کد مقاله | کد نشریه | سال انتشار | مقاله انگلیسی | نسخه تمام متن |
---|---|---|---|---|
7104212 | 1460336 | 2018 | 11 صفحه PDF | دانلود رایگان |
عنوان انگلیسی مقاله ISI
An improved variable selection method for support vector regression in NIR spectral modeling
دانلود مقاله + سفارش ترجمه
دانلود مقاله ISI انگلیسی
رایگان برای ایرانیان
کلمات کلیدی
موضوعات مرتبط
مهندسی و علوم پایه
مهندسی شیمی
تکنولوژی و شیمی فرآیندی
پیش نمایش صفحه اول مقاله
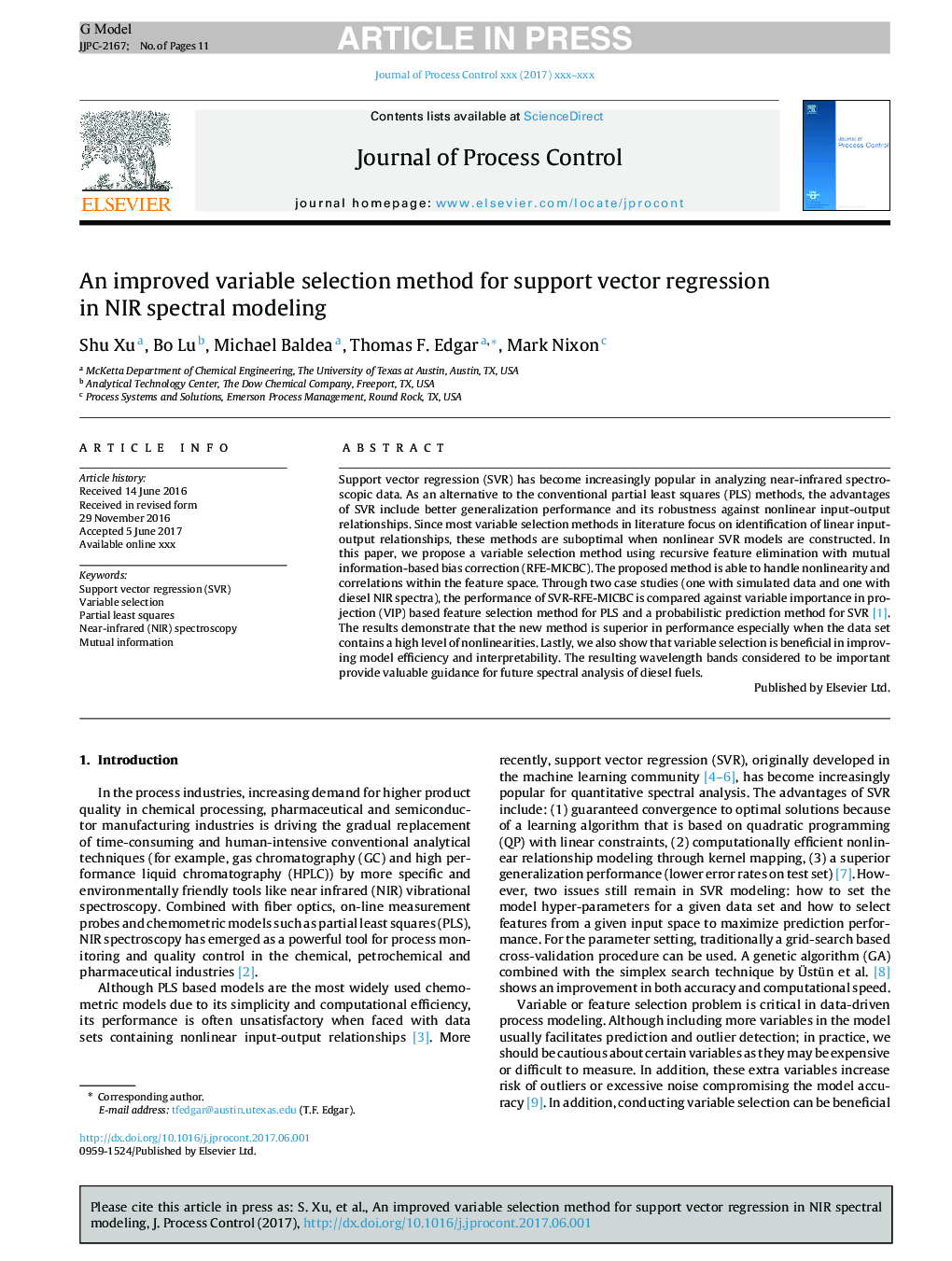
چکیده انگلیسی
Support vector regression (SVR) has become increasingly popular in analyzing near-infrared spectroscopic data. As an alternative to the conventional partial least squares (PLS) methods, the advantages of SVR include better generalization performance and its robustness against nonlinear input-output relationships. Since most variable selection methods in literature focus on identification of linear input-output relationships, these methods are suboptimal when nonlinear SVR models are constructed. In this paper, we propose a variable selection method using recursive feature elimination with mutual information-based bias correction (RFE-MICBC). The proposed method is able to handle nonlinearity and correlations within the feature space. Through two case studies (one with simulated data and one with diesel NIR spectra), the performance of SVR-RFE-MICBC is compared against variable importance in projection (VIP) based feature selection method for PLS and a probabilistic prediction method for SVR [1]. The results demonstrate that the new method is superior in performance especially when the data set contains a high level of nonlinearities. Lastly, we also show that variable selection is beneficial in improving model efficiency and interpretability. The resulting wavelength bands considered to be important provide valuable guidance for future spectral analysis of diesel fuels.
ناشر
Database: Elsevier - ScienceDirect (ساینس دایرکت)
Journal: Journal of Process Control - Volume 67, July 2018, Pages 83-93
Journal: Journal of Process Control - Volume 67, July 2018, Pages 83-93
نویسندگان
Shu Xu, Bo Lu, Michael Baldea, Thomas F. Edgar, Mark Nixon,