کد مقاله | کد نشریه | سال انتشار | مقاله انگلیسی | نسخه تمام متن |
---|---|---|---|---|
5132292 | 1491510 | 2017 | 10 صفحه PDF | دانلود رایگان |
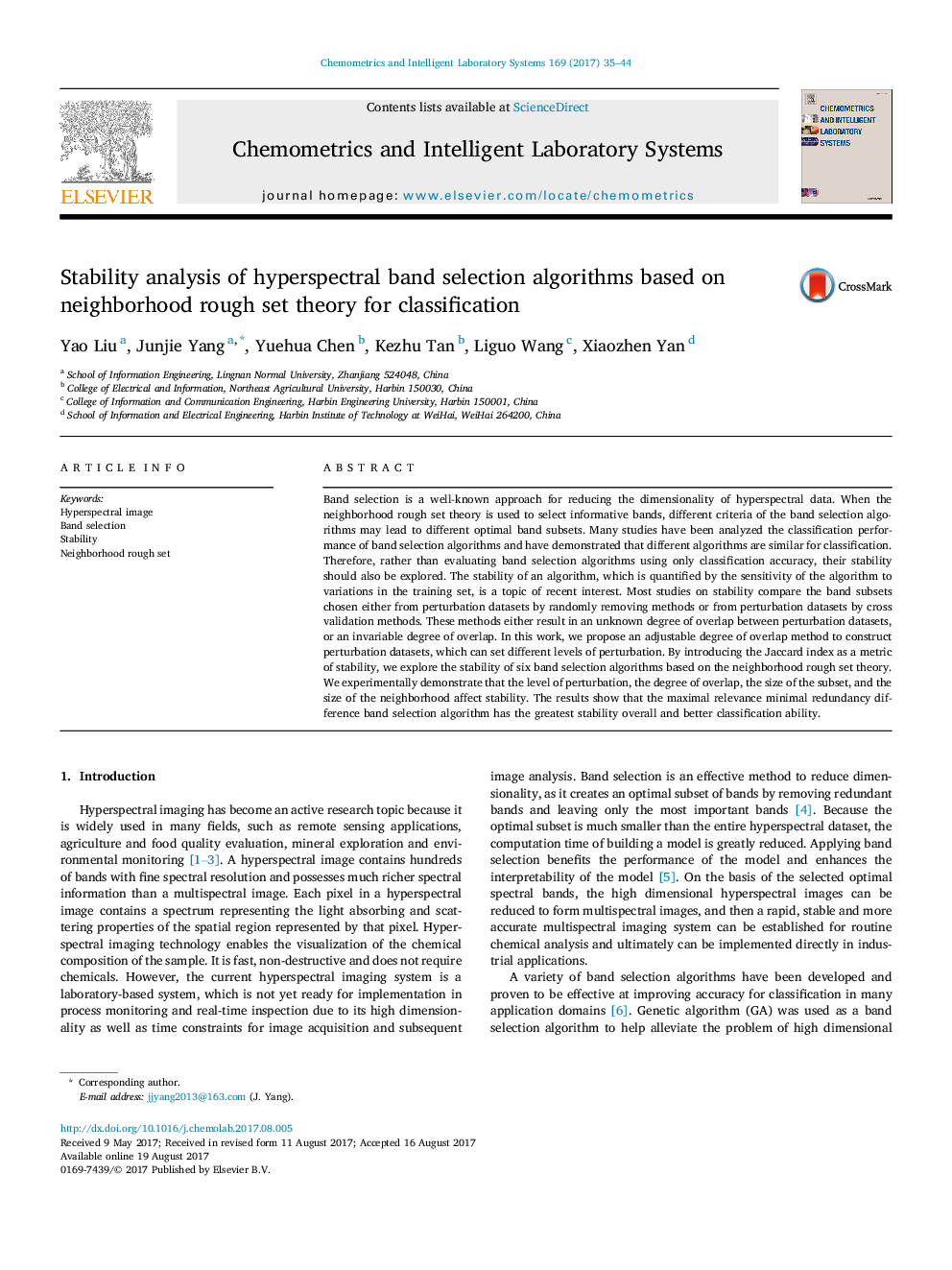
- Propose an adjustable overlap method to construct perturbation dataset.
- Explore the stability of band selection algorithms based on the NRS theory.
- Demonstrate influence factors of stability by introducing Jaccard index as metric.
- The algorithm was applied to soybeans' hyperspectral data between 400 and 1000Â nm.
- MRMRD algorithm has the greatest stability and a better classification ability.
Band selection is a well-known approach for reducing the dimensionality of hyperspectral data. When the neighborhood rough set theory is used to select informative bands, different criteria of the band selection algorithms may lead to different optimal band subsets. Many studies have been analyzed the classification performance of band selection algorithms and have demonstrated that different algorithms are similar for classification. Therefore, rather than evaluating band selection algorithms using only classification accuracy, their stability should also be explored. The stability of an algorithm, which is quantified by the sensitivity of the algorithm to variations in the training set, is a topic of recent interest. Most studies on stability compare the band subsets chosen either from perturbation datasets by randomly removing methods or from perturbation datasets by cross validation methods. These methods either result in an unknown degree of overlap between perturbation datasets, or an invariable degree of overlap. In this work, we propose an adjustable degree of overlap method to construct perturbation datasets, which can set different levels of perturbation. By introducing the Jaccard index as a metric of stability, we explore the stability of six band selection algorithms based on the neighborhood rough set theory. We experimentally demonstrate that the level of perturbation, the degree of overlap, the size of the subset, and the size of the neighborhood affect stability. The results show that the maximal relevance minimal redundancy difference band selection algorithm has the greatest stability overall and better classification ability.
Journal: Chemometrics and Intelligent Laboratory Systems - Volume 169, 15 October 2017, Pages 35-44