کد مقاله | کد نشریه | سال انتشار | مقاله انگلیسی | نسخه تمام متن |
---|---|---|---|---|
7562689 | 1491526 | 2016 | 11 صفحه PDF | دانلود رایگان |
عنوان انگلیسی مقاله ISI
Including noise characteristics in MCR to improve mapping and component extraction from spectral images
دانلود مقاله + سفارش ترجمه
دانلود مقاله ISI انگلیسی
رایگان برای ایرانیان
کلمات کلیدی
موضوعات مرتبط
مهندسی و علوم پایه
شیمی
شیمی آنالیزی یا شیمی تجزیه
پیش نمایش صفحه اول مقاله
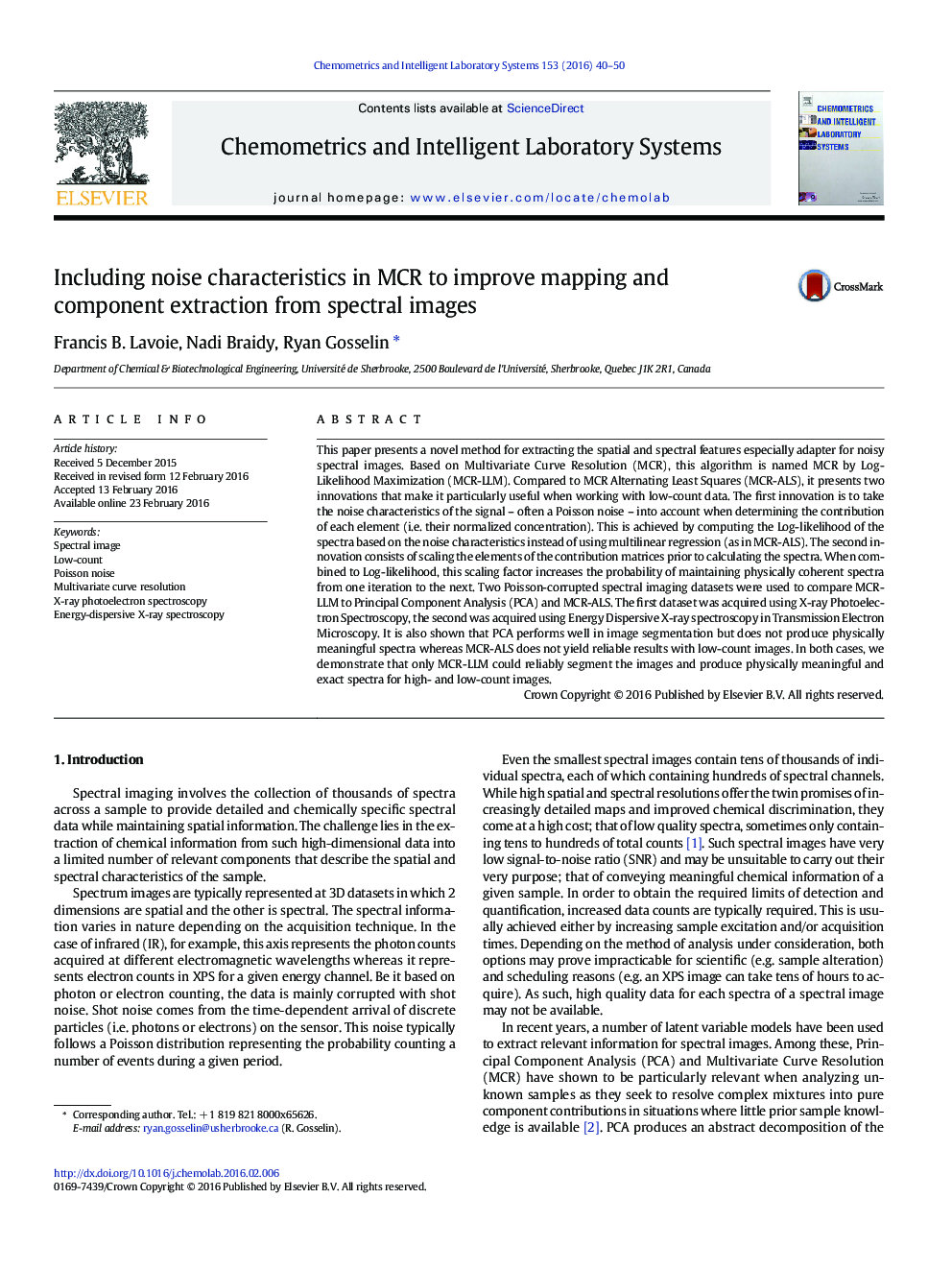
چکیده انگلیسی
This paper presents a novel method for extracting the spatial and spectral features especially adapter for noisy spectral images. Based on Multivariate Curve Resolution (MCR), this algorithm is named MCR by Log-Likelihood Maximization (MCR-LLM). Compared to MCR Alternating Least Squares (MCR-ALS), it presents two innovations that make it particularly useful when working with low-count data. The first innovation is to take the noise characteristics of the signal - often a Poisson noise - into account when determining the contribution of each element (i.e. their normalized concentration). This is achieved by computing the Log-likelihood of the spectra based on the noise characteristics instead of using multilinear regression (as in MCR-ALS). The second innovation consists of scaling the elements of the contribution matrices prior to calculating the spectra. When combined to Log-likelihood, this scaling factor increases the probability of maintaining physically coherent spectra from one iteration to the next. Two Poisson-corrupted spectral imaging datasets were used to compare MCR-LLM to Principal Component Analysis (PCA) and MCR-ALS. The first dataset was acquired using X-ray Photoelectron Spectroscopy, the second was acquired using Energy Dispersive X-ray spectroscopy in Transmission Electron Microscopy. It is also shown that PCA performs well in image segmentation but does not produce physically meaningful spectra whereas MCR-ALS does not yield reliable results with low-count images. In both cases, we demonstrate that only MCR-LLM could reliably segment the images and produce physically meaningful and exact spectra for high- and low-count images.
ناشر
Database: Elsevier - ScienceDirect (ساینس دایرکت)
Journal: Chemometrics and Intelligent Laboratory Systems - Volume 153, 15 April 2016, Pages 40-50
Journal: Chemometrics and Intelligent Laboratory Systems - Volume 153, 15 April 2016, Pages 40-50
نویسندگان
Francis B. Lavoie, Nadi Braidy, Ryan Gosselin,