کد مقاله | کد نشریه | سال انتشار | مقاله انگلیسی | نسخه تمام متن |
---|---|---|---|---|
5513579 | 1541216 | 2016 | 9 صفحه PDF | دانلود رایگان |
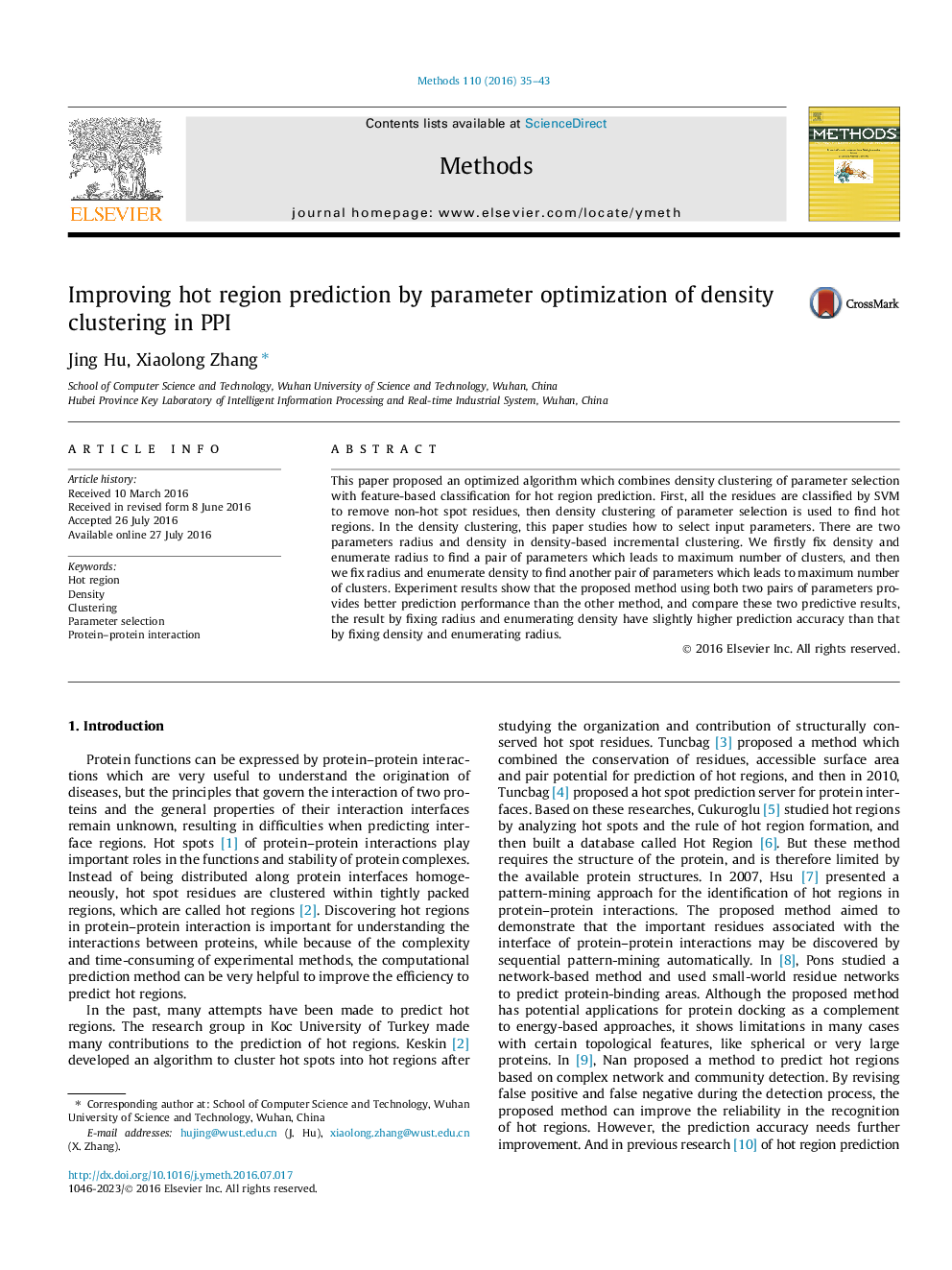
- Density-based and feature-based method for hot region prediction is presented.
- Parameter selection is used in clustering to improve prediction accuracy.
- Visualization and analysis of hybrid method result is provided.
This paper proposed an optimized algorithm which combines density clustering of parameter selection with feature-based classification for hot region prediction. First, all the residues are classified by SVM to remove non-hot spot residues, then density clustering of parameter selection is used to find hot regions. In the density clustering, this paper studies how to select input parameters. There are two parameters radius and density in density-based incremental clustering. We firstly fix density and enumerate radius to find a pair of parameters which leads to maximum number of clusters, and then we fix radius and enumerate density to find another pair of parameters which leads to maximum number of clusters. Experiment results show that the proposed method using both two pairs of parameters provides better prediction performance than the other method, and compare these two predictive results, the result by fixing radius and enumerating density have slightly higher prediction accuracy than that by fixing density and enumerating radius.
Journal: Methods - Volume 110, 1 November 2016, Pages 35-43