کد مقاله | کد نشریه | سال انتشار | مقاله انگلیسی | نسخه تمام متن |
---|---|---|---|---|
89450 | 159342 | 2008 | 7 صفحه PDF | دانلود رایگان |
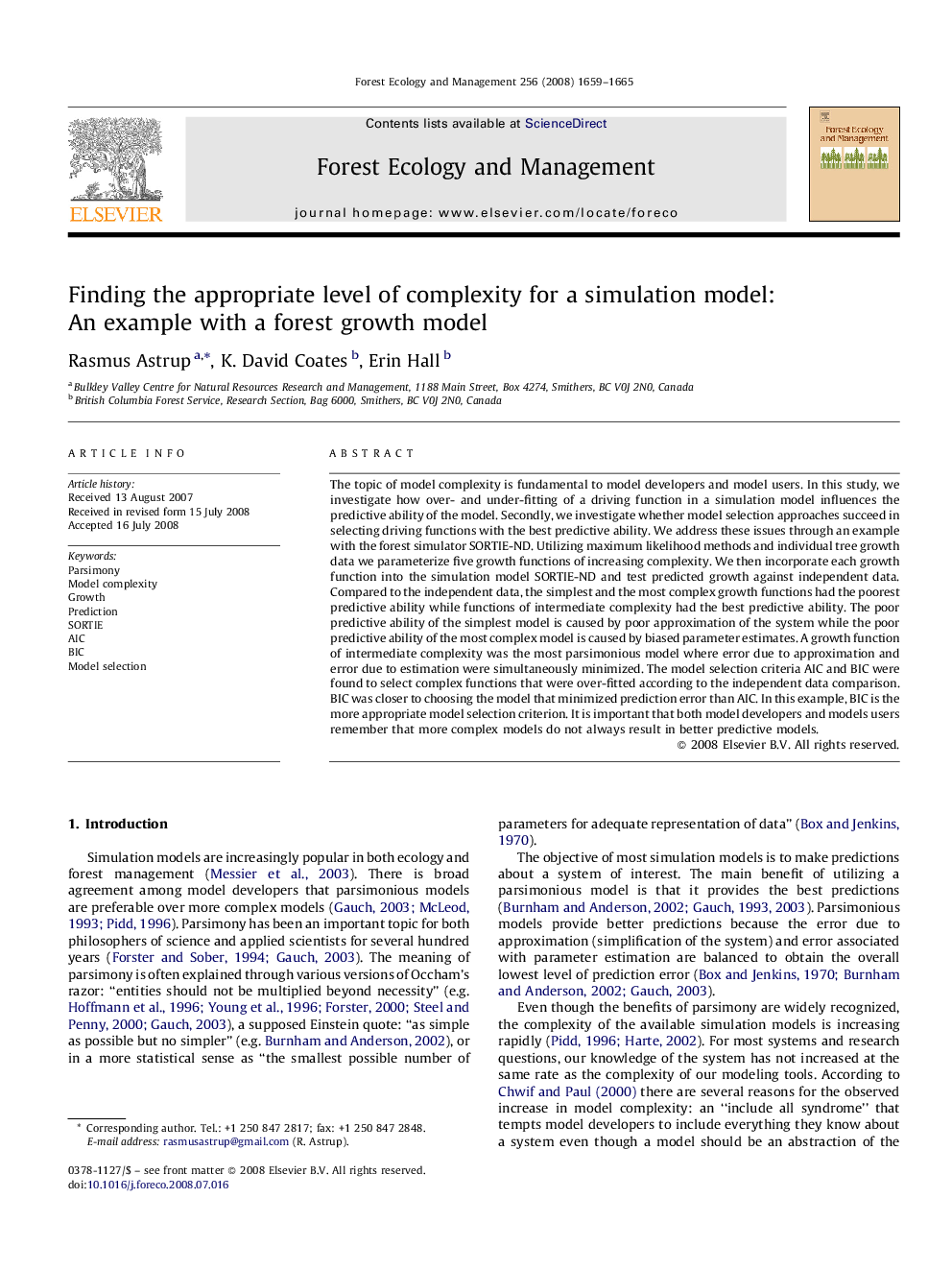
The topic of model complexity is fundamental to model developers and model users. In this study, we investigate how over- and under-fitting of a driving function in a simulation model influences the predictive ability of the model. Secondly, we investigate whether model selection approaches succeed in selecting driving functions with the best predictive ability. We address these issues through an example with the forest simulator SORTIE-ND. Utilizing maximum likelihood methods and individual tree growth data we parameterize five growth functions of increasing complexity. We then incorporate each growth function into the simulation model SORTIE-ND and test predicted growth against independent data. Compared to the independent data, the simplest and the most complex growth functions had the poorest predictive ability while functions of intermediate complexity had the best predictive ability. The poor predictive ability of the simplest model is caused by poor approximation of the system while the poor predictive ability of the most complex model is caused by biased parameter estimates. A growth function of intermediate complexity was the most parsimonious model where error due to approximation and error due to estimation were simultaneously minimized. The model selection criteria AIC and BIC were found to select complex functions that were over-fitted according to the independent data comparison. BIC was closer to choosing the model that minimized prediction error than AIC. In this example, BIC is the more appropriate model selection criterion. It is important that both model developers and models users remember that more complex models do not always result in better predictive models.
Journal: Forest Ecology and Management - Volume 256, Issue 10, 10 November 2008, Pages 1659–1665