کد مقاله | کد نشریه | سال انتشار | مقاله انگلیسی | نسخه تمام متن |
---|---|---|---|---|
5132186 | 1491512 | 2017 | 6 صفحه PDF | دانلود رایگان |
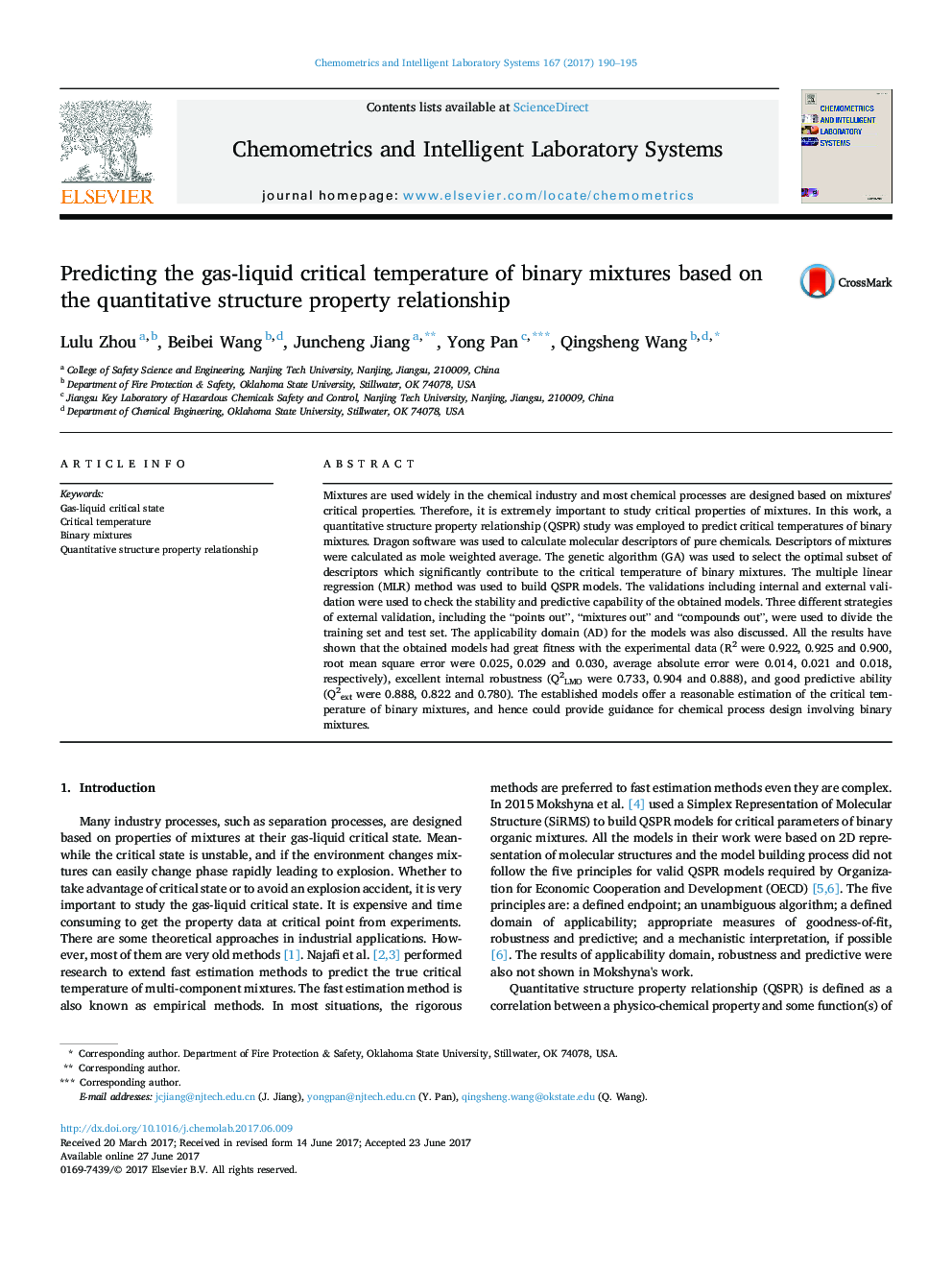
- Quantitative structure-property relationship (QSPR) models for mixture property prediction were built successfully.
- The multiple linear regression (MLR) method was used to build QSPR models.
- Three different strategies of external validation were used to divide the training set and test set.
- The models can estimate critical temperatures of binary mixtures correctly.
Mixtures are used widely in the chemical industry and most chemical processes are designed based on mixtures' critical properties. Therefore, it is extremely important to study critical properties of mixtures. In this work, a quantitative structure property relationship (QSPR) study was employed to predict critical temperatures of binary mixtures. Dragon software was used to calculate molecular descriptors of pure chemicals. Descriptors of mixtures were calculated as mole weighted average. The genetic algorithm (GA) was used to select the optimal subset of descriptors which significantly contribute to the critical temperature of binary mixtures. The multiple linear regression (MLR) method was used to build QSPR models. The validations including internal and external validation were used to check the stability and predictive capability of the obtained models. Three different strategies of external validation, including the “points out”, “mixtures out” and “compounds out”, were used to divide the training set and test set. The applicability domain (AD) for the models was also discussed. All the results have shown that the obtained models had great fitness with the experimental data (R2 were 0.922, 0.925 and 0.900, root mean square error were 0.025, 0.029 and 0.030, average absolute error were 0.014, 0.021 and 0.018, respectively), excellent internal robustness (Q2LMO were 0.733, 0.904 and 0.888), and good predictive ability (Q2ext were 0.888, 0.822 and 0.780). The established models offer a reasonable estimation of the critical temperature of binary mixtures, and hence could provide guidance for chemical process design involving binary mixtures.
Journal: Chemometrics and Intelligent Laboratory Systems - Volume 167, 15 August 2017, Pages 190-195