کد مقاله | کد نشریه | سال انتشار | مقاله انگلیسی | نسخه تمام متن |
---|---|---|---|---|
5513340 | 1541204 | 2017 | 8 صفحه PDF | دانلود رایگان |
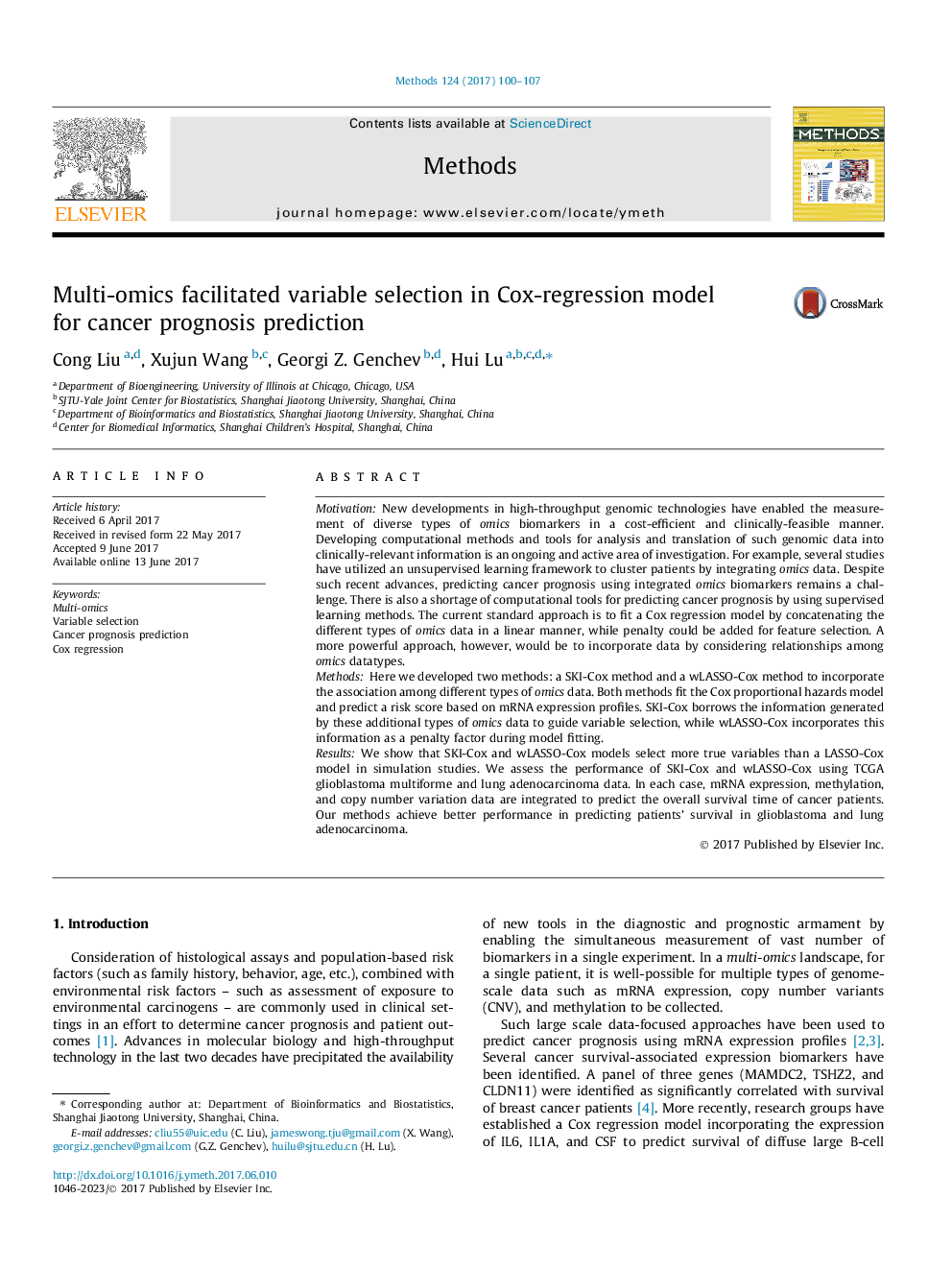
- Conventional LASSO-Cox model could be used to predict cancer patients' survival by concatenating multi-omics data.
- SKI-Cox borrows the information generated by additional types of omics data to guide variable selection.
- wLASSO-Cox puts a penalty factor to take the information derived from other omics data into account.
- Both methods only use mRNA-expression data for prediction.
MotivationNew developments in high-throughput genomic technologies have enabled the measurement of diverse types of omics biomarkers in a cost-efficient and clinically-feasible manner. Developing computational methods and tools for analysis and translation of such genomic data into clinically-relevant information is an ongoing and active area of investigation. For example, several studies have utilized an unsupervised learning framework to cluster patients by integrating omics data. Despite such recent advances, predicting cancer prognosis using integrated omics biomarkers remains a challenge. There is also a shortage of computational tools for predicting cancer prognosis by using supervised learning methods. The current standard approach is to fit a Cox regression model by concatenating the different types of omics data in a linear manner, while penalty could be added for feature selection. A more powerful approach, however, would be to incorporate data by considering relationships among omics datatypes.MethodsHere we developed two methods: a SKI-Cox method and a wLASSO-Cox method to incorporate the association among different types of omics data. Both methods fit the Cox proportional hazards model and predict a risk score based on mRNA expression profiles. SKI-Cox borrows the information generated by these additional types of omics data to guide variable selection, while wLASSO-Cox incorporates this information as a penalty factor during model fitting.ResultsWe show that SKI-Cox and wLASSO-Cox models select more true variables than a LASSO-Cox model in simulation studies. We assess the performance of SKI-Cox and wLASSO-Cox using TCGA glioblastoma multiforme and lung adenocarcinoma data. In each case, mRNA expression, methylation, and copy number variation data are integrated to predict the overall survival time of cancer patients. Our methods achieve better performance in predicting patients' survival in glioblastoma and lung adenocarcinoma.
Journal: Methods - Volume 124, 15 July 2017, Pages 100-107