کد مقاله | کد نشریه | سال انتشار | مقاله انگلیسی | نسخه تمام متن |
---|---|---|---|---|
6540689 | 158863 | 2015 | 10 صفحه PDF | دانلود رایگان |
عنوان انگلیسی مقاله ISI
An automatic object-based method for optimal thresholding in UAV images: Application for vegetation detection in herbaceous crops
دانلود مقاله + سفارش ترجمه
دانلود مقاله ISI انگلیسی
رایگان برای ایرانیان
کلمات کلیدی
موضوعات مرتبط
مهندسی و علوم پایه
مهندسی کامپیوتر
نرم افزارهای علوم کامپیوتر
پیش نمایش صفحه اول مقاله
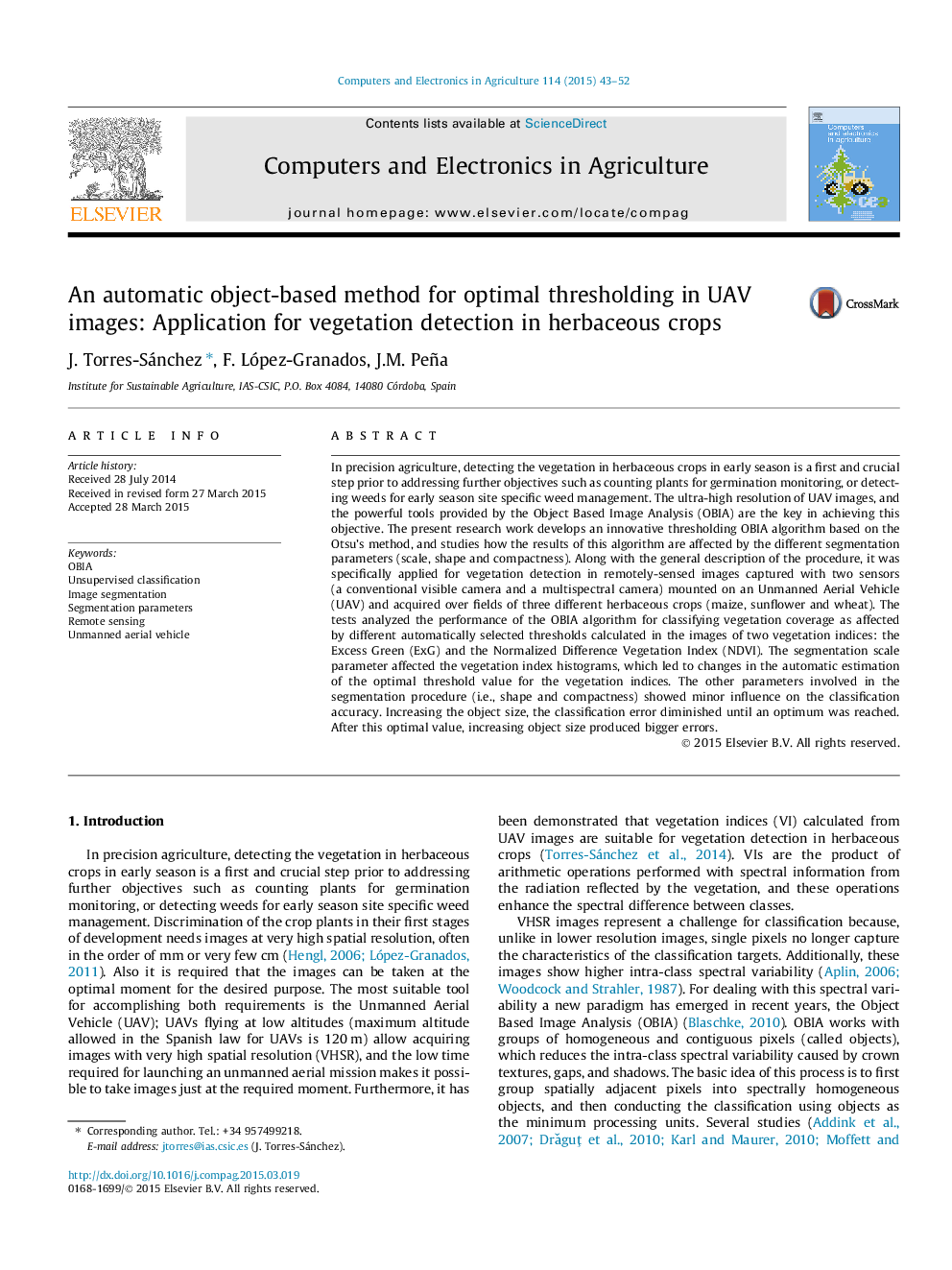
چکیده انگلیسی
In precision agriculture, detecting the vegetation in herbaceous crops in early season is a first and crucial step prior to addressing further objectives such as counting plants for germination monitoring, or detecting weeds for early season site specific weed management. The ultra-high resolution of UAV images, and the powerful tools provided by the Object Based Image Analysis (OBIA) are the key in achieving this objective. The present research work develops an innovative thresholding OBIA algorithm based on the Otsu's method, and studies how the results of this algorithm are affected by the different segmentation parameters (scale, shape and compactness). Along with the general description of the procedure, it was specifically applied for vegetation detection in remotely-sensed images captured with two sensors (a conventional visible camera and a multispectral camera) mounted on an Unmanned Aerial Vehicle (UAV) and acquired over fields of three different herbaceous crops (maize, sunflower and wheat). The tests analyzed the performance of the OBIA algorithm for classifying vegetation coverage as affected by different automatically selected thresholds calculated in the images of two vegetation indices: the Excess Green (ExG) and the Normalized Difference Vegetation Index (NDVI). The segmentation scale parameter affected the vegetation index histograms, which led to changes in the automatic estimation of the optimal threshold value for the vegetation indices. The other parameters involved in the segmentation procedure (i.e., shape and compactness) showed minor influence on the classification accuracy. Increasing the object size, the classification error diminished until an optimum was reached. After this optimal value, increasing object size produced bigger errors.
ناشر
Database: Elsevier - ScienceDirect (ساینس دایرکت)
Journal: Computers and Electronics in Agriculture - Volume 114, June 2015, Pages 43-52
Journal: Computers and Electronics in Agriculture - Volume 114, June 2015, Pages 43-52
نویسندگان
J. Torres-Sánchez, F. López-Granados, J.M. Peña,