کد مقاله | کد نشریه | سال انتشار | مقاله انگلیسی | نسخه تمام متن |
---|---|---|---|---|
5131345 | 1490881 | 2017 | 14 صفحه PDF | دانلود رایگان |
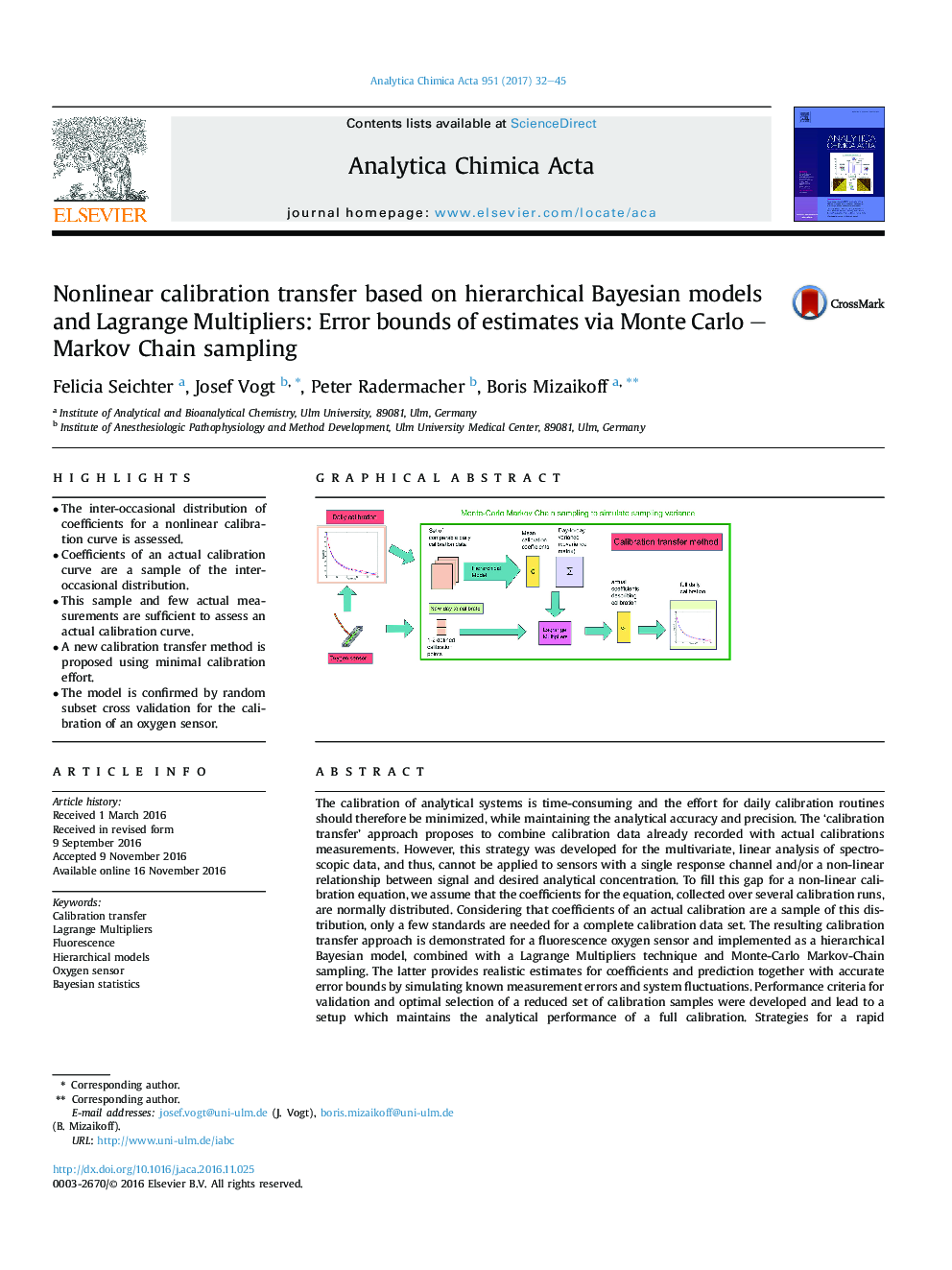
• The inter-occasional distribution of coefficients for a nonlinear calibration curve is assessed.
• Coefficients of an actual calibration curve are a sample of the inter-occasional distribution.
• This sample and few actual measurements are sufficient to assess an actual calibration curve.
• A new calibration transfer method is proposed using minimal calibration effort.
• The model is confirmed by random subset cross validation for the calibration of an oxygen sensor.
The calibration of analytical systems is time-consuming and the effort for daily calibration routines should therefore be minimized, while maintaining the analytical accuracy and precision. The ‘calibration transfer’ approach proposes to combine calibration data already recorded with actual calibrations measurements. However, this strategy was developed for the multivariate, linear analysis of spectroscopic data, and thus, cannot be applied to sensors with a single response channel and/or a non-linear relationship between signal and desired analytical concentration. To fill this gap for a non-linear calibration equation, we assume that the coefficients for the equation, collected over several calibration runs, are normally distributed. Considering that coefficients of an actual calibration are a sample of this distribution, only a few standards are needed for a complete calibration data set. The resulting calibration transfer approach is demonstrated for a fluorescence oxygen sensor and implemented as a hierarchical Bayesian model, combined with a Lagrange Multipliers technique and Monte-Carlo Markov-Chain sampling. The latter provides realistic estimates for coefficients and prediction together with accurate error bounds by simulating known measurement errors and system fluctuations. Performance criteria for validation and optimal selection of a reduced set of calibration samples were developed and lead to a setup which maintains the analytical performance of a full calibration. Strategies for a rapid determination of problems occurring in a daily calibration routine, are proposed, thereby opening the possibility of correcting the problem just in time.
Figure optionsDownload high-quality image (305 K)Download as PowerPoint slide
Journal: Analytica Chimica Acta - Volume 951, 25 January 2017, Pages 32–45