کد مقاله | کد نشریه | سال انتشار | مقاله انگلیسی | نسخه تمام متن |
---|---|---|---|---|
5471213 | 1519388 | 2017 | 15 صفحه PDF | دانلود رایگان |
عنوان انگلیسی مقاله ISI
Online identification of Takagi-Sugeno fuzzy models based on self-adaptive hierarchical particle swarm optimization algorithm
دانلود مقاله + سفارش ترجمه
دانلود مقاله ISI انگلیسی
رایگان برای ایرانیان
کلمات کلیدی
موضوعات مرتبط
مهندسی و علوم پایه
سایر رشته های مهندسی
مکانیک محاسباتی
پیش نمایش صفحه اول مقاله
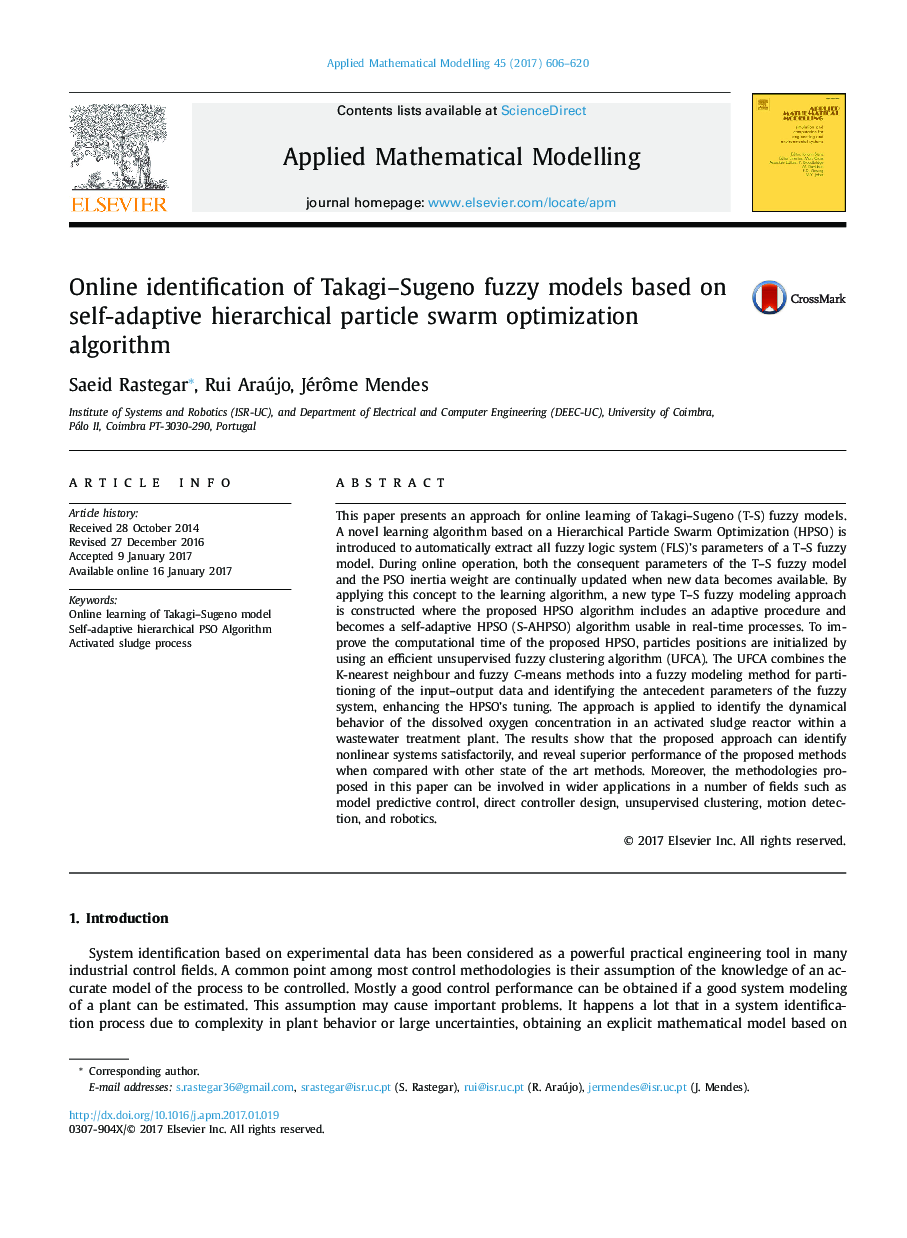
چکیده انگلیسی
This paper presents an approach for online learning of Takagi-Sugeno (T-S) fuzzy models. A novel learning algorithm based on a Hierarchical Particle Swarm Optimization (HPSO) is introduced to automatically extract all fuzzy logic system (FLS)'s parameters of a T-S fuzzy model. During online operation, both the consequent parameters of the T-S fuzzy model and the PSO inertia weight are continually updated when new data becomes available. By applying this concept to the learning algorithm, a new type T-S fuzzy modeling approach is constructed where the proposed HPSO algorithm includes an adaptive procedure and becomes a self-adaptive HPSO (S-AHPSO) algorithm usable in real-time processes. To improve the computational time of the proposed HPSO, particles positions are initialized by using an efficient unsupervised fuzzy clustering algorithm (UFCA). The UFCA combines the K-nearest neighbour and fuzzy C-means methods into a fuzzy modeling method for partitioning of the input-output data and identifying the antecedent parameters of the fuzzy system, enhancing the HPSO's tuning. The approach is applied to identify the dynamical behavior of the dissolved oxygen concentration in an activated sludge reactor within a wastewater treatment plant. The results show that the proposed approach can identify nonlinear systems satisfactorily, and reveal superior performance of the proposed methods when compared with other state of the art methods. Moreover, the methodologies proposed in this paper can be involved in wider applications in a number of fields such as model predictive control, direct controller design, unsupervised clustering, motion detection, and robotics.
ناشر
Database: Elsevier - ScienceDirect (ساینس دایرکت)
Journal: Applied Mathematical Modelling - Volume 45, May 2017, Pages 606-620
Journal: Applied Mathematical Modelling - Volume 45, May 2017, Pages 606-620
نویسندگان
Saeid Rastegar, Rui Araújo, Jérôme Mendes,