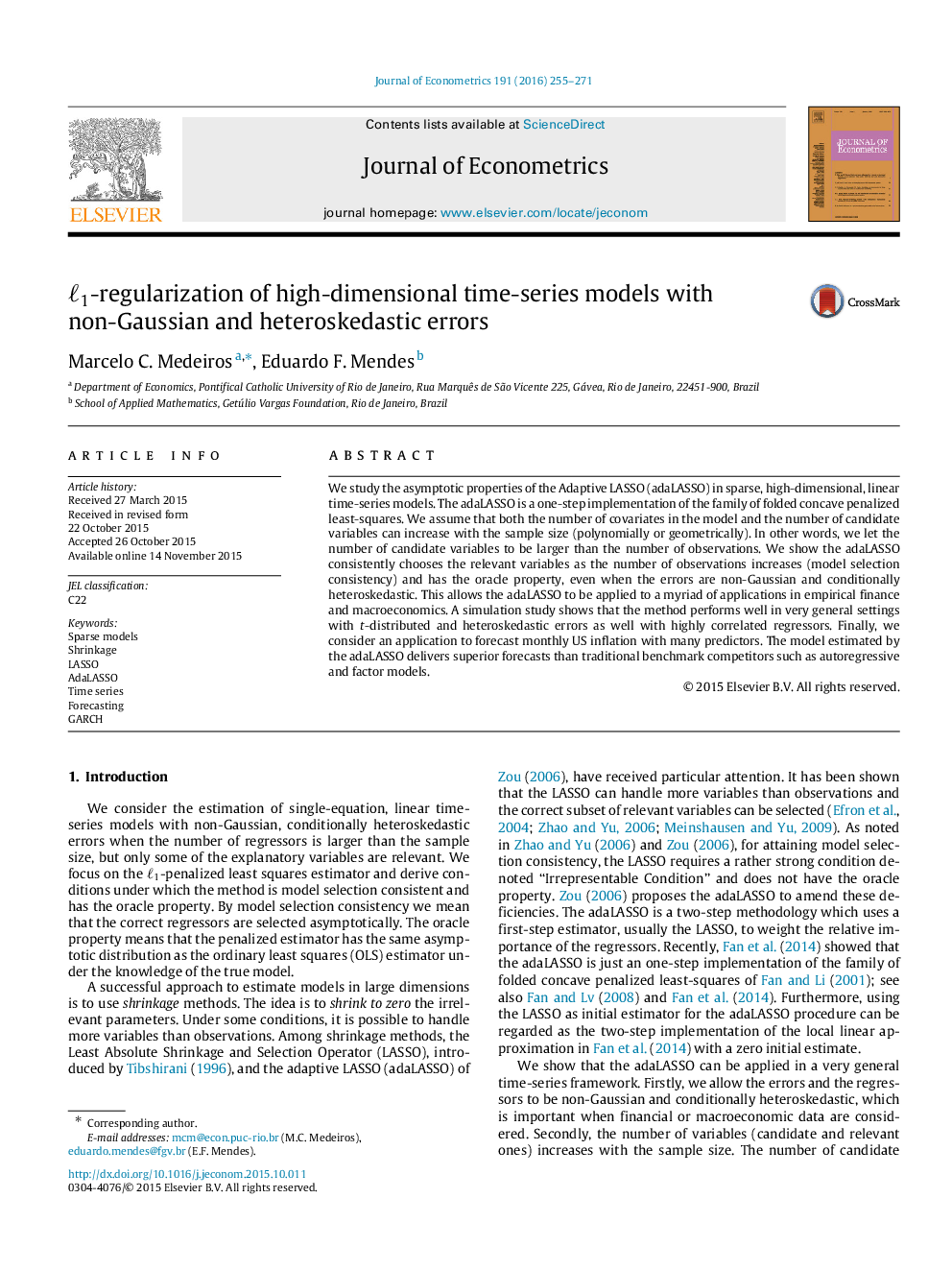
چکیده انگلیسی
We study the asymptotic properties of the Adaptive LASSO (adaLASSO) in sparse, high-dimensional, linear time-series models. The adaLASSO is a one-step implementation of the family of folded concave penalized least-squares. We assume that both the number of covariates in the model and the number of candidate variables can increase with the sample size (polynomially or geometrically). In other words, we let the number of candidate variables to be larger than the number of observations. We show the adaLASSO consistently chooses the relevant variables as the number of observations increases (model selection consistency) and has the oracle property, even when the errors are non-Gaussian and conditionally heteroskedastic. This allows the adaLASSO to be applied to a myriad of applications in empirical finance and macroeconomics. A simulation study shows that the method performs well in very general settings with t-distributed and heteroskedastic errors as well with highly correlated regressors. Finally, we consider an application to forecast monthly US inflation with many predictors. The model estimated by the adaLASSO delivers superior forecasts than traditional benchmark competitors such as autoregressive and factor models.
ناشر
Database: Elsevier - ScienceDirect (ساینس دایرکت)
Journal: Journal of Econometrics - Volume 191, Issue 1, March 2016, Pages 255-271
Journal: Journal of Econometrics - Volume 191, Issue 1, March 2016, Pages 255-271
نویسندگان
Marcelo C. Medeiros, Eduardo F. Mendes,