کد مقاله | کد نشریه | سال انتشار | مقاله انگلیسی | نسخه تمام متن |
---|---|---|---|---|
5132245 | 1491517 | 2017 | 14 صفحه PDF | دانلود رایگان |
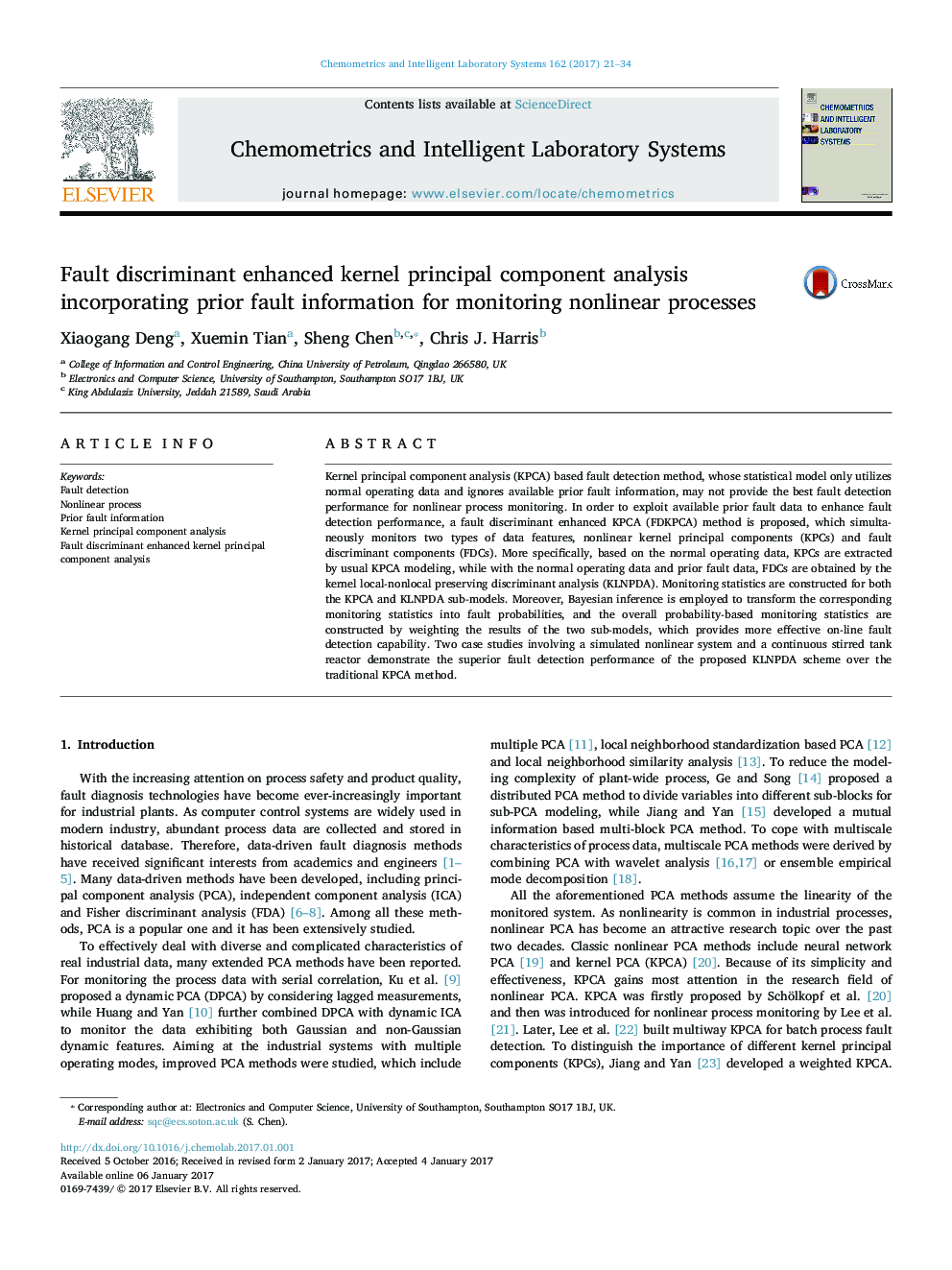
- Propose a fault discriminant enhanced kernel principal component analysis (KPCA) method for monitoring nonlinear processes.
- Extract a KPCA sub-model from the normal operating data.
- Built a kernel local and nonlocal preserving discriminant analysis sub-model from both the normal and prior fault data.
- Derive the probability-based monitoring statistics by weighting the two sub-models with Bayesian inference.
Kernel principal component analysis (KPCA) based fault detection method, whose statistical model only utilizes normal operating data and ignores available prior fault information, may not provide the best fault detection performance for nonlinear process monitoring. In order to exploit available prior fault data to enhance fault detection performance, a fault discriminant enhanced KPCA (FDKPCA) method is proposed, which simultaneously monitors two types of data features, nonlinear kernel principal components (KPCs) and fault discriminant components (FDCs). More specifically, based on the normal operating data, KPCs are extracted by usual KPCA modeling, while with the normal operating data and prior fault data, FDCs are obtained by the kernel local-nonlocal preserving discriminant analysis (KLNPDA). Monitoring statistics are constructed for both the KPCA and KLNPDA sub-models. Moreover, Bayesian inference is employed to transform the corresponding monitoring statistics into fault probabilities, and the overall probability-based monitoring statistics are constructed by weighting the results of the two sub-models, which provides more effective on-line fault detection capability. Two case studies involving a simulated nonlinear system and a continuous stirred tank reactor demonstrate the superior fault detection performance of the proposed KLNPDA scheme over the traditional KPCA method.
Journal: Chemometrics and Intelligent Laboratory Systems - Volume 162, 15 March 2017, Pages 21-34