کد مقاله | کد نشریه | سال انتشار | مقاله انگلیسی | نسخه تمام متن |
---|---|---|---|---|
10140719 | 1646045 | 2018 | 36 صفحه PDF | دانلود رایگان |
عنوان انگلیسی مقاله ISI
DBPPred-PDSD: Machine learning approach for prediction of DNA-binding proteins using Discrete Wavelet Transform and optimized integrated features space
دانلود مقاله + سفارش ترجمه
دانلود مقاله ISI انگلیسی
رایگان برای ایرانیان
کلمات کلیدی
موضوعات مرتبط
مهندسی و علوم پایه
شیمی
شیمی آنالیزی یا شیمی تجزیه
پیش نمایش صفحه اول مقاله
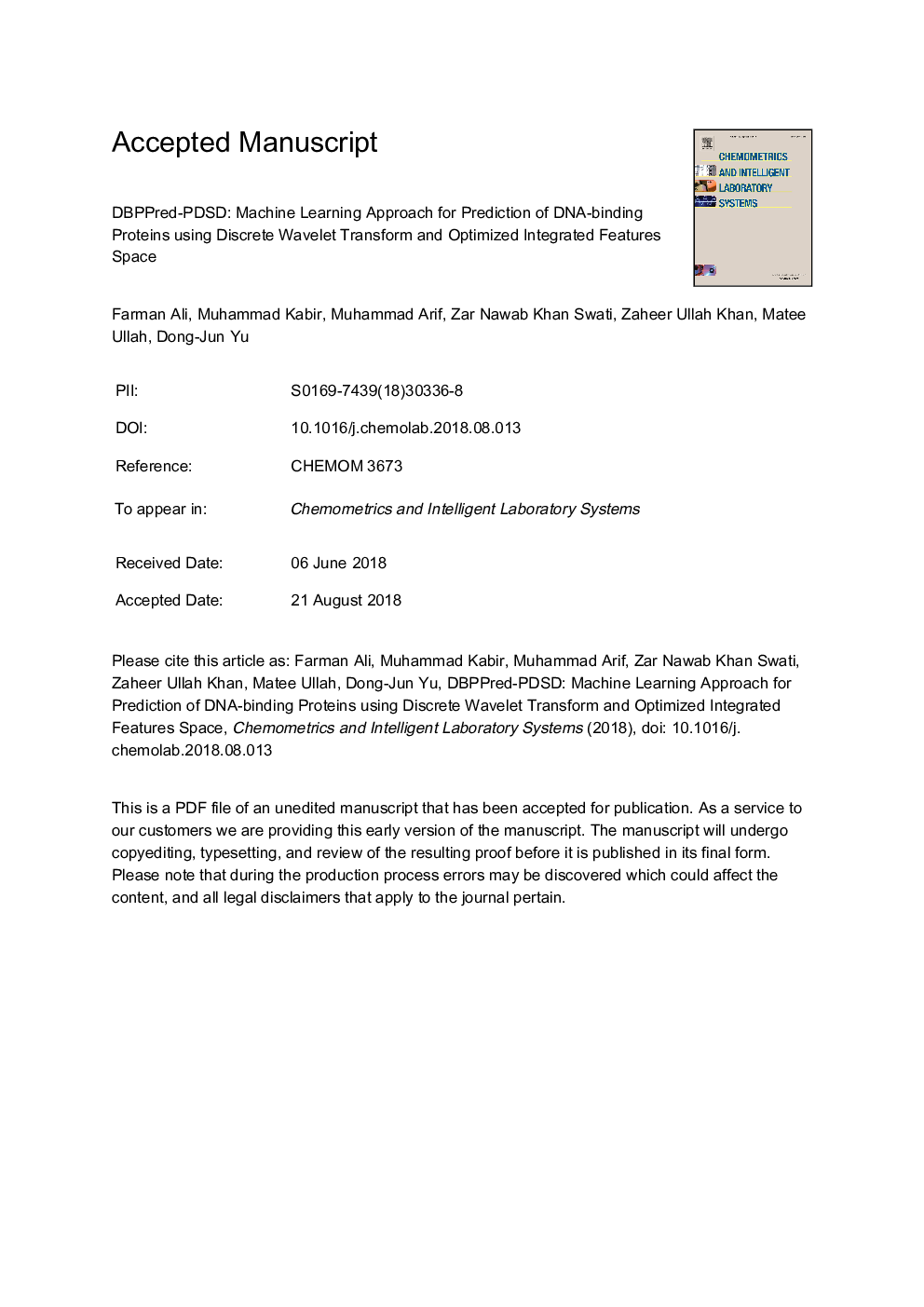
چکیده انگلیسی
DNA-binding proteins play a crucial role in various biological processes such as regulation of DNA modification, repair, replication, and transcription. These proteins widely participate in the production of drugs, antibiotics, and steroids. Many computational approaches have been developed to identify DNA-binding proteins, but some methods are time-consuming and expensive while some are laborious. Still, it is a challenging task for the researchers to develop highly promising computational methods to identify DNA-binding proteins with high precision. In our work, we developed a new computational approach named as DBPPred-PDSD which has more promising prediction power for DNA-binding proteins. We employed two datasets, extracted features via Split Amino Acid Composition (SAAC) and Position Specific Scoring Matrix (PSSM). Further, we applied the Discrete Wavelet Transform (DWT) on PSSM to extract dominant features. From these features space, optimal features are generated by Maximum Relevance and Minimum Redundancy (mRMR) and fused. To obtain highly informative features, we used Support Vector Machine-Recursive Feature Elimination (SVM-RFE) and provided to well-known classifiers namely Support Vector Machine (SVM) and Random Forest (RF). Our model with the SVM classifier on three tests i.e. Jackknife cross-validation, 10-fold cross-validation and Independent tests achieved the highest success rate than other existing methods in the literature.
ناشر
Database: Elsevier - ScienceDirect (ساینس دایرکت)
Journal: Chemometrics and Intelligent Laboratory Systems - Volume 182, 15 November 2018, Pages 21-30
Journal: Chemometrics and Intelligent Laboratory Systems - Volume 182, 15 November 2018, Pages 21-30
نویسندگان
Farman Ali, Muhammad Kabir, Muhammad Arif, Zar Nawab Khan Swati, Zaheer Ullah Khan, Matee Ullah, Dong-Jun Yu,