کد مقاله | کد نشریه | سال انتشار | مقاله انگلیسی | نسخه تمام متن |
---|---|---|---|---|
5409076 | 1506535 | 2017 | 33 صفحه PDF | دانلود رایگان |
عنوان انگلیسی مقاله ISI
Modeling gas/vapor viscosity of hydrocarbon fluids using a hybrid GMDH-type neural network system
دانلود مقاله + سفارش ترجمه
دانلود مقاله ISI انگلیسی
رایگان برای ایرانیان
کلمات کلیدی
موضوعات مرتبط
مهندسی و علوم پایه
شیمی
شیمی تئوریک و عملی
پیش نمایش صفحه اول مقاله
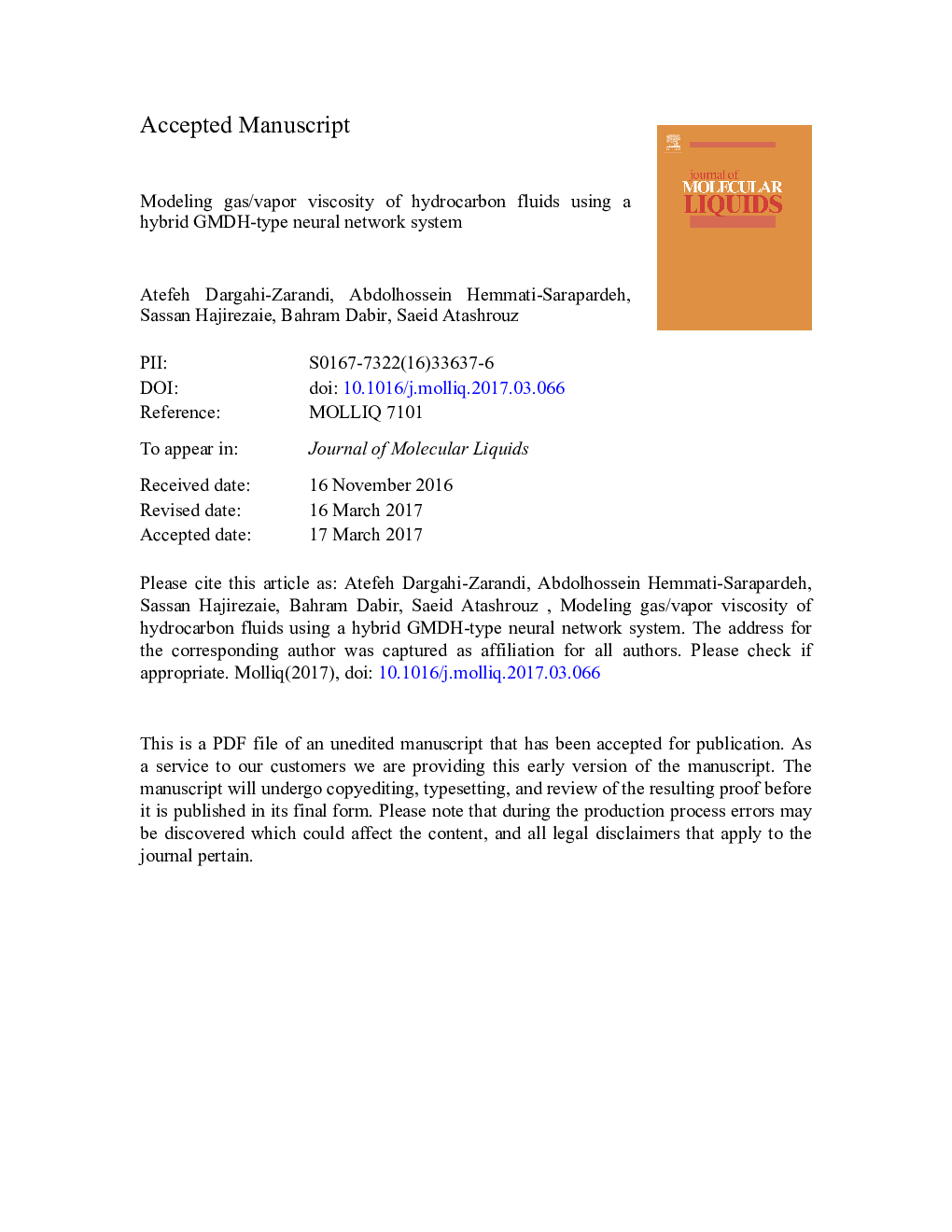
چکیده انگلیسی
Estimation of natural gas viscosity is essential for accurate analysis of gas reserves, reservoir simulation and optimum gas consumption. The general method for calculation of gas viscosity is to conduct experimental tests, however, these tests are normally time consuming and expensive as well as sometimes even impossible at some specific pressure and temperature conditions. In this study, a group method of data handling (GMDH)-type neural network was utilized to predict the viscosity of pure hydrocarbon and gas mixtures containing heavy components and impurities such as carbon dioxide, helium and nitrogen by using more than 3800 data points. First, the data sets were divided into three parts based on their density. Next, a model was developed for each part as a function of molecular weight, pseudo reduced temperature, pseudo reduced pressure and density. The proposed models were compared to 13 well-known models by performing graphical and statistical error analyses. The results indicate that the average absolute percent relative error of the proposed GMDH models is 3.45% and the standard deviation from experimental data is 0.0566. Error distribution curves indicate that the proposed models are able to accurately estimate natural gas viscosity over a wide range of pressure and temperature conditions as no significant error trend is observed in the predictions. Finally, the results of relevancy factor analysis showed that the mixture density has the largest impact on viscosity compared to the other input parameters.
ناشر
Database: Elsevier - ScienceDirect (ساینس دایرکت)
Journal: Journal of Molecular Liquids - Volume 236, June 2017, Pages 162-171
Journal: Journal of Molecular Liquids - Volume 236, June 2017, Pages 162-171
نویسندگان
Atefeh Dargahi-Zarandi, Abdolhossein Hemmati-Sarapardeh, Sassan Hajirezaie, Bahram Dabir, Saeid Atashrouz,