کد مقاله | کد نشریه | سال انتشار | مقاله انگلیسی | نسخه تمام متن |
---|---|---|---|---|
6233772 | 1608172 | 2013 | 4 صفحه PDF | دانلود رایگان |
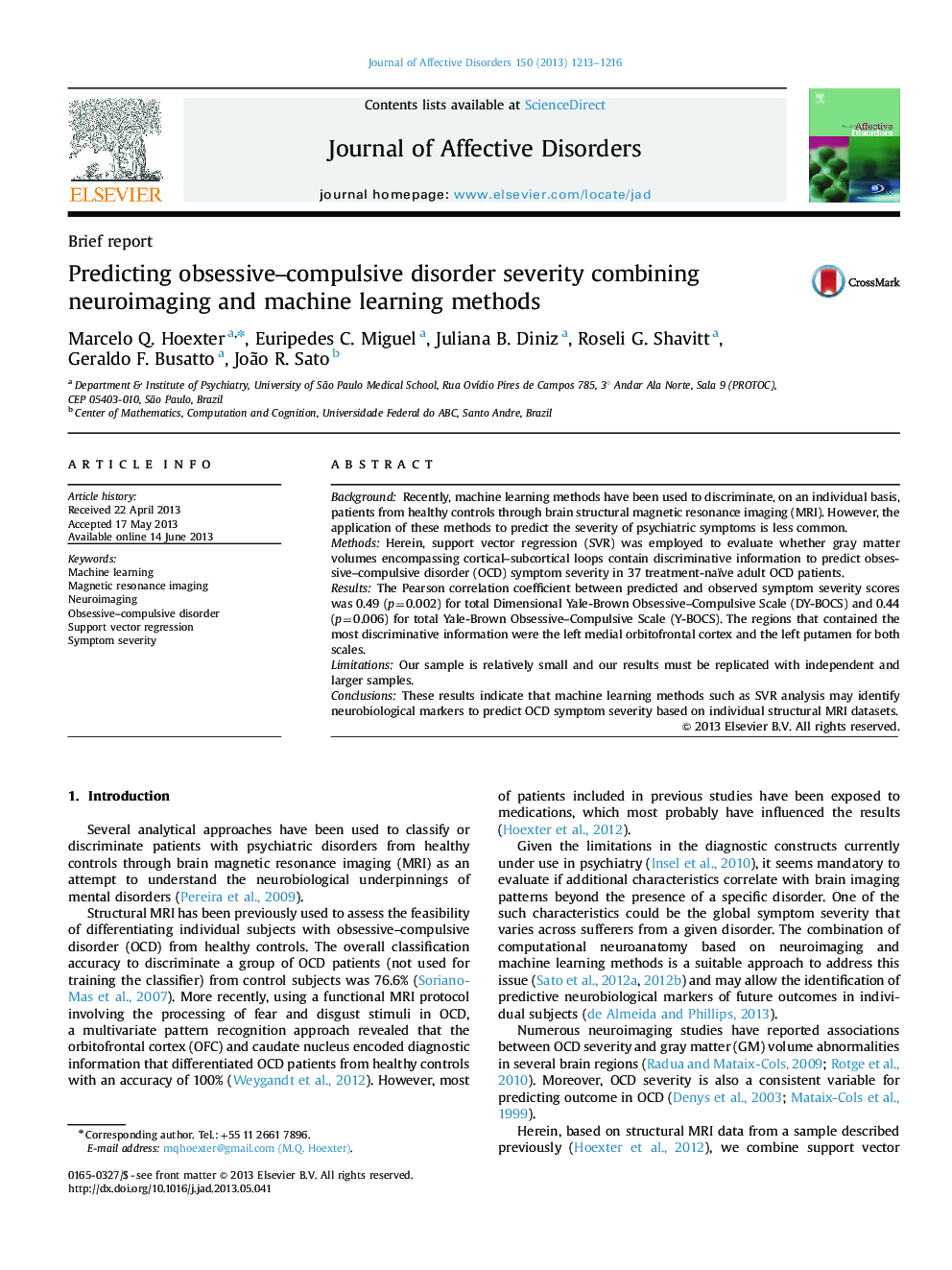
BackgroundRecently, machine learning methods have been used to discriminate, on an individual basis, patients from healthy controls through brain structural magnetic resonance imaging (MRI). However, the application of these methods to predict the severity of psychiatric symptoms is less common.MethodsHerein, support vector regression (SVR) was employed to evaluate whether gray matter volumes encompassing cortical-subcortical loops contain discriminative information to predict obsessive-compulsive disorder (OCD) symptom severity in 37 treatment-naïve adult OCD patients.ResultsThe Pearson correlation coefficient between predicted and observed symptom severity scores was 0.49 (p=0.002) for total Dimensional Yale-Brown Obsessive-Compulsive Scale (DY-BOCS) and 0.44 (p=0.006) for total Yale-Brown Obsessive-Compulsive Scale (Y-BOCS). The regions that contained the most discriminative information were the left medial orbitofrontal cortex and the left putamen for both scales.LimitationsOur sample is relatively small and our results must be replicated with independent and larger samples.ConclusionsThese results indicate that machine learning methods such as SVR analysis may identify neurobiological markers to predict OCD symptom severity based on individual structural MRI datasets.
Journal: Journal of Affective Disorders - Volume 150, Issue 3, 25 September 2013, Pages 1213-1216