کد مقاله | کد نشریه | سال انتشار | مقاله انگلیسی | نسخه تمام متن |
---|---|---|---|---|
6874893 | 1441463 | 2018 | 25 صفحه PDF | دانلود رایگان |
عنوان انگلیسی مقاله ISI
A malicious threat detection model for cloud assisted internet of things (CoT) based industrial control system (ICS) networks using deep belief network
دانلود مقاله + سفارش ترجمه
دانلود مقاله ISI انگلیسی
رایگان برای ایرانیان
کلمات کلیدی
موضوعات مرتبط
مهندسی و علوم پایه
مهندسی کامپیوتر
نظریه محاسباتی و ریاضیات
پیش نمایش صفحه اول مقاله
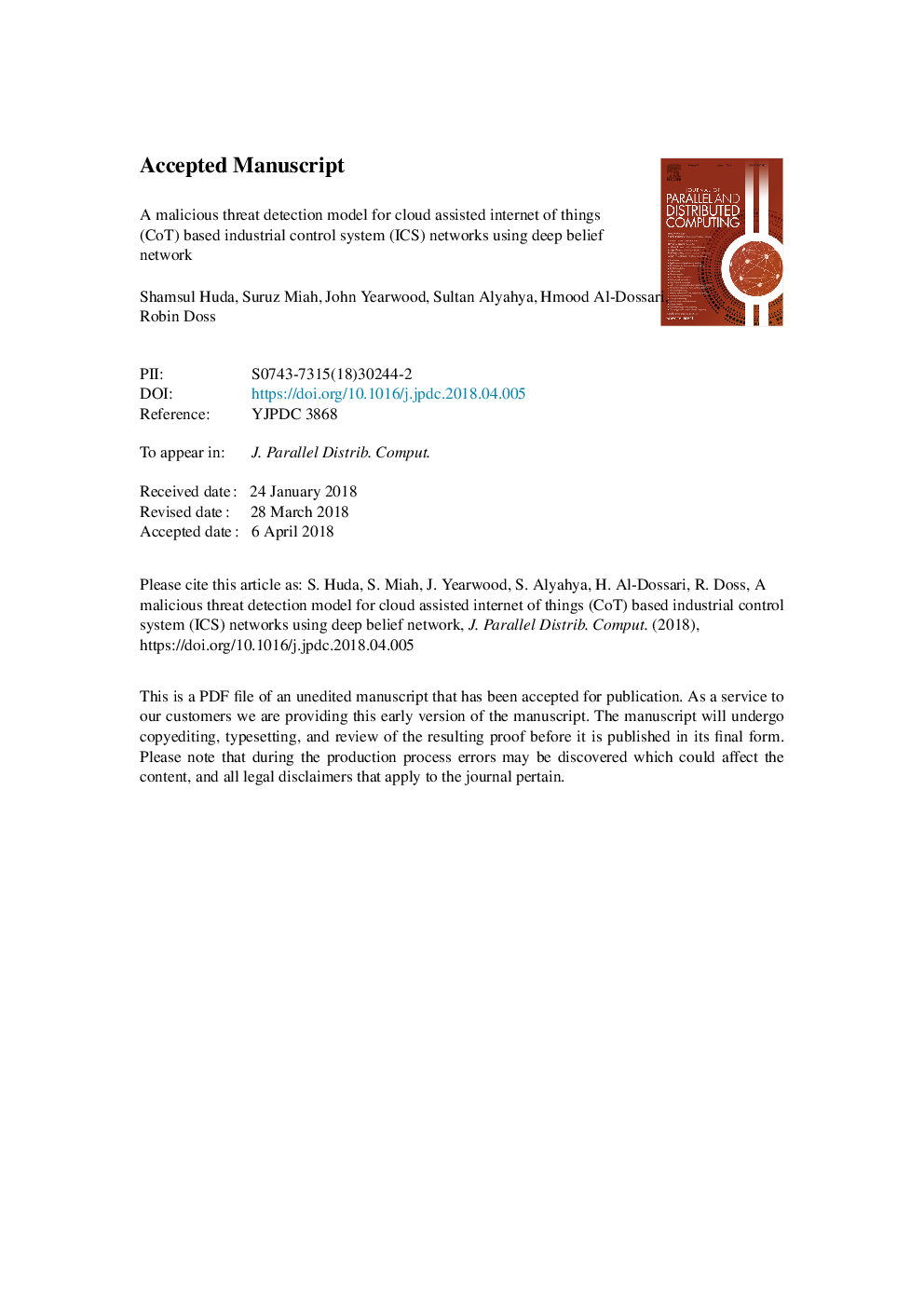
چکیده انگلیسی
Two different types of deep learning based detection models are proposed in this work. The first model uses a disjoint training and testing data for a deep belief network (DBN) and corresponding artificial neural network (ANN). In the second proposed detection model, DBN is trained using new unlabeled data to provide DBN with additional knowledge about the changes in the malicious attack patterns. Novelty of the proposed detection models is that the models are adaptive where training procedures is simpler than earlier work (Huda et al, 2017) and can adapt new malware behaviors from already available and cheap unlabeled data at the same time. This will avoid expensive manual labeling of new attacks and corresponding time complexity making it feasible for ICS networks. Performances of standard DBNs are sensitive to its configurations and values for the hyper-parameters including number of hidden nodes, learning rate and number epochs. Therefore proposed detection models find an optimal configuration by varying the structure of DBNs and other parameters. The proposed detection models are extensively tested on a real malware test bed. Experimental results show that the proposed approaches achieve higher accuracies than standard detection algorithms and obtain similar performances with earlier semi-supervised work (Huda et al., 2017) but provide a comparatively simplified training model.
ناشر
Database: Elsevier - ScienceDirect (ساینس دایرکت)
Journal: Journal of Parallel and Distributed Computing - Volume 120, October 2018, Pages 23-31
Journal: Journal of Parallel and Distributed Computing - Volume 120, October 2018, Pages 23-31
نویسندگان
Shamsul Huda, Suruz Miah, John Yearwood, Sultan Alyahya, Hmood Al-Dossari, Robin Doss,