کد مقاله | کد نشریه | سال انتشار | مقاله انگلیسی | نسخه تمام متن |
---|---|---|---|---|
10560924 | 969755 | 2005 | 6 صفحه PDF | دانلود رایگان |
عنوان انگلیسی مقاله ISI
Principal component analysis versus fuzzy principal component analysis
دانلود مقاله + سفارش ترجمه
دانلود مقاله ISI انگلیسی
رایگان برای ایرانیان
موضوعات مرتبط
مهندسی و علوم پایه
شیمی
شیمی آنالیزی یا شیمی تجزیه
پیش نمایش صفحه اول مقاله
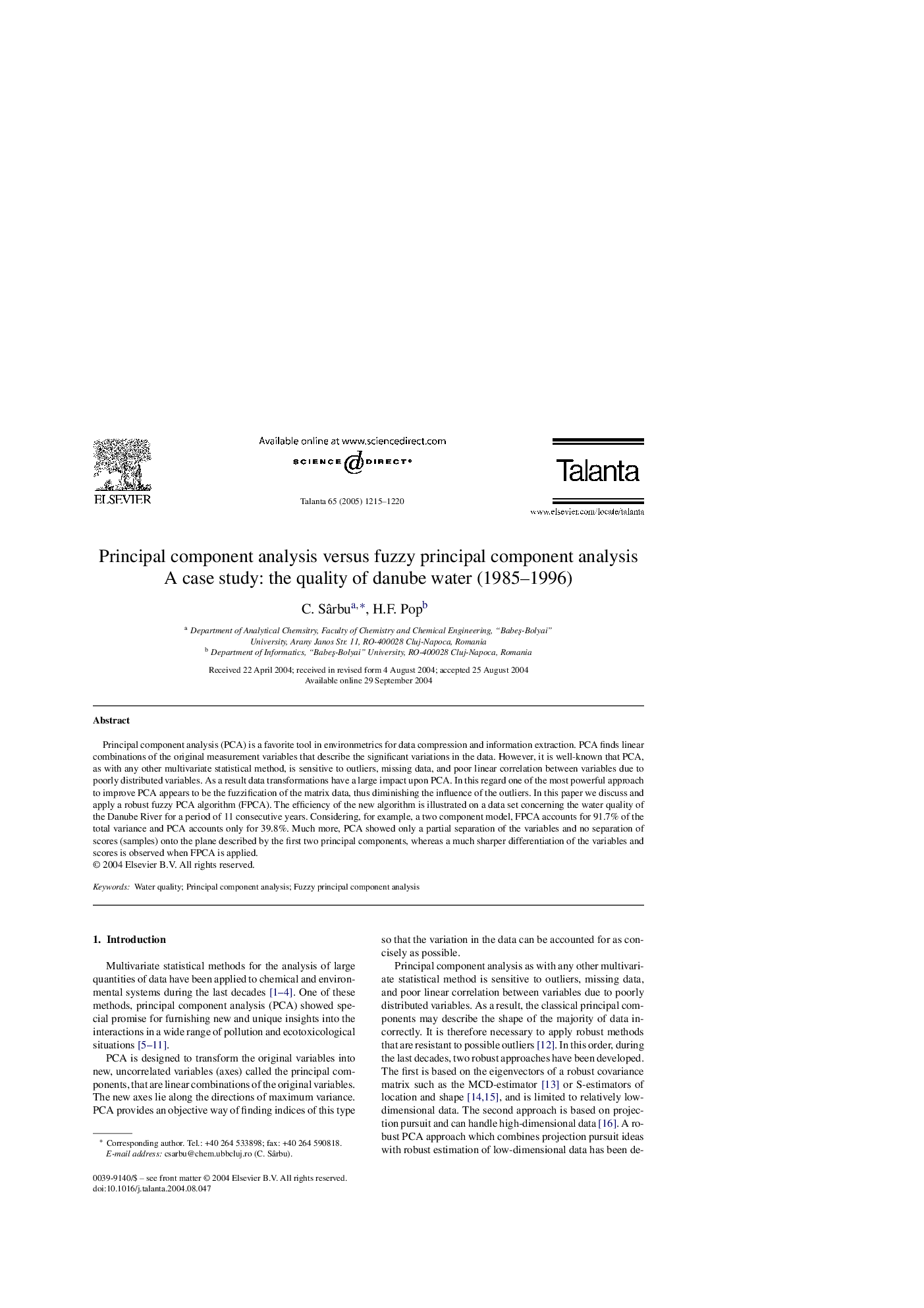
چکیده انگلیسی
Principal component analysis (PCA) is a favorite tool in environmetrics for data compression and information extraction. PCA finds linear combinations of the original measurement variables that describe the significant variations in the data. However, it is well-known that PCA, as with any other multivariate statistical method, is sensitive to outliers, missing data, and poor linear correlation between variables due to poorly distributed variables. As a result data transformations have a large impact upon PCA. In this regard one of the most powerful approach to improve PCA appears to be the fuzzification of the matrix data, thus diminishing the influence of the outliers. In this paper we discuss and apply a robust fuzzy PCA algorithm (FPCA). The efficiency of the new algorithm is illustrated on a data set concerning the water quality of the Danube River for a period of 11 consecutive years. Considering, for example, a two component model, FPCA accounts for 91.7% of the total variance and PCA accounts only for 39.8%. Much more, PCA showed only a partial separation of the variables and no separation of scores (samples) onto the plane described by the first two principal components, whereas a much sharper differentiation of the variables and scores is observed when FPCA is applied.
ناشر
Database: Elsevier - ScienceDirect (ساینس دایرکت)
Journal: Talanta - Volume 65, Issue 5, 15 March 2005, Pages 1215-1220
Journal: Talanta - Volume 65, Issue 5, 15 March 2005, Pages 1215-1220
نویسندگان
C. Sârbu, H.F. Pop,