کد مقاله | کد نشریه | سال انتشار | مقاله انگلیسی | نسخه تمام متن |
---|---|---|---|---|
5132153 | 1491509 | 2017 | 12 صفحه PDF | دانلود رایگان |
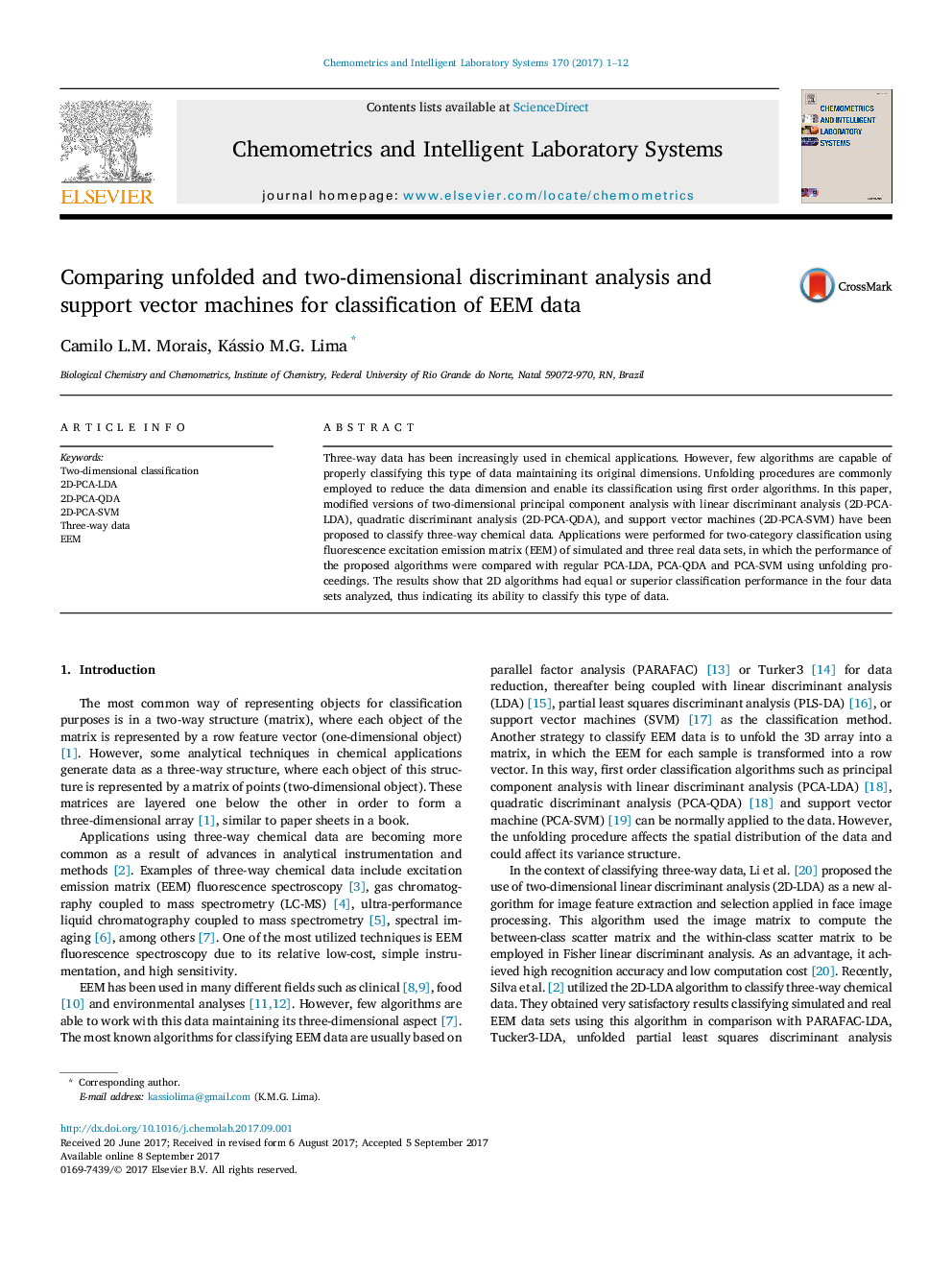
- Modified versions of 2D-PCA-LDA, 2D-PCA-QDA and 2D-PCA-SVM have been proposed.
- Applications were performed for two-category classification using fluorescence EEM.
- The results show that 2D algorithms had equal or superior classification performance.
Three-way data has been increasingly used in chemical applications. However, few algorithms are capable of properly classifying this type of data maintaining its original dimensions. Unfolding procedures are commonly employed to reduce the data dimension and enable its classification using first order algorithms. In this paper, modified versions of two-dimensional principal component analysis with linear discriminant analysis (2D-PCA-LDA), quadratic discriminant analysis (2D-PCA-QDA), and support vector machines (2D-PCA-SVM) have been proposed to classify three-way chemical data. Applications were performed for two-category classification using fluorescence excitation emission matrix (EEM) of simulated and three real data sets, in which the performance of the proposed algorithms were compared with regular PCA-LDA, PCA-QDA and PCA-SVM using unfolding proceedings. The results show that 2D algorithms had equal or superior classification performance in the four data sets analyzed, thus indicating its ability to classify this type of data.
198
Journal: Chemometrics and Intelligent Laboratory Systems - Volume 170, 15 November 2017, Pages 1-12