کد مقاله | کد نشریه | سال انتشار | مقاله انگلیسی | نسخه تمام متن |
---|---|---|---|---|
5116655 | 1485220 | 2017 | 9 صفحه PDF | دانلود رایگان |
عنوان انگلیسی مقاله ISI
Application of decomposition-ensemble learning paradigm with phase space reconstruction for day-ahead PM2.5 concentration forecasting
دانلود مقاله + سفارش ترجمه
دانلود مقاله ISI انگلیسی
رایگان برای ایرانیان
کلمات کلیدی
موضوعات مرتبط
مهندسی و علوم پایه
مهندسی انرژی
انرژی های تجدید پذیر، توسعه پایدار و محیط زیست
پیش نمایش صفحه اول مقاله
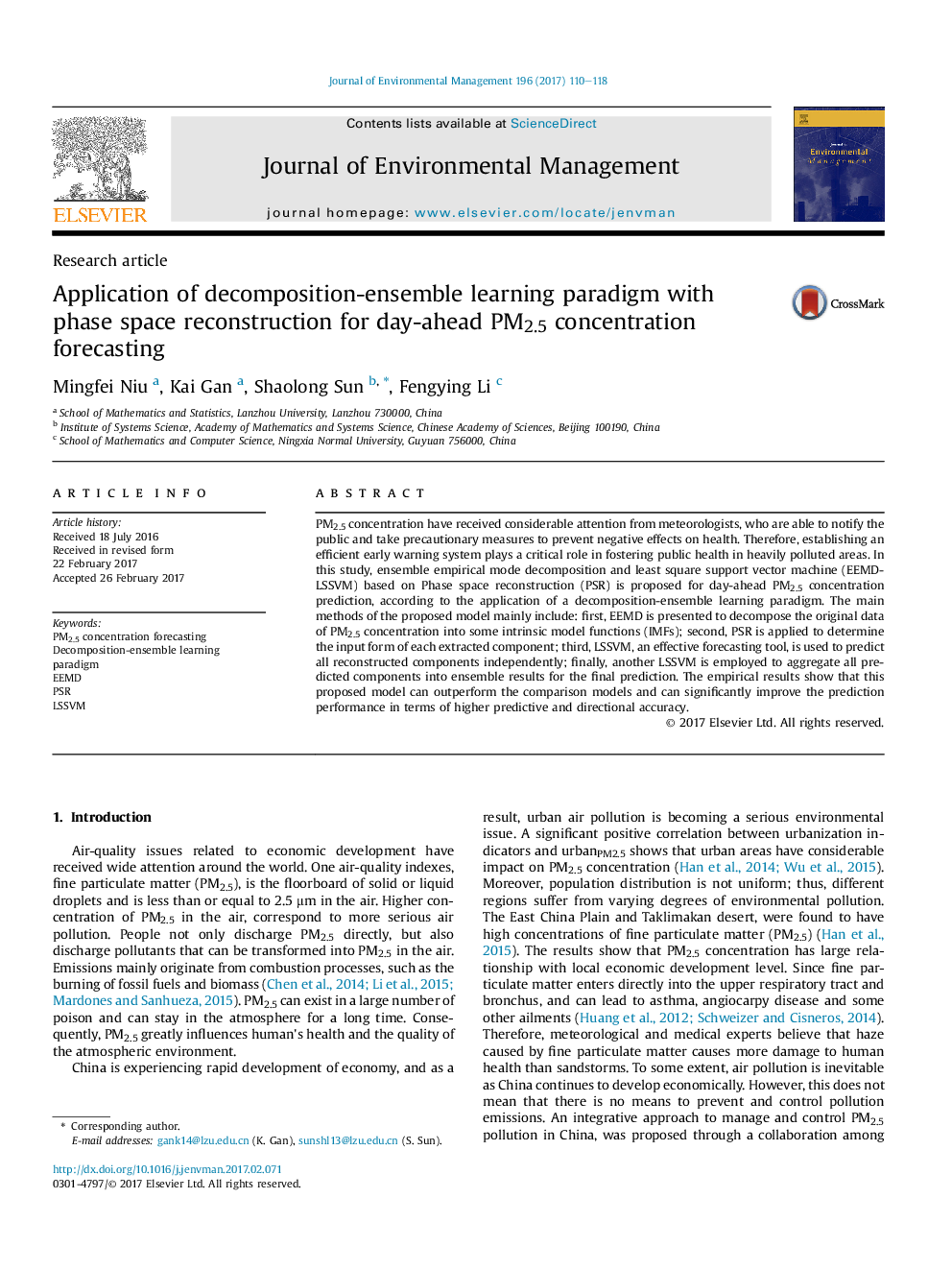
چکیده انگلیسی
PM2.5 concentration have received considerable attention from meteorologists, who are able to notify the public and take precautionary measures to prevent negative effects on health. Therefore, establishing an efficient early warning system plays a critical role in fostering public health in heavily polluted areas. In this study, ensemble empirical mode decomposition and least square support vector machine (EEMD-LSSVM) based on Phase space reconstruction (PSR) is proposed for day-ahead PM2.5 concentration prediction, according to the application of a decomposition-ensemble learning paradigm. The main methods of the proposed model mainly include: first, EEMD is presented to decompose the original data of PM2.5 concentration into some intrinsic model functions (IMFs); second, PSR is applied to determine the input form of each extracted component; third, LSSVM, an effective forecasting tool, is used to predict all reconstructed components independently; finally, another LSSVM is employed to aggregate all predicted components into ensemble results for the final prediction. The empirical results show that this proposed model can outperform the comparison models and can significantly improve the prediction performance in terms of higher predictive and directional accuracy.
ناشر
Database: Elsevier - ScienceDirect (ساینس دایرکت)
Journal: Journal of Environmental Management - Volume 196, 1 July 2017, Pages 110-118
Journal: Journal of Environmental Management - Volume 196, 1 July 2017, Pages 110-118
نویسندگان
Mingfei Niu, Kai Gan, Shaolong Sun, Fengying Li,