کد مقاله | کد نشریه | سال انتشار | مقاله انگلیسی | نسخه تمام متن |
---|---|---|---|---|
5132249 | 1491517 | 2017 | 8 صفحه PDF | دانلود رایگان |
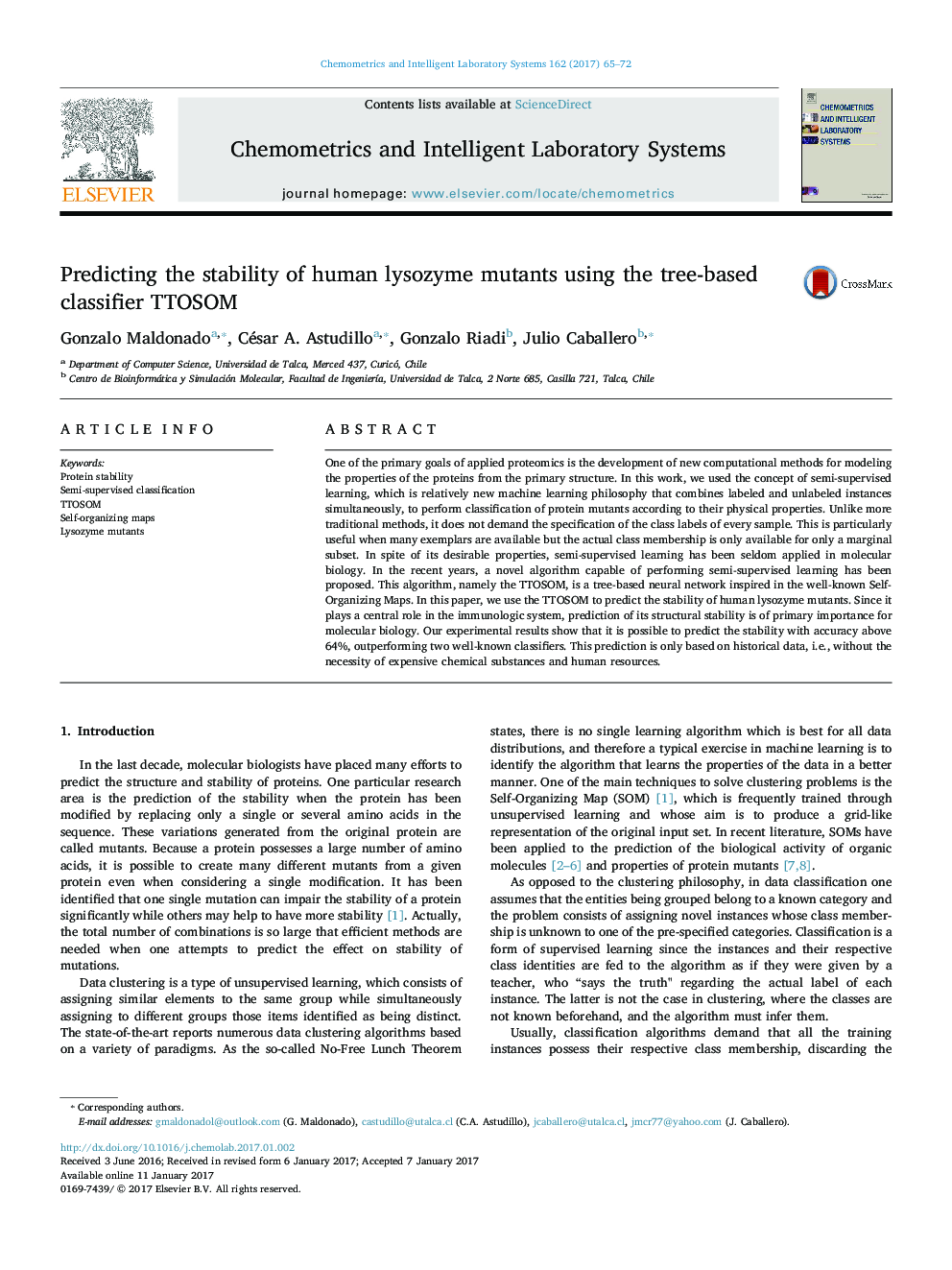
- We present a semi-supervised paradigm for predicting stability of protein sequences.
- The study focuses in the case when only few cases possess known stability.
- We successfully predict the stability of the mutants of the human lysozyme.
- Our experiments show that it is possible to obtain accuracies above the 64%.
- We predict the stability of lysozyme mutants using few labeled instances.
One of the primary goals of applied proteomics is the development of new computational methods for modeling the properties of the proteins from the primary structure. In this work, we used the concept of semi-supervised learning, which is relatively new machine learning philosophy that combines labeled and unlabeled instances simultaneously, to perform classification of protein mutants according to their physical properties. Unlike more traditional methods, it does not demand the specification of the class labels of every sample. This is particularly useful when many exemplars are available but the actual class membership is only available for only a marginal subset. In spite of its desirable properties, semi-supervised learning has been seldom applied in molecular biology. In the recent years, a novel algorithm capable of performing semi-supervised learning has been proposed. This algorithm, namely the TTOSOM, is a tree-based neural network inspired in the well-known Self-Organizing Maps. In this paper, we use the TTOSOM to predict the stability of human lysozyme mutants. Since it plays a central role in the immunologic system, prediction of its structural stability is of primary importance for molecular biology. Our experimental results show that it is possible to predict the stability with accuracy above 64%, outperforming two well-known classifiers. This prediction is only based on historical data, i.e., without the necessity of expensive chemical substances and human resources.
Journal: Chemometrics and Intelligent Laboratory Systems - Volume 162, 15 March 2017, Pages 65-72