کد مقاله | کد نشریه | سال انتشار | مقاله انگلیسی | نسخه تمام متن |
---|---|---|---|---|
5754597 | 1620999 | 2017 | 10 صفحه PDF | دانلود رایگان |
عنوان انگلیسی مقاله ISI
Potential of hyperspectral metrics and classifiers for mapping Brazilian savannas in the rainy and dry seasons
دانلود مقاله + سفارش ترجمه
دانلود مقاله ISI انگلیسی
رایگان برای ایرانیان
کلمات کلیدی
موضوعات مرتبط
مهندسی و علوم پایه
علوم زمین و سیارات
کامپیوتر در علوم زمین
پیش نمایش صفحه اول مقاله
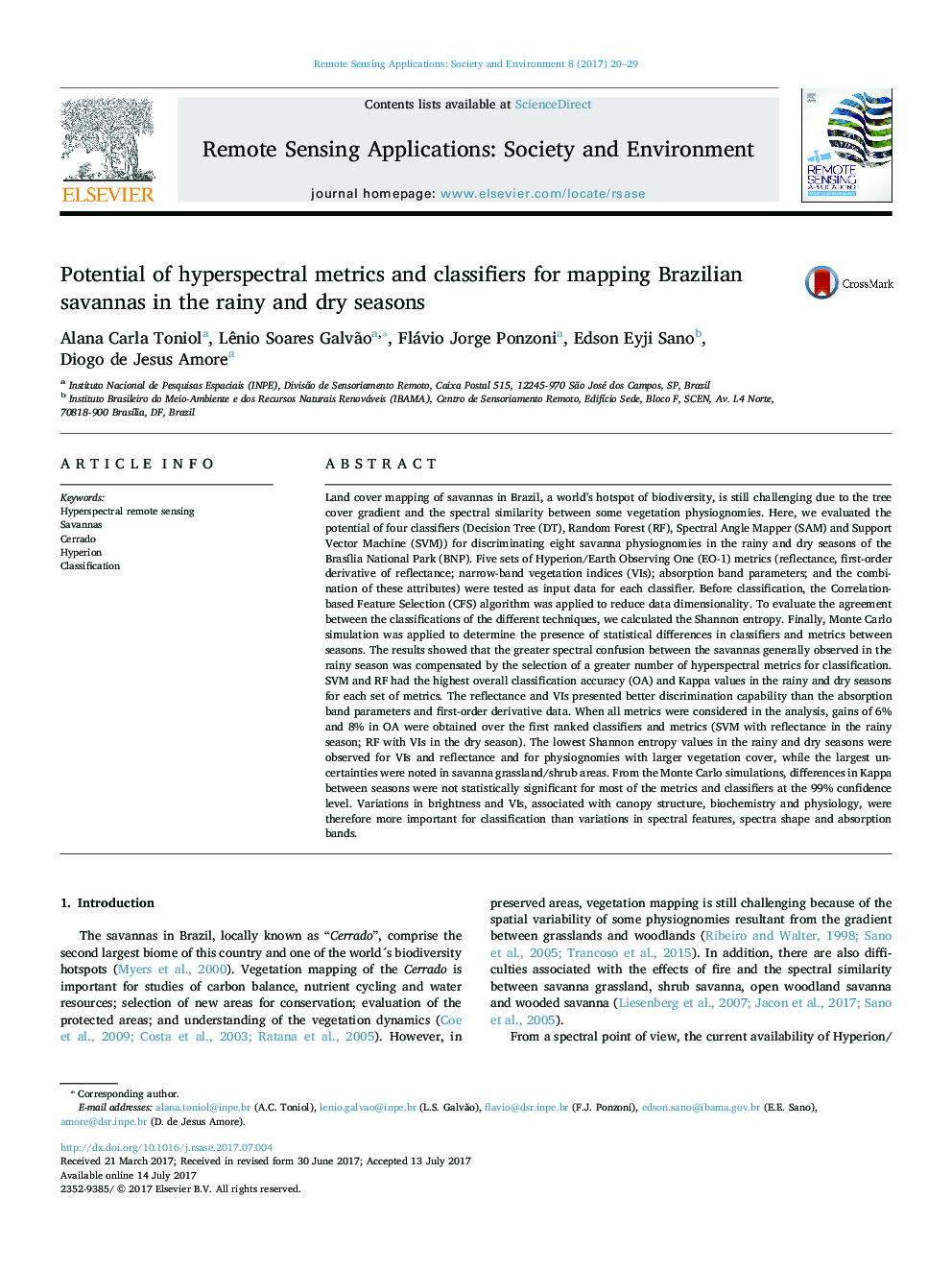
چکیده انگلیسی
Land cover mapping of savannas in Brazil, a world's hotspot of biodiversity, is still challenging due to the tree cover gradient and the spectral similarity between some vegetation physiognomies. Here, we evaluated the potential of four classifiers (Decision Tree (DT), Random Forest (RF), Spectral Angle Mapper (SAM) and Support Vector Machine (SVM)) for discriminating eight savanna physiognomies in the rainy and dry seasons of the BrasÃlia National Park (BNP). Five sets of Hyperion/Earth Observing One (EO-1) metrics (reflectance, first-order derivative of reflectance; narrow-band vegetation indices (VIs); absorption band parameters; and the combination of these attributes) were tested as input data for each classifier. Before classification, the Correlation-based Feature Selection (CFS) algorithm was applied to reduce data dimensionality. To evaluate the agreement between the classifications of the different techniques, we calculated the Shannon entropy. Finally, Monte Carlo simulation was applied to determine the presence of statistical differences in classifiers and metrics between seasons. The results showed that the greater spectral confusion between the savannas generally observed in the rainy season was compensated by the selection of a greater number of hyperspectral metrics for classification. SVM and RF had the highest overall classification accuracy (OA) and Kappa values in the rainy and dry seasons for each set of metrics. The reflectance and VIs presented better discrimination capability than the absorption band parameters and first-order derivative data. When all metrics were considered in the analysis, gains of 6% and 8% in OA were obtained over the first ranked classifiers and metrics (SVM with reflectance in the rainy season; RF with VIs in the dry season). The lowest Shannon entropy values in the rainy and dry seasons were observed for VIs and reflectance and for physiognomies with larger vegetation cover, while the largest uncertainties were noted in savanna grassland/shrub areas. From the Monte Carlo simulations, differences in Kappa between seasons were not statistically significant for most of the metrics and classifiers at the 99% confidence level. Variations in brightness and VIs, associated with canopy structure, biochemistry and physiology, were therefore more important for classification than variations in spectral features, spectra shape and absorption bands.
ناشر
Database: Elsevier - ScienceDirect (ساینس دایرکت)
Journal: Remote Sensing Applications: Society and Environment - Volume 8, November 2017, Pages 20-29
Journal: Remote Sensing Applications: Society and Environment - Volume 8, November 2017, Pages 20-29
نویسندگان
Alana Carla Toniol, Lênio Soares Galvão, Flávio Jorge Ponzoni, Edson Eyji Sano, Diogo de Jesus Amore,