کد مقاله | کد نشریه | سال انتشار | مقاله انگلیسی | نسخه تمام متن |
---|---|---|---|---|
8994365 | 1114394 | 2005 | 16 صفحه PDF | دانلود رایگان |
عنوان انگلیسی مقاله ISI
Quantitative Structure-Pharmacokinetic Relationships for Drug Distribution Properties by Using General Regression Neural Network
دانلود مقاله + سفارش ترجمه
دانلود مقاله ISI انگلیسی
رایگان برای ایرانیان
کلمات کلیدی
موضوعات مرتبط
علوم پزشکی و سلامت
داروسازی، سم شناسی و علوم دارویی
اکتشاف دارویی
پیش نمایش صفحه اول مقاله
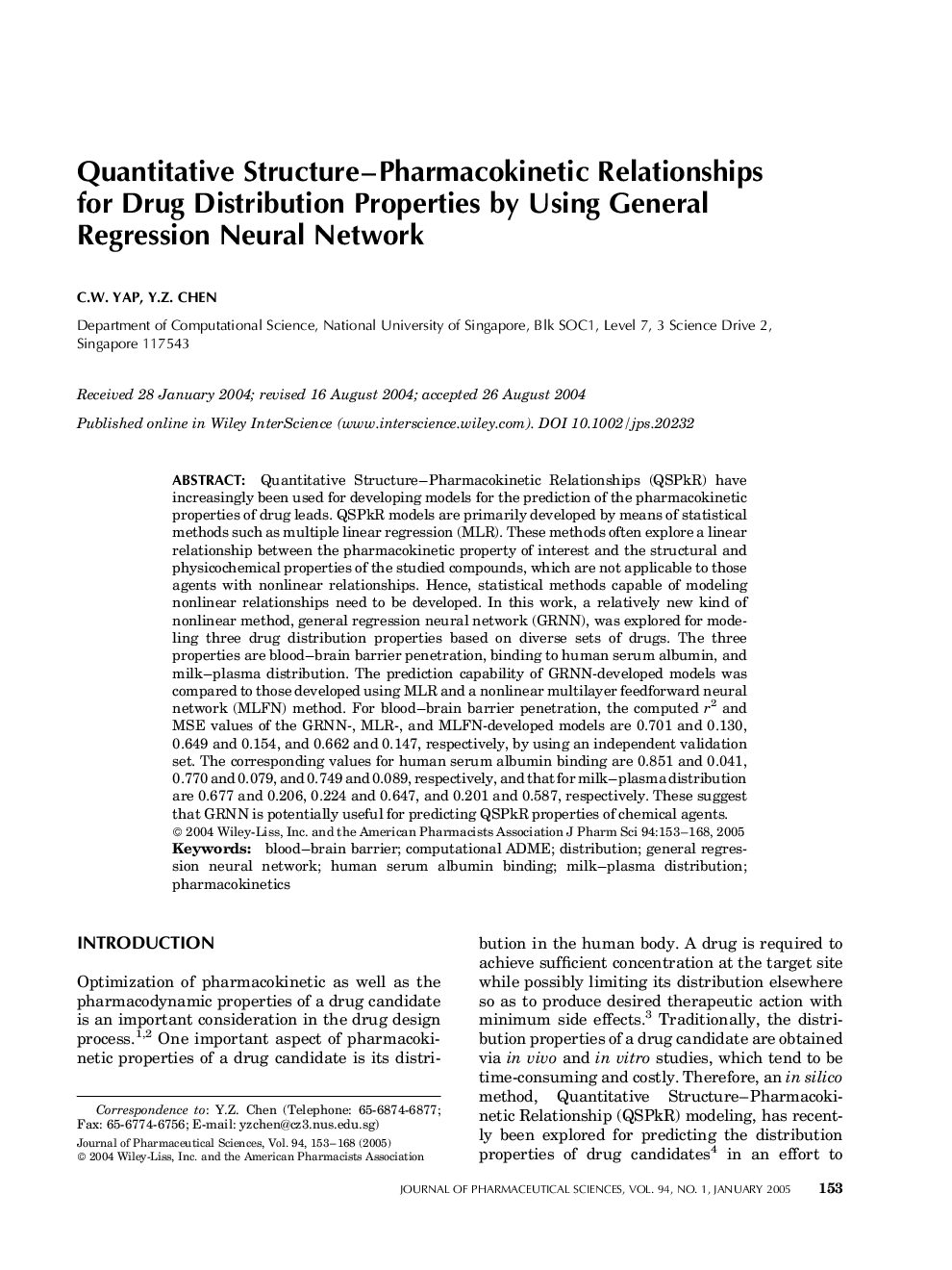
چکیده انگلیسی
Quantitative Structure-Pharmacokinetic Relationships (QSPkR) have increasingly been used for developing models for the prediction of the pharmacokinetic properties of drug leads. QSPkR models are primarily developed by means of statistical methods such as multiple linear regression (MLR). These methods often explore a linear relationship between the pharmacokinetic property of interest and the structural and physicochemical properties of the studied compounds, which are not applicable to those agents with nonlinear relationships. Hence, statistical methods capable of modeling nonlinear relationships need to be developed. In this work, a relatively new kind of nonlinear method, general regression neural network (GRNN), was explored for modeling three drug distribution properties based on diverse sets of drugs. The three properties are blood-brain barrier penetration, binding to human serum albumin, and milk-plasma distribution. The prediction capability of GRNNâdeveloped models was compared to those developed using MLR and a nonlinear multilayer feedforward neural network (MLFN) method. For blood-brain barrier penetration, the computed r2 and MSE values of the GRNNâ, MLRâ, and MLFNâdeveloped models are 0.701 and 0.130, 0.649 and 0.154, and 0.662 and 0.147, respectively, by using an independent validation set. The corresponding values for human serum albumin binding are 0.851 and 0.041, 0.770 and 0.079, and 0.749 and 0.089, respectively, and that for milk-plasma distribution are 0.677 and 0.206, 0.224 and 0.647, and 0.201 and 0.587, respectively. These suggest that GRNN is potentially useful for predicting QSPkR properties of chemical agents.
ناشر
Database: Elsevier - ScienceDirect (ساینس دایرکت)
Journal: Journal of Pharmaceutical Sciences - Volume 94, Issue 1, January 2005, Pages 153-168
Journal: Journal of Pharmaceutical Sciences - Volume 94, Issue 1, January 2005, Pages 153-168
نویسندگان
C.W. Yap, Y.Z. Chen,